Towards Equity Projecting and Converging the Human Development Index in Indonesia
on
pISSN : 2301 – 8968
JEKT ♦ 17 [1] :90-107
eISSN : 2303 – 0186
Towards Equity: Projecting and Converging the Human Development Index in Indonesia
ABSTRACT
Human development has become an imperative for Indonesia amid the ongoing demographic bonus. However, various challenges persist in the realm of human development in the country, as evidenced by disparities in Human Development Index (HDI) achievements. This research aims to project Indonesia's HDI for the years 2023, 2024, 2035, and the "Golden Indonesia" period in 2045 to discern the developmental trajectory of HDI and its disparities. Additionally, it seeks to measure the convergence of Indonesia's HDI to attain equitable development. The results of the trend projection estimations indicate that Indonesia's HDI is projected to be 73.74 in 2023 and 74.28 in 2024, which is anticipated to fall below the target set by the government, aiming for an HDI of 75.54 in 2024. Indonesia's HDI is projected to reach 80.22 in 2035 and 85.62 in 2045, categorizing it as 'Very High,' aligning with the vision for the Golden Indonesia in 2045. Furthermore, conditional convergence analysis results indicate that it would take 75 years for provinces with lower HDI to catch up with those with higher HDI, achieving zero inequality. Based on the estimation results, it is found that Per Capita Spending on Educational Functions is a significant variable influencing HDI convergence.
Keywords: Human Development Index, Disparity, Convergence, Projection JEL Classification: C01, O11, O15
INTRODUCTION
The concept of human development emphasizes humans as the central and ultimate focus of development. Human development can be defined as efforts to enhance the quality of human life across various dimensions, such as education, health, access to resources, and security. The primary goal of human development is to improve human well-being equitably, comprehensively, and sustainably. This concept has been internationally recognized and employed by various organizations to assess development progress across different countries (UNDP, 2016). The urgency of human development is highly relevant to
Indonesia's current demographic bonus. Data from the Badan Pusat Statistik (2022) indicate that the majority of Indonesia's population falls within the productive age group of 15-64 years, comprising 69.16% of productive-age males and 69.08% of females. Furthermore, it is projected that by 2045, there will be 207.992 million individuals in the productive age group, constituting 65.21% of Indonesia's total population of 318.956 million. The dominance of this productive-age population represents significant potential for a nation, as it can contribute positively to economic growth and national development (Wisnumurti,
Darma, & Suasih, 2018). This aligns with the goals of human development, which not only focus on economic growth but also on individual empowerment, improvement of quality of life, and social justice. Effective human development policies must encompass efforts to reduce unemployment and poverty (Wulandari et al., 2022). The Human Development Index (HDI) is a widely used indicator to measure the level of human development in a country (see Kovacevic, 2010; Ghislandi, Sanderson, & Scherbov, 2019; Dasic et al., 2020; Mangaraj & Aparajita, 2020; Resce, 2021).
In general, Indonesia's Human Development Index (HDI) has exhibited relatively good performance, experiencing an upward trend over the years. The average HDI score for Indonesia from 2010 to 2022 is 69.96 (medium category), with 11 provinces having HDI scores above the national average. Some of them fall into the high category, such as DKI Jakarta (score 79.28), DI Yogyakarta (score 78.15), East Kalimantan (score 74.60), Riau Islands (score 73.99), Bali (score 73.55), Riau (score 71.18), North Sulawesi (score 71.00), Banten (score 70.74), and West Sumatra (score 70.47), while two other provinces, North Kalimantan (score 69.98) and Aceh (score 69.97), fall into the medium HDI category. However, there are still provinces with low HDI, such as Papua, with an average score of 58.13 from 2010 to 2022.
Additionally, Indonesia continues to face various challenges in human development. In terms of education, indicators such as the average years of schooling (AYS) and expected years of schooling (EYS) within the HDI have shown improvement. In 2022, the average years of schooling for the population aged 25 and above in Indonesia is 8.69 years. This implies that, on average, individuals aged 25 and above have completed their education up to the eighth grade of Junior High School (SMP). Compared to 2021, the average years of schooling in 2022 increased by 1.76 percent. Regarding the EYS indicator, Indonesia's score in 2022 is 13.10 years, meaning that individuals aged 7 and above are expected to complete education up to the first year of university. Provinces like DI Yogyakarta have an EYS score reaching 15.65 years (third year of university), but there are still provinces like Papua with an EYS of 11.14 years (not yet completed high school).
Furthermore, there are concerns about the low quality of life for newborns, despite the early phase of human life being crucial as it influences one's quality of life into later stages (Coatsworth, 1996). This is reflected in the prevalence of stunting among toddlers in Indonesia, which remains relatively high. The prevalence of stunting in Indonesian toddlers reached 21.6% in 2022. While this figure decreased by 2.8 points from the previous year, there are still 18
provinces with stunting prevalence higher than the national average. For instance, the prevalence of stunting in NTT decreased from 37.8% in 2021. Subsequently, West Sulawesi ranked second with a prevalence of stunting in toddlers at 35%. Moreover, West Papua and West Nusa Tenggara have stunting prevalence rates of 34.6% and 32.7%, respectively. This implies that in these regions, 1 out of 3 toddlers still faces the risk of stunting. On the other hand, Bali has the lowest national prevalence of stunting, standing at only 8%.
The disparities in the Human Development Index (HDI) across various provinces in Indonesia provide insights into the inequalities in human development achievements. While some provinces record high HDI scores, such as DKI Jakarta and DI Yogyakarta, there are also provinces with low HDI, such as Papua. This phenomenon reflects the uneven distribution of resources and access to education, health, and infrastructure nationwide. Essentially, this inequality is not unique to Indonesia; it is observed in other countries as well, as seen in studies by Banerjee & Kuri (2015) in India, Aguenane (2020) in Morocco, and Shi & Tang (2020) in China. Research in developing countries often identifies the primary sources of inequality as non-uniform standards of living distribution and imbalanced access to basic social facilities. However, trends of
decreasing HDI disparities towards convergence have also been observed.
This research aims to project the HDI for the years 2023, 2024, 2035, and 2045 to observe the development trends and disparities. Additionally, a convergence analysis will be conducted to understand how HDI disparities in Indonesia can be leveled. Overall, the study of disparities in human development in Indonesia and how to address them plays a crucial and relevant role in advancing human resources towards improvement. Through a deep understanding of the challenges faced by Indonesia in achieving quality human development, a comprehensive picture of the quality of human resource development in Indonesia will be obtained. This includes assessing the effectiveness of government interventions and projecting the future conditions of human development. Therefore, the goal of this research is to identify regional disparities in human development in Indonesia and project the conditions of human development in the country. The research findings will be used as policy inputs for the government's efforts in accelerating human resource development reforms until 2024, and for projections in 2035 and 2045. These inputs cover the relevance of human development policies in Indonesia across various timelines.
DATA Indonesia. The table below elucidates the
This research relies on secondary data variables employed in this study along with obtained from scholarly publications, their respective data sources. research findings, and other relevant documents published by authoritative institutions such as the Central Statistics
Agency (Badan Pusat Statistik) and the Directorate General of Fiscal Balance, Ministry of Finance of the Republic of
Table 1. Data, Symbol, and Source
No |
Data |
Symbol |
Source |
1 |
Human Development Index |
HDI |
BPS |
2 |
Government Spending in Health per Capita |
GS_Health_Cap |
DJPK |
3 |
Government Spending in Education per Capita |
GS_Education_Cap |
DJPK |
4 |
Government Spending in Economy per Capita |
GS_Economy_Cap |
DJPK |
5 |
Investment |
Investment |
BPS |
6 |
Percentage of Poverty |
Poverty |
BPS |
Source: Researcher (2023) |
The sample utilized in this research observations amounts to 408. The operational comprises 34 provinces in Indonesia, definitions of each variable are outlined as spanning the timeframe from 2010 to 2022. follows: Consequently, the total number of
Table 2. Operational Definition of Variables
No Data |
Operational Definition |
1 Human Development Index |
HDI is a statistical measure that shows the progress and quality of human life in a country. HDI is based on three main dimensions, namely long and healthy life, knowledge, and a decent standard of living. |
|
Represents per capita expenditure of local governments to fund education. Represents per capita expenditure of local governments to fund health. Represents per capita expenditure of local governments to fund the economy, including social spending. |
5 Investment |
Represents expenditure on capital goods that have a useful life of more than one year and are not consumer goods. PMTB includes residential and non-residential buildings, other buildings such as roads and airports, as well as machinery |
No
Data
Operational Definition
6 Percentage of Poverty |
and equipment. Represents the percentage of the population living below the poverty line. |
Source: |
Researcher (2023) |
METHODOLOGIES
To achieve the objectives of this research, the economic growth convergence econometric modeling method was used to identify regional disparities in human development in Indonesia. The method used is panel data regression with the Generalized Least Square (GLS) estimation technique. The convergence method has been used in previous research, especially on the topic of development convergence, such as in Fung (2009), Bahadir & Valev (2015), and Szczepańska-Woszczyna et al (2022). β-Convergence has two hypotheses, namely absolute convergence and conditional convergence. Absolute convergence means that regions with low HDI experience greater growth than regions with high HDI. At an empirical level, this can be done by estimating an econometric model where the HDI at the beginning of the period is the only explanatory variable for HDI growth. Conditional convergence indicates that the model specification includes a number of variables other than the HDI at the beginning of the period which are expected to influence the HDI growth rate. Conditional convergence is considered more adequate to use if you want to know the impact of a particular policy. The absolute
convergence and conditional convergence models in this research are as follows.
Absolute Convergence Model:
∆HDIit = βo + βrLogHDIit~r + μit
Where:
ΔHDI : Growth of Human
Development Index (%)
β0 : Constant
β1 : Convergence Coefficient
Log_HDIt-1 : Logarithm of the Human
Development Index at the Beginning of the Period
µ : Error Term
i : Province
t : Year
Conditional Convergence Model:
n
∆HDIit = θo + S1LogHDIi,, + ^ θjFCCit
7=2 + εit
Where:
ΔHDI : Growth of Human
Development Index (%)
θ0 : Constant
θ1 : Convergence Coefficient
Log_HDIt-1 : Logarithm of the Human
Development Index at the Beginning of the Period
FCC : Several Factors that Can
Influence Convergence
θj : Variable Coefficients that Can
Influence Convergence
ε : Error Term
i : Province
t : Year
Several variables that are thought to influence HDI convergence are Government Spending in Health per Capita, Government Spending in Education per Capita, Government Spending in Economic per Capita, Investment, and Percentage of Poverty. The convergence coefficient β1 or θ1 is a quantity that can be used to calculate the speed of convergence. If the value of β1 or θ1 < 0, then convergence occurs, and conversely if β1 or θ1 > 0, then divergence occurs. Perfect convergence if the value of β1 or θ1 = -1 and perfect divergence if β1 or θ1 = 1. The convergence speed (λ) of an area can be calculated using the formula:
_ [ln(Λ1 + 1)]
^abs — τ
_ [ln(θι + 1)]
^cond — ,p
T is the number of years of observation. By using λ, you can calculate the half life of convergence (h) or the time needed to close half of the initial gap with the formula:
h ln(2)
h——
The half life of convergence has ‘year’ units.
Furthermore, to obtain projection results for the condition of human development in Indonesia until 2045, the Time Series Forecasting Model (Trend Projection) method is used. This technique matches a trend line to a series of historical data points and then projects the line into the future for mid- to long-term forecasting. There are several mathematical trend equations that can be developed in addition to the general model of linear equations (for example, exponential or quadratic). A trend line is simply a linear regression equation where the independent variable (X) is a time period. The form is:
Y = β0+βιX
dimana:
Y: Predicted Value
β0: Intercept β1: Slope
X:Time Period (X — 1,2,3, ...,ri) RESULTS AND DISCUSSION Overview of Government Intervention in Human Development in Indonesia Government intervention is one of the determinants that will determine development achievements. Government interventions in human development can be at different levels of scope and aggregation— from small-scale interventions to interventions that impact entire countries or regions. An example of this effort is the
macroeconomic policy intervention carried out by the central and regional governments (Conradie & Robeyns, 2013). Intervention
through APBN and APBD instruments in expenditure allocation will help increase HDI in terms of its constituent dimensions.
Figure 1. Government Spending in Education, Health, and Economic Per Capita by Province 2010-2022 (Million Rupiah)
a) Education
b) Health
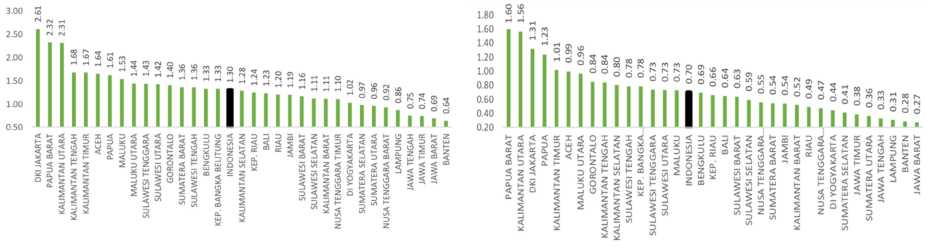
c) Economic
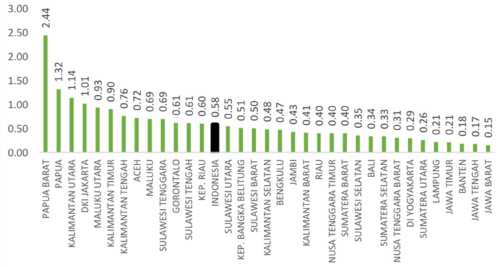
Source: Central Statistics Agency, 2023 (processed)
Education expenditure is a type of regional spending utilized to fund the implementation of governance affairs under the jurisdiction of provinces or regencies/cities in the field of education. Law Number 20 of 2003 regarding the allocation of education funds stipulates that
education funds, apart from teacher salaries and civil servant education costs, should be allocated a minimum of 20% of the State Budget (APBN) in the education sector and a minimum of 20% of the Regional Revenue and Expenditure Budget (Muliza et al., 2017). Government spending in the field of
education encompasses expenditures made by the government in the education sector, including but not limited to the construction of schools, educational facilities, education-related scholarships, and others. According to Figure 1, it can be observed that Indonesia's Per Capita Education Expenditure is IDR 1,300,000. The province with the highest Per Capita Education Expenditure is DKI Jakarta, while the province with the lowest is Banten.
Furthermore, health expenditure is a type of regional spending used to finance the implementation of governance affairs under the jurisdiction of provinces or regencies/cities in the field of health. Based on Law Number 36 of 2009 Article 171 paragraph (2), it is stated that the government's health budget should be allocated a minimum of 10% of the Regional Budget (APBD) excluding salaries. Expenditure in the health budget sector constitutes government spending aimed at fulfilling one of the fundamental rights to obtain health services, including health services and facilities, which are prerequisites for improving community productivity. Government spending in the health sector includes expenditures for the construction of health facilities, public health insurance, and others (Royda & Melvani, 2018). It can be seen from Figure 1 that Indonesia's Per Capita Health Expenditure is IDR 700,000. The province with the highest
Per Capita Health Expenditure is West Papua, while the province with the lowest is West Java. Similarly, Per Capita Economic Expenditure in Indonesia is IDR 580,000. The province with the highest Per Capita Economic Expenditure is West Papua, while the province with the lowest is West Java. The large population size is a contributing factor to the low per capita expenditure.
The presence of densely populated regions with low per capita government spending can lead to several consequences that may have negative impacts on societal well-being (Wu, Tang, & Lin, 2010; Marjit, Sasmal, & Sasmal, 2020). Firstly, the low per capita government spending can result in limited or inadequate quality of basic services such as education and health. Insufficient investment may hinder the access of densely populated areas to quality education and adequate healthcare, creating inequalities in public services. Secondly, low government spending can affect infrastructure and public services, including transportation, sanitation, and clean water. Insufficient investment may lead to an inability to address environmental issues and community well-being, increasing the risk of diseases and accidents. Thirdly, the low per capita government spending can hinder local economic growth. Minimal facilities and support from the government may impede business development and the creation of new job opportunities, resulting in economic
instability and higher levels of poverty. Moreover, low per capita government spending can also trigger mass migration of the population from those areas, creating additional pressure on other regions and increasing inter-regional inequality.
The Projection of the Human Development Index (HDI) for Indonesia in the Years 2023, 2024, 2035, and 2045
Furthermore, projecting the Human Development Index (HDI) is crucial as it provides a more accurate and comprehensive depiction of societal well-being compared to purely economic measurements. The HDI amalgamates health, education, and income parameters, creating an indicator that offers a holistic insight into human development. The projection results, utilizing trend projection on past data from 2010 to 2022, indicate that Indonesia's HDI will continue to rise until 2045, with an average annual
growth rate of 0.72%. All dimensions of the HDI are also projected to increase until 2045, with average growth rates in the Health Dimension (UHH) at 0.23% per year, Education Dimension (HLS) at 1.17% per year, Education Dimension (RLS) at 1.13% per year, and Expenditure Dimension (PPK) at 1.43% per year.
The projected HDI for Indonesia is estimated to be 73.74 in 2023, while in 2024, it is projected to be 74.28. However, the projection for 2024 is anticipated to fall below the target, where the Government aims for an HDI of 75.54 in 2024. In 2022, Indonesia's HDI also failed to reach the Government's targeted figure of 74.01, achieving only 72.91. This indicates that various factors continue to impede the attainment of the HDI in 2022, such as issues in policy implementation, resource constraints, or unforeseen changes in economic and social conditions.
Figure 2. Indonesian HDI Projection Results and Dimensions 2023, 2024, 2035, 2045
90.00 80.00 70.00 ea.oo ⅞∙∞ ⅛.00 ¾e.oo 2p.00 ^.00 ¾.00 |
•— | |||||
2010 |
2022 |
2023 |
2024 |
2035 |
2045 | |
—•—HDI |
66.53 |
72.91 |
73.74 |
74.28 |
80.22 |
85.62 |
—•—Health Dimension |
69.81 |
71.85 |
72.00 |
72.16 |
73.94 |
75.56 |
—^Education Dimension |
11.29 |
13.10 |
13.55 |
13.71 |
15.41 |
16.96 |
—•—Education Dimension |
7.46 |
8.69 |
8.74 |
8.85 |
10.01 |
11.07 |
—•—Expenditure Dimension |
9437 |
11479 |
11675 |
11849 |
13767 |
15511 |
18000
16000
14000
12000
10000
8000
6000
4000
2000
0
Year

Source: Analysis Results, 2023 (processed)
The Human Development Index (HDI) for Indonesia is projected to reach 80.22 in the year 2035, categorizing it as 'Very High' HDI. During this period, Indonesia is estimated to be at the peak of its demographic bonus. Meanwhile, the projected HDI for Indonesia in the year 2045 is 85.62, aligning with the achievement of the Indonesia Emas 2045 vision. The anticipated increase in HDI in the future is expected to have broad positive impacts on societal well-being. With the
elevation of HDI, improvements are anticipated in health, education, and living standards. The enhancement of HDI can also trigger sustainable economic growth, as a healthier and more educated population tends to contribute to a more productive workforce. Thus, the forthcoming increase in HDI not only reflects social progress but can also serve as a catalyst for inclusive and sustainable economic growth (Satrianto & Juniardi, 2023).
Figure 3. HDI Map of Provinces in Indonesia 2010 and 2022 and Projections for 2023, 2024, 2035, 2045
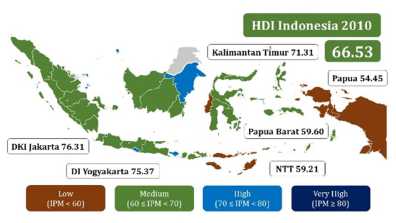
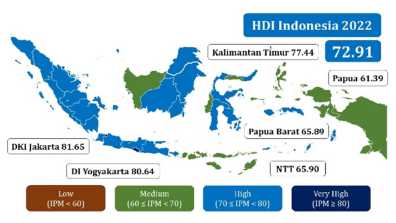
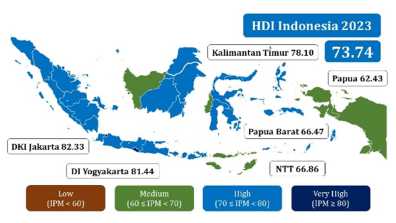
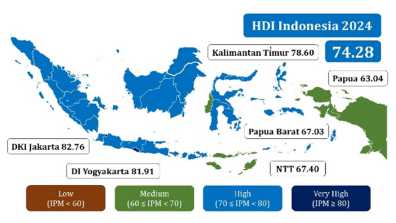
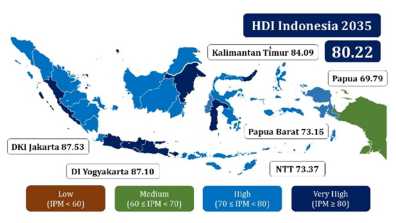
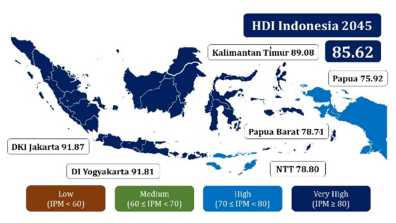
Source: Analysis Results, 2023 (processed)
Examining the HDI condition from a regional perspective, both achieved and projected until 2045, reveals three provinces with the highest HDI: DKI Jakarta, DI Yogyakarta, and East Kalimantan. Meanwhile, the three provinces with the lowest HDI are East Nusa Tenggara, West Papua, and Papua. DKI Jakarta, with the highest HDI, is projected to reach 91.87 in 2045. Subsequently, DI Yogyakarta and East Kalimantan are estimated to touch 91.81 and 89.08, respectively, by 2045. The three lowest-ranking provinces, namely East Nusa Tenggara, West Papua, and Papua, are projected to have HDI scores of 78.80, 78.71, and 75.92, respectively. However, these three lowest-ranking provinces are projected to enter the 'High' HDI category by 2045. The highest and lowest HDI rankings have not changed from 2010 to 2022, according to the projections until 2045.
The attainment of the Indonesia Emas vision is built on four pillars of development: Human Development and Mastery of Science and Technology, Sustainable Economic Development, Equitable Development, and Strengthening National Resilience and Governance (BAPPENAS, 2019). Each pillar encompasses development areas, from education to foreign policy, that must be built and accelerated by 2045 to realize the Indonesia 2045 vision. Overall, the Indonesia 2045 vision aims to achieve better and more equitable welfare for the Indonesian people,
with higher human quality and an elevated Indonesian economy as one of the world's top 5 economic powers, fostering justice in all development areas within the framework of the Unitary State of the Republic of Indonesia that is sovereign and democratic.
The projections for Human Development Index (HDI) growth until 2045 show an increasingly positive trend, reflecting significant progress in human development. Factors such as improved access to education, healthcare system enhancements, and poverty alleviation strategies contribute to the rise in HDI. Innovations in resource management, inclusive policies, and sustainable economic growth are key drivers in achieving this milestone.
Consistent with the findings of this study in the previous section, one of the key factors in improving HDI is investing in the education sector (Escosura, 2010; Citrawan, Widana, & Suarta, 2018). Efforts to enhance access and quality of education, including skills training, will play a crucial role. Inclusive and evenly distributed education programs will help reduce disparities between regions and social groups. Additionally, education relevant to the demands of the global job market should be considered to ensure that individuals have skills aligned with the evolving economy.
Positive projections for HDI growth also necessitate attention to the healthcare
sector (Razmi, Abbasian, & Mohammadi, 2012). Improving accessibility and quality of healthcare services, as well as promoting a healthy lifestyle, can significantly enhance life expectancy and societal well-being. Prevention of diseases and early intervention are also key to achieving sustainable HDI improvement. Sustainable economic growth provides a robust foundation for HDI enhancement. Policies focusing on inclusive economic development, empowerment of local communities, and wise natural resource management will support this improvement process.
Convergence Analysis of the Human Development Index (HDI) in Indonesia Following Barro and Sala-i-Martin (1995), there are two perspectives on how the catchup and convergence processes can occur in a regional context: β-convergence, where poorer regions tend to grow faster than more advanced regions because diminishing marginal returns to capital occur in the latter regions, and σ-convergence, which is related to the decreasing disparities across regions over time. Estimation results indicate that the HDI of provinces in Indonesia experiences both absolute and conditional convergence.
The time needed for provinces with low HDI to catch up with provinces with high HDI until reaching zero disparity is 75 years, in other words, reaching the convergence point. Based on the estimation results, it is found that Per Capita Education Function Expenditure is a significant variable affecting HDI convergence. Investment, as a control variable, does not significantly influence HDI convergence, while Poverty affects HDI convergence.
Table 3. Human Development Index Convergence Estimation Results
Dependent Variables
Growth of HDI |
Growth of HDI | |
Independent Variables |
(Absolute |
(Conditional |
Convergence) |
Convergence) |
Coef |
Prob |
Coef |
Prob | |
Constant |
0.2292 |
0.000*** |
0.4572 |
0.000*** |
Log HDI |
-0.0524 |
0.000*** |
-0.1052 |
0.000*** |
Government Spending in Health per Capita |
- |
- |
0.0012 |
0.406 |
Government Spending in Education per Capita |
- |
- |
0.0019 |
0.043** |
Government Spending in Economic per Capita |
- |
- |
0.0005 |
0.465 |
Investment |
- |
- |
0.00001 |
0.183 |
Percentage of Poverty |
- |
- |
-0.0005 |
0.000*** |
Number of Observation
Time Periods
Prob>chi2
R-Squared
The Half Life of Convergence
404 2011-2022 0.0000 0.6285 155 Years
404 2011-2022 0.0000 0.6558 75 Years
Source: Analysis Results, 2023 (processed)
The disparity in the Human Development Index (HDI) among regions can be caused by various complex and diverse factors. Consequently, disparities in economic growth rates among regions can lead to differences in per capita income and employment opportunities (Lu, 2018; Saudi, 2021). Regions with stronger economies tend to have better access to resources and economic opportunities, while regions with weaker economies struggle to improve living standards and quality of life. Additionally, disparities in infrastructure, such as transportation networks, clean water, sanitation, and electricity, can affect accessibility and quality of life in a region (Calderon & Serven, 2014). Regions with underdeveloped infrastructure may face
difficulties in addressing development challenges and improving the HDI.
The dynamics of growth poles and the inverted U-hypothesis state that regional disparities in developing countries will eventually be reduced through factor mobility. Neoclassical growth theory emphasizes factor mobility on the supply side, particularly the supply of capital, technological change, and labor, as reasons for reducing these disparities. On the other hand, opposing theories, especially dependency theory and structural change, argue that regional inequality is an unavoidable result of capital accumulation and profit maximization (Noorbakhsh, 2003). Specifically in Indonesia, differences in geographical and demographic conditions,
such as remote locations, population size, and regional demographic composition, can affect access to resources and economic opportunities. Sparsely populated and remote areas may face particular challenges in improving the HDI at the regional level. However, the challenge of regional HDI disparities can be overcome through various serious and comprehensive efforts to achieve convergence.
Convergence of the HDI among regions refers to the process in which regions with low HDI tend to experience faster growth than regions with high HDI. This phenomenon indicates that regional HDI disparities can decrease over time, leading to more even human development across all regions. The occurrence of HDI convergence is also found in the research by Permanyer & Smits (2020), who created the Subnational Human Development Index (SHDI) to provide a more detailed picture of human development variations within a country. Covering more than 1,600 regions in 161 countries, the SHDI and its dimensions provide a resolution 10 times higher than what was previously available.
A key finding from the research by Permanyer & Smits (2020) is the significant variation in human development within countries, especially in low- and middleincome developing countries, which aligns with the findings of this study. Educational disparities are a primary factor explaining
inequality in SHDI in low-income developing countries, while differences in living standards are the main factor in more developed countries. HDI convergence can occur due to various factors. First, regions with low HDI usually have more room for significant growth and improvement compared to regions that already have high HDI. Improvements in infrastructure, access to education and health, and economic empowerment programs can have a greater impact on regions with suboptimal development.
Second, when lagging regions experience rapid HDI improvement, positive spillover effects can occur in surrounding areas (Giuntini, 2015). For example, when one region enhances its education or health quality, it will impact nearby regions through the improvement of education or health levels in the neighboring population. Moreover, the role of the government is crucial in promoting HDI convergence. The government can provide support and investment in regions with low HDI through policies focused on development equalization. More equitable resource allocation and strengthened coordination between the central and regional governments can accelerate development in lagging regions.
CONCLUSION AND RECOMMENDATION
From the results of the analysis and discussion, several conclusions can be drawn. First, per capita spending on health, education, and the economy, along with control variables such as investment and poverty, has a significant positive impact on the Human Development Index (HDI). This implies that government interventions through regional spending play a crucial role in improving the HDI. Second, to reduce HDI disparities among provinces, it takes about 75 years for provinces with low HDI to catch up with those with high HDI, provided that it is accompanied by appropriate interventions in spending on education, health, and the economy, as well as increased investment and poverty reduction. Third, the projection results show that Indonesia's HDI will continue to increase until 2045 with an average annual growth of 0.72%, reaching the Very High HDI category in 2035 and aligning with the Vision of Indonesia Emas 2045 in 2045. Fourth, all dimensions of HDI are also projected to increase with varying growth rates, highlighting the importance of attention to health, education, and per capita expenditure. Finally, there are challenges in facing the demographic bonus, including high poverty rates, unequal quality of education, limited access to health facilities, vulnerable food security, and the aging population. Therefore, strategic and planned steps need to be taken to address these
challenges to achieve inclusive and sustainable human development.
Based on the findings of the research and discussion, several policy recommendations are formulated, which can serve as a reference for the central and regional governments in supporting human development in Indonesia. First, given the positive influence of per capita spending on health, education, and the economy on HDI, the government is expected to optimize sectoral budget allocations. The implementation of this policy model involves increasing resource allocation to these sectors, considering their direct impact on HDI indicators, and diversifying the investment portfolio at the central and regional government levels to support HDI growth. Additionally, given the negative impact of poverty on HDI, poverty alleviation policies need to be strengthened with a more focused and outcome-oriented approach. Innovations in the form of economic empowerment programs, targeted social transfers, and skill training are expected to be integral parts of the poverty alleviation strategy.
Next, structured and measurable long-term development planning is needed to achieve zero disparities within 75 years. Increased budget allocations for the education, health, and economic sectors at the central and regional government levels are crucial steps. Adequate funding
allocation will support the implementation of intervention policies to accelerate HDI improvement, reduce disparities, and achieve zero disparities more quickly. Lastly, the integration of technology into key sectors such as education, health, and the economy is considered crucial for achieving efficiency and innovation. Policies supporting digitization and technology adoption need to be implemented to make significant progress in human development, following the projected trend, to achieve the Very High HDI category in all provinces in Indonesia by 2035. The implementation of these recommendations is expected to help realize more equitable and sustainable human development across all regions of Indonesia.
REFERENCE
Aguenane, N. E., 2020. Regional Disparities In Human Development: The Case Of Moroccan Regions. International Journal of Social Sciences and Economic Review, 2(2), 28-34.
Bahadir, B., & Valev, N., 2015. Financial development convergence. Journal of Banking & Finance, 56, 61-71.
Banerjee, A., Kuri, P. K., 2015. Regional disparity and convergence in human development in India. Development Disparities in India: An Enquiry into Convergence, 69-99.
Barro, R. and Sala-i-Martin, X., 1995. Economic Growth. McGraw Hill. New York.
Badan Pusat Statistik. 2022. Kependudukan. https://www.archive.bps.go.id/subj ect/12/kependudukan.html#subjekV iewTab3
BAPPENAS. 2019. Ringkasan Eksekutif Visi Indonesia Emas.
https://perpustakaan.bappenas.go.id /e-
library/file_upload/koleksi/migrasi-data-
publikasi/file/Policy_Paper/Ringkas an%20Eksekutif%20Visi%20Indonesia %202045_Final.pdf
Calderón, C., & Servén, L., 2014.
Infrastructure, growth, and inequality: An overview. World Bank Policy Research Working Paper, (7034).
Citrawan, I. W., Widana, I. W., & Suarta, I., 2018. Education sector optimization for improving human development index. International Journal of Social Sciences and Humanities, 2(1), 117-133.
Coatsworth, J. H., 1996. Welfare. The American historical review, 101(1), 1-12.
Conradie, I., & Robeyns, I., 2013. Aspirations and human development interventions. Journal of Human Development and Capabilities, 14(4), 559-580.
Dasic, B., Devic, Z., Denic, N., Zlatkovic, D., Ilic, I. D., Cao, Y., ... & Le, H. V., 2020. Human development index in a context of human development: Review on the western Balkans countries. Brain and Behavior, 10(9), e01755.
Escosura, L. P. D. L., 2010. Improving human development: a long‐run view. Journal of Economic Surveys, 24(5), 841-894.
Fung, M. K., 2009. Financial development and economic growth: convergence or divergence?. Journal of international money and finance, 28(1), 56-67.
Ghislandi, S., Sanderson, W. C., & Scherbov, S., 2019. A simple measure of human development: The human life indicator. Population and development review, 45(1), 219.
Giuntini, G., 2015. Spillover Effects: Border Effect on Values of Human Development Index (HDI). Available at SSRN 2692266.
Kovacevic, M., 2010. Measurement of inequality in Human Development–A review. Measurement, 35, 1-65.
Lu, D., 2018. Rural-urban income disparity: impact of growth, allocative efficiency and local growth welfare. In Urbanization and Social Welfare in China (pp. 255-268). Routledge.
Mangaraj, B. K., & Aparajita, U., 2020. Constructing a generalized model of the human development index. SocioEconomic Planning Sciences, 70, 100778.
Marjit, S., Sasmal, R., & Sasmal, J., 2020. Composition of public expenditure and growth of per capita income in Indian states: a political
perspective. Journal of Social and Economic Development, 22, 1-17.
Muliza, M., Zulham, T., & Seftarita, C., 2017. Analisis pengaruh belanja
pendidikan, belanja kesehatan,
tingkat kemiskinan dan PDRB terhadap IPM di provinsi Aceh. Jurnal Perspektif Ekonomi Darussalam (Darussalam Journal of Economic Perspec, 3(1), 51-69.
Noorbaksh, Farhad., 2023. Human Development and Regional Disparities in India. Presented in the United Nations World Institute for Development Economics Research Conference on Inequality, Poverty and Human Well-being, Helsinki., Finland 30-31 May 2003
Permanyer, I., & Smits, J., 2020. Inequality in human development across the globe. Population and Development Review, 46(3), 583-601.
Razmi, M. J., Abbasian, E., & Mohammadi, S., 2012. Investigating the effect of government health expenditure on HDI in Iran. Journal of Knowledge Management, Economics and Information Technology, 2(5).
Resce, G., 2021. Wealth-adjusted human development index. Journal of Cleaner Production, 318, 128587.
Royda, R., & Melvani, F. N., 2018. Pengaruh Belanja Pemerintah Untuk Pendidikan, Kesehatan, Infrastruktur Serta Pertanian Terhadap Pertumbuhan Ekonomi Di Kabupaten/Kota Provinsi Sumatera Selatan. Adminika, 4(1), 73–84.
Satrianto, A., & Juniardi, E., 2023. Inclusive Human Development and Inclusive Green Growth: A Simultaneous Approach. International Journal of Sustainable Development & Planning, 18(2).
Saudi, N. D. S., 2021. Analysis of Economic Growth and Income Disparity on Inter-Regional Welfare. Point of View Research Economic Development, 2(4), 68-87.
Shi, Z., & Tang, X., 2020. Exploring the new era: An empirical analysis of China’s regional HDI development. Emerging Markets Finance and Trade, 56(9), 19571970.
Szczepańska-Woszczyna, K., Gedvilaitė, D., Nazarko, J., Stasiukynas, A., & Rubina, A., 2022. Assessment of economic convergence among countries in the European Union. Technological and Economic Development of Economy, 28(5), 1572-1588.
UNDP. 2016. Human Development Report 2016.
https://hdr.undp.org/content/huma n-development-report-2016?gad_source=1&gclid=CjwKCAi AvdCrBhBREiwAX6-6Unu0NG7IysJiR9v67M_3ld4dXZ0uh pSmMZhkx4JjPbi29DnmghOJFxoCito QAvD_BwE
Wisnumurti, A. A. G. O., Darma, I. K., & Suasih, N. N. R. 2018. Government policy of Indonesia to managing demographic bonus and creating
Indonesia gold in 2045. Journal Of Humanities And Social Science (IOSR-JHSS), 23(1), 23-34.
Wulandari, S., Dasopang, A. P., Rawani, G. A., Hasfizetty, I., Sofian, M. Y., Dwijaya, R., & Rachmalija, S. (2022). Kebijakan Anti Kemiskinan Program Pemerintah dalam
Penananggulangan Kemiskinan di Indonesia. Jurnal Inovasi Penelitian, 2(10), 3209-3218.
Wu, S. Y., Tang, J. H., & Lin, E. S., 2010. The impact of government expenditure on economic growth: How sensitive to the level of development?. Journal of Policy Modeling, 32(6), 804-817.
107
Discussion and feedback