Testing of January Effect on the Indonesian Capital Market: Sectoral Analysis of GARCH Models
on
I Gusti Bagus Wiksuana, Testing of January Effect on the Indonesian …
MATRIK: JURNAL MANAJEMEN, STRATEGI BISNIS DAN KEWIRAUSAHAAN
Homepage: https://ojs.unud.ac.id/index.php/jmbk/index
Vol. 15 No. 2, Agustus (2021), 303-325
Testing of January Effect on the Indonesian Capital Market:
Sectoral Analysis of GARCH Models
I Gusti Bagus Wiksuana1) , Ni Ketut Purnawati 2),
I Made Surya Negara Sudirman3)
1,2 Faculty of Economics and Business Udayana University
Bali, Indonesia
email: [email protected]
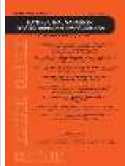

SINTA 2
DOI : https://doi.org/10.24843/MATRIK:JMBK.2021.v15.i02.p12
ABSTRACT
January Effect is the stock returns in January are higher than other months. This study uses the Generalized Autoregresive Conditional Heteroscedasticity (GARCH) method to test and analyze the January Effect in the Indonesian capital market from 2000 up to 2018. The novelty of this study is a research methodology that classifies samples by sector and period on the January Effect test. The results of this study indicate that the January Effect occurs in several sectors and periods. The January Effect occurs in the mining sector, consumer goods sector, property sector, and trade sector. Thus, the results of this study confirm the tax loss selling hypothesis and window dressing hypothesis in several sectors and observation periods. This shows that the January Effect is related to the characteristics of the sector and the period of observation.
Keywords: January Effect, GARCH Models, Indonesian Capital Market.
Pengujian January Effect di Pasar Modal Indonesia: Analisis
Sektoral Model GARCH
ABSTRAK
Efek Januari adalah return saham di bulan Januari lebih tinggi dibandingkan bulan-bulan lainnya. Penelitian ini menggunakan metode Generalized Autoregresive Conditional Heteroscedasticity (GARCH) untuk menguji dan menganalisis January Effect di pasar modal Indonesia sejak tahun 2000 s.d. 2018. Kebaruan penelitian ini adalah metodologi penelitian yang mengklasifikasikan sampel berdasarkan sektor dan periode pada uji January Effect. Hasil penelitian ini menunjukkan bahwa January Effect terjadi pada beberapa sektor dan periode. Efek Januari terjadi pada sektor pertambangan, sektor barang konsumsi, sektor properti, dan sektor perdagangan. Dengan demikian, hasil penelitian ini mengkonfirmasi hipotesis penjualan rugi pajak dan hipotesis window dressing di beberapa sektor dan periode pengamatan. Hal ini menunjukkan bahwa January Effect berkaitan dengan karakteristik sektor dan periode pengamatan.
Kata Kunci: Efek Januari, GARCH Models, Pasar Modal Indonesia
INTRODUCTION
The January effect is a phenomenon where stock returns in January are higher than other months of the year (Moller & Zilca, 2008). In the framework of Efficient Capital Market (EMH), stock returns in January should not be higher than in other months, because stock prices 313
should move to follow a random walk pattern so it is impossible to predict stock prices based on historical data of the stock prices (Fama, 1965, 1970). However, most of the study results found that stock returns in January were higher than other months on the capital markets in various countries (Gultekin & Glutekin, 1983; Seif, Docherty, & Shamsuddin, 2017; Yakob, Beal, & Delpachitra, 2005). The implication of this phenomenon is that capital markets become inefficient. Where it is possible for investors to obtain an abnormal return by considering January in stock trading (Sun & Tong, 2010). The potential for abnormal return in January indicates a higher risk premium in January, so January effect actually challenges the concept of the Capital Asset Pricing Model (Lintner, 1965; Mossin, 1966; Sharpe, 1964) which does not take into account seasonal patterns in risk premiums (Tinic & West, 1984). Thus, January effect is an anomaly that challenges some basic concepts in conventional financial theory (Thaler, 1987).
January effect has been widely studied after this phenomenon was first revealed by Rozeff & Kinney (1976). January Effect study was conducted in the American capital market (Gu, 2003; Keim, 1983; Moller & Zilca, 2008; Reinganum, 1983; Robins & Smith, 2017; Rozeff & Kinney, 1976), Canadian capital market (Athanassakos, 1992; Berges, Mcconnell, & Schlarbaum, 1984), Japanese capital market (Kato & Schallheim, 1985), Australian capital market (Brown, Keim, Kleidon, & Marsh, 1983), Pakistan capital market (Ullah & Ullah, 2001), Turkish capital market (Eyuboglu & Euboglu, 2016). Some researchers also conducted January Effect study in several countries, among others are 17 countries (Gultekin & Glutekin, 1983), capital markets in the Asia Pacific (Yakob et al., 2005), di in several developing capital markets (Seif et al., 2017), in four countries with a majority of Muslim population namely Indonesia, Jordan, Turkey and Pakistan. (Halari, Helliar, & Power, 2018)
The novelty in this research is the January Effect testing and analysis conducted in each sector in Indonesian Stock Exchange. Previous researchers have not considered the January Effect testing and analysis on various sectors contained in the capital market in their research. Where, there are nine sectors on the Indonesian Stock Exchange, among others: the mining sector; basic chemical industry sector; various industrial sectors; consumer goods industry sector; the property, real estate and building construction sector; infrastructure, utilities, and transportation sectors; financial sector; and the trade, services and investment sectors. This study examines and analyzes the January Effect in all nine sectors.
RESEARCH METHODS
The population of this study is the stock price index of each sector in the Indonesian Stock Exchange. The nine sectors on the Indonesian Stock Exchange include: the mining sector; basic chemical industry sector; various industrial sectors; consumer goods industry sector; the property, real estate and building construction sector; infrastructure, utilities, and transportation sectors; financial sector; and the trade, services and investment sectors. The sample of this study is the stock price index of each sector in Indonesian Stock Exchange from 2000 to 2018. The sample determining - method in this study uses a purposive sampling method (Sekaran & Bougie, 2016:248), where the criteria used are sample period when the stock market did not experience a crisis. The period of 2000 was a period after the 1998 economic crisis, the stock market situation has gradually improved. During the period 2000 to 2018 there was no economic crisis in Indonesia.
Variables measured by nominal scale in this study are stock trading months in a year, namely January, February, March, April, May, June, July, August, September, October, November, and December. The variable measured by the ratio scale in this study is the market return of the capital market sectoral index on the Jakarta Stock Exchange. The stock market
returns of each sector are calculated using the following equation.
p _ Pt-Pt-1
1
Pt-1
Where,
R = daily market return of each sector on Indonesian Stock Exchange
Pt = sectoral stock price index in t of each sector on Indonesian Stock Exchange
Pt-1 = sectoral stock price index in t-1 of each sector on Indonesian Stock Exchange
Data analysis in this study using the GARCH Model. Where the descriptive analysis which was a prerequisite of the GARCH model was previously conducted that included data normality testing, stationarity data testing and heteroscedasticity testing. Data normality testing is done by looking at statistical statistics. Data stationarity testing was performed with the Augmented Dickey-Fuller Test (ADF) (Dickey & Fuller, 1979) and Philips-Perron Test (PP) (Philip & Perron, 1988). Heteroscedasticity testing was performed with the Lagrange Multiplier Test (LM) (Engle, 1982). The GARCH model requires data to be stationary and contain heteroscedasticity.
The GARCH model examines the effect of the stock trading month in a year (January, February, March, April, May, June, July, August, September, October, November, and December) on the stock market returns in each sector on Indonesian Stock Exchange. yt = δ1D1t + δ2D2t + δ3D3t + δ4D4t + δ5D5t + δ6D6t + δ7D7t + δ8D8t +
δ9D9t + δ10D10t + δ11D11t + δ12D12t + εt(2)
Ψt-1
ht = Y1D1t + Y2D2t + γ3D3t + γ4D4t + γ5D5t + Y6D6t + Y7D7t + YsD8t +
Y9D9t + Y10D10t + Y11D11t + Y12D12t + ∑vj=4βjht-4 + ∑j=1 ajεt2-j(4)
where yt is a linearly related stock return for an explanatory dummy variable vector (Dt) and an error term (εt) that depends on previous information (ψt-1); ht is conditional variance. Error term is assumed to follow the conditional student-t density (t.d.) with v degrees of the zero approach. Thus, in this research model, error distribution can be conditionally heteroscedasctic and non-normal.
The dummy variable (Ddt) in the return and variance equation shows twelve months in a year. In other words, Ddt is equal to one if t is January (d = 1), and the other month is zero. Likewise, Ddt sama is equal to one or nonzero if month t is February (d = 2), and so on. The coefficients δ1 to δ12 in equation 2 show the magnitude and direction of the effect on each month of the year on stock returns. In other words, the coefficients δ1, δ2, δ3, δ4, δ5, δ6, δ7, δ8 , δ9, δ10, δ11 and δ12 show January effects, February effects, March effects, April effects, May effects, June effects, July effects, July effects, August effects, September effects, October effects, November effects and December effects on each sectoral stock return in Indonesian Stock Exchange. So do the coefficients Y1 to Y12 which indicate the magnitude and direction of monthly effect volatility. In general, the study found the January dummy coefficient (δ1) was positive and significantly different from zero.
RESULTS AND DISCUSSION
This study examines and analyzes January Effect on all nine sectors on Indonesian Stock Exchange from 2000 to 2018. All of these sectors include, among others, agribusiness, mining, property, finance, trade, basic industry, misc industry, consumer goods, and
infrastructure. Descriptive statistics of the nine sectoral sectors can be seen in Table 1 and Table 2.
Table 1. Descriptive Statistics of Daily Returns for Agribusiness, Mining, Property, Financial and Trading Sectors in Indonesian Capital Market
Indicator |
Agribusiness |
Mining |
Property |
Financial |
Trade |
Mean |
0,000599 |
0,000699 |
0,000576 |
0,000779 |
0,000389 |
Median |
-0,000200 |
0,000300 |
0,000400 |
0,000700 |
0,000600 |
Maximum |
0,147600 |
0,122700 |
0,099400 |
0,109600 |
0,075100 |
Minimum |
-0,194200 |
-0,222500 |
-0,126800 |
-0,104000 |
-0,167200 |
Std, Dev, |
0,021270 |
0,020220 |
0,015765 |
0,015918 |
0,013850 |
Skewness |
0,302850 |
-0,060179 |
-0,166283 |
0,027215 |
-0,740823 |
Kurtosis |
10,07750 |
10,39488 |
8,390076 |
7,007911 |
11,81855 |
Jarque-Bera |
9702,636 |
10518,11 |
5607,902 |
3089,419 |
15376,06 |
Probability |
0,000000 |
0,000000 |
0,000000 |
0,000000 |
0,000000 |
Observations |
4.615 |
4.615 |
4.615 |
4.615 |
4.615 |
Table 2 . Results of Daily Descriptive Statistics of Basic Industry, Misc Industry, Consumer Goods, and Infrastructure Sectors in Indonesian Capital Market
Indicator |
Basic Industry |
Misc Industry |
Consumer Goods |
Infrastructure |
Mean |
0,000540 |
0,000694 |
0,000651 |
0,000590 |
Median |
0,000500 |
0,000200 |
0,000500 |
0,000400 |
Maximum |
0,101000 |
0,142500 |
0,088800 |
0,250700 |
Minimum |
-0,117600 |
-0,155100 |
-0,099500 |
-0,184500 |
Std, Dev, |
0,016093 |
0,019386 |
0,014255 |
0,018419 |
Skewness |
-0,323384 |
0,219885 |
-0,038836 |
0,370270 |
Kurtosis |
7,037241 |
7,480471 |
7,134378 |
17,04971 |
Jarque-Bera |
3214,659 |
3897,371 |
3288,017 |
38062,72 |
Probability |
0,000000 |
0,000000 |
0.000000 |
0,000000 |
Observations |
4.615 |
4.6 15 |
4.615 |
4.615 |
Based on the results of descriptive analysis in Table 1 and Table 2, the sectors with the largest daily returns to the smallest in a row are consumer goods, financial, mining, misc industry, agribusiness, infrastructure, property, basic industry and trading. The largest daily return value is the infrastructure sector and the smallest is the mining sector. The high standard deviation value is in agribusiness sector and the lowest is in trade sector. A negative skewness value indicates a tendency for daily returns which is greater than the mean to occur more than those whose daily returns are smaller than the mean. On the other hand, a positive skewness value indicates a tendency for daily returns which is smaller than the mean to occur more than daily return that is greater than the mean. The kurtosis value of each sector is greater than three, which implies that the daily return of each sector is not normally distributed as indicated by the value of probability of Jarque-Bera <5%. The number of daily return observations of each sector is 4,615.
Table 3 shows the presence of unit root in the daily return data of each sector tested by Augmented Dikey-Fuller (ADF) and Philip Perron (PP). Based on the table, the values of p ADF and PP are less than 5%. Both the ADF and PP tests reject the null hypothesis at a significance level of less than 1%. Thus, the test results confirm that the daily return data of each sector on Indonesian Stock Exchange is stationary.
Table 3. Daily Unit Root Return Test Results for Agribusiness, Mining, Property, Financial, Trading, Basic Industry, Misc Industry, Consumer Goods, and Infrastructure Sectors in Indonesian Capital Market
No. |
Sectors |
t-Statistik ADF |
Prob. |
t-Statistik PP |
Prob. |
1. |
Agribusiness |
-62.84558 |
*** |
-62.90128 |
*** |
2. |
Mining |
-61.93859 |
*** |
-62.03660 |
*** |
3. |
Property |
-62.46718 |
*** |
-63.22266 |
*** |
4. |
Financial |
-62.55426 |
*** |
-62.34564 |
*** |
5. |
Trade |
-64.31919 |
*** |
-64.36335 |
*** |
6. |
Basic Industry |
-62.95947 |
*** |
-62.90065 |
*** |
7. |
Misc Industry |
-36.67583 |
*** |
-63.07156 |
*** |
8. |
Consumer Goods |
-75.00903 |
*** |
-75.01258 |
*** |
9. |
Infrastructure |
-51.52467 |
*** |
-70.08679 |
*** |
*** Significant <1%
ARCH test is used to detect the presence or absence of the ARCH effect on the time series of capital market returns. Table 4 and Table 5 show the results of the ARCH test which were very significant.
Table 4. The ARCH Daily Return Test Results for Agribusiness, Mining, Property, Financial and Trading Sectors in Indonesian Capital Market
Indicators |
Agribusiness |
Mining |
Property |
Financial |
Trading |
ARCH |
131.1645 |
135.1987 |
87.88160 |
344.9128 |
88.89688 |
Prob. (1) |
0.0000 |
0.0000 |
0.0000 |
0.0000 |
0.0000 |
Table 5 . The ARCH Daily Return Test Results for Basic Industry, Misc Industry, Consumer Goods, and Infrastructure Sectors in Indonesian Capital Market
Indicators |
Basic Industry |
Misc Industry |
Consumer Goods |
Infrastructure |
ARCH |
155.9092 |
333.5423 |
232.5903 |
526.1794 |
Prob. (1) |
0.0000 |
0.0000 |
0.0000 |
0.0000 |
Where the p value is less than 0.01, meaning the null hypothesis which states "there is no ARCH effect" is rejected at the 1% significance level. Thus, in each sector on Indonesian Stock Exchange, there is an ARCH effect so that the GARCH Model can be estimated.
Based on the test results, it is known that the January Effect occurs in mining sector, trade sector, consumer goods sector, while the January effect does not occur in other sectors. For this reason, January Effect will be discussed in each of these sectors.
The January Effect test results for mining sector are shown in Table 6. Based on the table we can find out the January Effect coefficient value in the mean equation for the fullsample period (04/1/2000 until 12/28/2018), and sub-sample 1 (04 / 1/2000 to 12/30/2009) significant (p <10%). Meanwhile, for the sub-sample period 2 (04/01/2010 to 28/12/2018) the value of the January Effect coefficient on the mean equation is not significant (p> 10%). January Effect coefficient value in the testing period of the full-sample period (04/1/2000 until 12/28/2018), sub-sample 1 (04/1/2000 until 12/30/2009) and sub-sample 2 (04 / 01/2010 till 12/28/2018) respectively were 0.001543, 0.002882 and 0.000789.
Table 6. The Estimation Results of the GARCH Model (1.1) in Mining Sector
Mining Sector
Begin date End date |
Full-Sample 04/01/2000 28/12/2018 |
Sub-Sample 1 04/01/2000 30/12/2009 |
Sub-Sample 2 04/01/2010 28/12/2018 |
Variable | |||
Mean | |||
January |
0,001543 |
** 0,002882 |
** 0,000789 |
February |
0,000655 |
0,001199 |
0,000516 |
March |
0,000166 |
0,000467 |
0,000140 |
April |
0,000583 |
0,001039 |
0,000451 |
May |
-0,000197 |
0,002822 |
** -0,001709 ** |
June |
0,000249 |
0,001941 |
-0,000547 |
July |
0,000578 |
0,000761 |
0,000446 |
August |
-3,96E-05 |
0,000310 |
-0,000125 |
September |
0,001012 |
0,001010 |
0,000926 |
October |
0,000483 |
0,000258 |
0,000665 |
November |
-0,000894 |
0,000722 |
-0,001747 ** |
December |
0,000861 |
0,002524 |
* 3,76E-05 |
Variance | |||
Constant (ω) |
6,36E-06 |
*** 1,20E-05 |
*** 1,19E-05 *** |
ARCH Effect (α) |
0,095484 |
*** 0,091727 |
*** 0,106697 *** |
GARCH Effect (β) |
0,892042 |
*** 0,893795 |
*** 0,832723 *** |
T-DIST. DOF |
5,452809 |
*** 4,892875 |
*** 7,038643 *** |
R-squared |
0,000647 |
0,002025 |
0,004763 |
ARCH-LM Test for heteroscedasticity | |||
ARCH-LM test statistics |
0,509310 |
0,825528 |
0,205921 |
Autocorrelation & Partial Correlation | |||
Autocorrelation (AC) |
10 -0,000 |
-0,003 |
0,006 |
20 -0,005 |
-0,010 |
0,004 | |
30 -0,012 |
0,008 |
0,009 | |
Partial Correlation(PAC) |
10 -0,001 |
-0,004 |
0,005 |
20 -0,005 |
-0,012 |
0,005 | |
30 -0.010 |
0,005 |
0,008 |
*significant at 10%, **significant at 5%, *** significant at 10%, - not significant
Table 6 also shows that there is an ARCH-GARCH Effect which is characterized by a significant coefficient of the ARCH Effect (α) and GARCH Effect (β) with a p-value <1%. The estimation model in each testing period does not contain heteroscedasticity which is indicated by Prob value. Chi-square of each equation is greater than 5% for each ARCH test statistics value. In addition, the estimation model in each testing period also does not contain autocorrelation which is marked by each autocorrelation (AC) and partial correlation (PAC) significance values greater than 5%. Thus, it can be concluded that there was a January Effect in mining sector in the full-sample period (04/1/2000 until 28/12/2018), and sub-sample 1 (04/1/2000 until 30/12/2009).
The January Effect test results for trade sector are shown in Table 7. Based on Table 7 we can find out that the January Effect coefficient values in the mean equation for the fullsample period (04/1/2000 until 12/28/2018) and sub-sample 1 (04 / 1/2000 to 30/12/2009) are significant with a p value less than 10%. Meanwhile, for the sub-sample period 2 (01/04/2010 to 28/12/2018), the January Effect coefficient value on the mean equation is not significant with a p value greater than 10%. The January Effect coefficient value in the testing period of the full-sample period (04/1/2000 until 12/28/2018), sub-sample 1 (04/1/2000 until
12/30/2009) and sub-sample 2 (04 / 01 / 2010 until 12/28/2018) respectively are 0.001092, 0.001558 and 0.000785.
Table 7. Estimation Results of the GARCH Model (1,1) in Trade Sector
Begin date End date |
Trade Sector | ||
Full-Sample 04/01/2000 28/12/2018 |
Sub-Sample 1 04/01/2000 30/12/2009 |
Sub-Sample 2 04/01/2010 28/12/2018 | |
Variable | |||
Mean | |||
January |
0,001092 |
** 0,001558 |
* 0,000785 |
February |
0,001442 |
*** -0,000159 |
0,002407 *** |
March |
0,001336 |
*** 0,001270 |
0,001338 ** |
April |
0,001036 |
* 0,002123 |
** 0,000668 |
May |
0,000719 |
0,002348 |
** -0,000131 |
June |
0,001050 |
* 0,001588 |
* 0,000639 |
July |
0,001056 |
** 0,000947 |
0,001113 * |
August |
-0,000627 |
-0,000972 |
-0,000463 |
September |
0,000985 |
* 0,001270 |
** 0,000667 |
October |
0,000449 |
0,000209 |
0,000518 |
November |
-0,000415 |
-7,74E-07 |
** -0,000703 |
December |
0,001275 |
** 0,002245 |
** 0,000686 |
Variance | |||
Constant (ω) |
6,04E-06 |
*** 2,22E-05 |
*** 5,43E-06 *** |
ARCH Effect (α) |
0,120145 |
*** 0,153738 |
*** 0,104358 *** |
GARCH Effect (β) |
0,848619 |
*** 0,760852 |
*** 0,842010 *** |
T-DIST. DOF |
5,930489 |
*** 5,569100 |
*** 7,113101 *** |
R-squared |
0,002981 |
0,002878 |
0,007274 |
ARCH-LM Test for heteroscedasticity | |||
ARCH-LM test statistics |
2,931492 |
* 0,152628 |
2,781177 * |
Autocorrelation & Partial Correlation | |||
Autocorrelation (AC) 10 |
-0,006 |
-0,008 |
0,005 |
20 |
0,009 |
0,017 |
0,016 |
30 |
-0,021 |
-0,022 |
-0,003 |
Partial Correlation(PAC) 10 |
-0,008 |
-0,009 |
0,002 |
20 |
0,011 |
0,018 |
0,018 |
30 |
-0.021 |
-0,024 |
0,006 |
*significant at 10%, **significant at 5%, |
*** significant at |
10%, - not significant |
Table 7 also shows that there is an ARCH-GARCH Effect which is characterized by a significant coefficient of ARCH Effect (α) and GARCH Effect (β) with a p-value <1%. The estimation model in each testing period does not contain heteroscedasticity which is indicated by Prob value. Chi-square of each equation is greater than 5% for each ARCH test statistics value. In addition, the estimation model in each testing period also does not contain autocorrelation which is marked by the respective autocorrelation (AC) and partial correlation (PAC) values greater than 5%. Thus, it can be concluded that there was a January Effect in trade sector in the full-sample period (04/1/2000 until 28/12/2018) and sub-sample 1 (04/1/2000 until 30/12/2009).
January Effect test results for the consumer goods sector are shown in Table 8. Based on the table, it can be seen the value of the January Effect coefficient in the mean equation for the sub-sample period 1 (04/1/2000 to 12/30/2009) significant with a p value <10 %. Meanwhile, the January Effect coefficient value on the mean equation for the full-sample period (04/1/2000 until 12/28/2018) and sub-sample 2 (04/01/2010 until 12/28/2018) is not significant with the p value greater than 10%. The January Effect coefficient value in the testing period full-sample
period (04/1/2000 until 12/28/2018), sub-sample 1 (04/1/2000 until 12/30/2009) and subsample 2 (04 / 01/2010 till 12/28/2018) respectively were 0.001330, 0.001897 and 0.000750. Table 8 also shows that there is an ARCH-GARCH Effect which is characterized by a significant coefficient of ARCH Effect (α) and GARCH Effect (β) with a p-value less than 1%. The estimation model in the full-sample period (04/1/2000 until 28/12/2018) contains heteroscedasticity which is indicated by Prob. Chi-sqare equation is less than 1% for ARCH test statistics. Meanwhile, the estimation model in the sub-sample 1 (04/1/2000 to 30/12/2009) and sub-sample 2 (04/01/2010 to 28/12/2018) does not contain heteroscedasticity which is indicated by Prob value. Chi-suqare each equation is greater than 5% for each ARCH test statistics value. In addition, the estimation model in the full-sample period (04/1/2000 to 28/12/2018) contains autocorrelation characterized by a significant value of autocorrelation (AC) and partial correlation (PAC) values less than 5%. Meanwhile, the estimation model in the sub-sample 1 period (04/1/2000 until 12/30/2009) and sub-sample 2 (04/01/2010 until 12/28/2018) does not contain autocorrelation marked with each autocorrelation (AC) and partial correlation (PAC) values were significantly greater than 5%. Thus, it can be concluded that there was a January Effect in the finance sector in sub-sample 2 (04/01/2010 to 28/12/2018).
Table 8. Estimation Results of GARCH Model (1.1) in Consumer Goods Sector Consumer Goods
Begin date End date |
Full-Sample 04/01/2000 28/12/2018 |
Sub-Sample 1 04/01/2000 30/12/2009 |
Sub-Sample 2 04/01/2010 28/12/2018 | ||||
Variable | |||||||
Mean | |||||||
January |
0,001330 |
0,001897 |
** |
0,000750 | |||
February |
0,000171 |
-0,000335 |
0,001329 |
* | |||
March |
0,000191 |
-0,000250 |
0,000642 | ||||
April |
0,001480 |
0,001616 |
* |
0,001211 |
* | ||
May |
0,001343 |
0,002285 |
*** |
0,000702 | |||
June |
0,001262 |
0,001291 |
0,000708 | ||||
July |
0,000821 |
0,000986 |
0,001046 | ||||
August |
-0,000758 |
-0,001131 |
0,000354 | ||||
September |
0,000193 |
0,000639 |
0,001025 | ||||
October |
-0,000438 |
0,000167 |
6,11E-05 | ||||
November |
0,000878 |
0,001871 |
** |
0,000494 | |||
December |
0,001466 |
0,001733 |
** |
0,000926 | |||
Variance | |||||||
Constant (ω) |
0,000203 |
*** |
2,58E-05 |
*** |
8,33E-06 |
*** | |
ARCH Effect (α) |
0,150000 |
*** |
0,174192 |
*** |
0,126575 |
*** | |
GARCH Effect (β) |
0,600000 |
*** |
0,719683 |
*** |
0,830427 |
*** | |
T-DIST. DOF |
20,00000 |
*** |
5,098460 |
*** |
6,269377 |
*** | |
R-squared |
0,002706 |
0,005187 |
0,000656 | ||||
ARCH-LM Test for heteroscedasticity | |||||||
ARCH-LM test statistics |
35,48100 |
*** |
0,008546 |
0,042544 | |||
Autocorrelation & Partial Correlation | |||||||
Autocorrelation (AC) |
10 |
0,082 |
*** |
0,025 |
-0,015 | ||
20 |
0,013 |
*** |
-0,022 |
0,001 | |||
30 |
0,028 |
*** |
0,016 |
0,021 | |||
Partial Correlation(PAC) |
10 |
0,063 |
*** |
0,024 |
-0,015 | ||
20 |
-0,008 |
*** |
-0,021 |
0,001 | |||
30 |
0.011 |
*** |
0,016 |
0.024 |
*significant at 10%, **significant at 5%, *** significant at 10%, - not significant
The main contribution of this research is the sectoral analysis of the January Effect in Indonesian capital market. Where each sector has a different concentration that has consequences on different risk premiums. Companies in the concentrated sector get lower returns because the highly concentrated sector has high barriers for new companies to enter. As a result, there is no incentive for companies to innovate. Lack of innovation makes companies in concentrated sectors less risky. Therefore, shares of companies in concentrated industries provide lower returns (Hou & Robinson, 2006).
There are three factors that determine the prospects of the company, namely the specific characteristics of the company, economic conditions and the industrial structure where the company is located (Chou, Ho, & Ko, 2012). Companies from different sectors have different sensitivity to the business cycle. This has implications for sector differences which may contain differences in the level of availability of a country's economic information, which is characterized by an industry portfolio that can be used to predict several macroeconomic variables (Lamont, 2001). The role of information processing capacities for asset prices is also different for each sector. Where investors can have limited rationality and do not have the capability to process information. Investors tend not to process all information for all shares traded, but only process information for a few shares that have information only. This has implications for investors to form a portfolio consisting of shares in which information is owned and investors only compare information from stocks in certain sectors for which information is owned (Merton, 1987).
The January Effect statistical test results on all nine sectors in the Indonesian capital market show that January Effect occurs in several sectors at a certain time period. These sectors include: mining, property, trade, and consumer goods. January Effect occurred in the mining sector in full-sample (04/1/2000 until 28/12/2018) and sub-sample 1 (04/1/2000 until 30/12/2009); in the property sector in sub-sample 2 (04/01/2010 to 28/12/2018); in the trade sector in full-sample (04/1/2000 until 28-28/2018) and sub-sample 1 (04/1/2000 until 30/12/2009); in the consumer goods sector in sub-sample 1 (04/1/2000 until 30/12/2009). Thus, the findings in this study support the tax loss selling hypothesis and window dressing hypothesis in several sectors and observation periods. This shows the January Effect fact is also related to the type of sector and observation period. Where each sector has different levels of industry competition and company maturity that can have implications for variations in potential returns and risks. Finally, variations in potential returns and risks in sectoral classifications and specific time periods determine investor behavior in making investment decisions that have consequences on January Effect existence.
The window dressing hypothesis states that institutional investors use the "window dressing" technique at the end of the fiscal year to improve portfolio performance ahead of the performance evaluation report. Improved portfolio performance is generally done by institutional investors by rebalancing the portfolio before the end of the fiscal year 31 December. Institutional investors generally sell shares of small companies that are performing poorly at the end of the year, to improve the performance of their portfolio. Sales of shares of small companies that are performing poorly, making the company's stock prices decline further. After evaluating the performance of institutional investors as of December 31, in January institutional investors return to buy shares of small companies whose share prices are relatively cheap because they have previously declined. In the end, selling pressure at the end of the year followed by buying pressure at the beginning of the year generates January Effect identified with the share prices of small companies. Window dressing carried out by institutional investors with rebalancing portfolio has implications for portfolios consisting of shares of small
companies outperforming the market in January (Porter, Powell, & Weaver, 1996; Ritter & Chopra, 1989).
The tax loss selling hypothesis states that investors are trying to reduce the taxes they bear by realizing losses at the end of the year so that it has implications for further falling stock prices. If the share price continues to decline below its book value or undervalue just because of the motive to reduce taxes, then at the beginning of the year or in January these shares will be attractive for investors to buy. The increasing demand for the company's shares caused the price of the company's shares to rise again in January so that the January Effect (Reinganum, 1983; Watchel, 1942). occurs. The magnitude of January Effect is determined by investor sensitivity to tax regulations in the country concerned, as well as the characteristics of companies in the investor portfolio. Investor sensitivity to tax regulations in the country concerned is also related to the characteristics of existing investors. While most investors are institutional investors with relatively large funding capabilities, then the investment choice tends to be in stocks with large market capitalization at relatively high prices. Shares with large market capitalization tend to provide more stable profits than small companies. Whereas when most investors are individual investors who have relatively small funding abilities, the investment choices tend to be on stocks that have small market capitalization at relatively cheap prices. Shares with small market capitalization tend to provide unstable profits compared to large companies. Apart from the relatively low share prices of small companies, individual investors choose to invest in small companies as well because they expect the share price to increase more than the price of other companies' shares in the future. Individual investors also have limitations in possessing and processing information so that they are vulnerable to noise trading behavior that can increase the magnitude of the January Effect. The irrational retail behavior hypothesis argues that retail investors tend to do noise trading based on their irrational beliefs, then causing stock prices to deviate from their fundamental values over a long period of time (De Long, Shleifer, Summers, & Robert, 1990).
Thus, the microtructure market of a stock market determines the presence and magnitude of January Effect. This was confirmed by Gultekin & Glutekin (1983) who found that the January Effect on the capital market in the United States was smaller than in many other countries. The magnitude of the January effect in this study ranges from the largest to the smallest in a row occurred in the mining sector in sub-sample 1, the consumer goods sector in sub-sample l, the property sector in sub-sample 2, the trade sector in sub-sample 1, the mining sector in fullsample and the trade sector in full-sample.
Until now, the January Effect is an anomaly that has not been able to be fully explained by various explanations proposed by previous researchers. January Effect testing in this study is based on the explanation of the tax loss selling hypothesis, window dressing hypothesis, irrational retail behavior hypothesis. Although the three hypotheses have not been able to explain the January Effect in full, but the explanation of the two hypotheses is most acceptable to researchers to date.
This study was designed by including sectoral classification and time period classification in the estimation model which is expected to provide a deeper explanation related to the existence of January Effect. The latest estimation method, GARCH model, which used in the analysis of this research data is expected to strengthen the validity of the explanation without being distorted by heteroscedasticity and autocorrelation problems that are characteristic of daily stock return data.
Based on the research discussion, it is important to consider the sectoral classification and research period in developing theoretical models based on the tax loss selling hypothesis, window dressing hypothesis, irrational retail behavior hypothesis in the January Effect test.
Where each sector has a different level of competition and is dynamic in a certain timeframe that can have consequences on differences in risk premiums in each sector.
CONCLUSSION
Based on the results and discussion, the conclusion of this research is that there is a January Effect in several sectors in a certain period in Indonesian capital market. These sectors include: mining, property, trade, and consumer goods. Thus, the findings in this study support the tax loss selling hypothesis and window dressing hypothesis in several sectors and observation periods. This shows a fact that January Effect is also related to the type of sector and observation period. Where each sector has different levels of industry competition and company maturity that can have implications for variations in potential returns and risks. Finally, variations in potential returns and risks in sectoral classifications and specific time periods determine variations in investor behavior in making investment decisions that have consequences for the existence and magnitude of January Effect. January Effect in this study, from the largest to the smallest in a row occurred in the mining sector in sub-sample 1, the consumer goods sector in sub-sample l, the property sector in sub-sample 2, the trade sector in sub-sample 1, the mining sector in full-sample and trade sector in full-sample.
REFERENCE
Athanassakos, G. (1992). Portfolio Rebalancing and the January Effect in Canada. Financial Analysts Journal, 48(6), 67–78.
Berges, A., Mcconnell, J. J., & Schlarbaum, G. G. (1984). The Turn-of-the-Year in Canada. The Journal of Finance, XXXIX(1), 185–192.
Brown, P., Keim, D. B., Kleidon, A. W., & Marsh, T. A. (1983). Stock Return Seasonalities and The Tax-Loss Selling Hypothesis: Analysis of the Arguments and Australian Evidence. Journal of Financial Economics, 12(1), 105–127.
Chou, P., Ho, P., & Ko, K. (2012). Do Industries Matter in Explaining Stock Returns and AssetPricing Anomalies ? Journal of Banking and Finance, 36(2), 355-370.
https://doi.org/10.1016/j.jbankfin.2011.07.016
De Long, J. B., Shleifer, A., Summers, L. H., & Robert, J. W. (1990). Noise Trader Risk in Financial Markets Andrei Shleifer. Journal of Political Economy, 98(4), 703–738.
Dickey, D. A., & Fuller, W. A. (1979). Distribution of the Estimators for Autoregressive Time Series With a Unit Root. Distribution of the Estimators for Autoregressive Time Series With a Unit Root., 74(366), 427–431.
Engle, R. F. (1982). Autoregressive Conditional Heteroscedasticity with Estimates of the Variance of United Kingdom Inflation. Econometrica, 50(4), 987–1007.
Eyuboglu, K., & Euboglu, S. (2016). Examining the January Effect in Borsa Istanbul Sector and Sub- Sector Indices. International Journal of Economic Perspectives, 10(2), 102–109.
Fama, E. F. (1965). The Behavior of Stock-Market Prices. The Journal of Business, 38(1), 34– 105.
Fama, E. F. (1970). Efficient Capital Markets: A Review of Theory and Empirical Work. The Journal of Finance, 25(2), 28–30.
Gu, A. Y. (2003). The Declining January Effect: Evidences From the U . S . Equity Markets. The Quarterly Review of Economics and Finance, 43(2), 395–404.
Gultekin, M. N., & Glutekin, N. B. (1983). Stock Market Seasonality: International Evidence. Journal of Financial Economics, 12(4), 469–481.
Halari, A., Helliar, C., & Power, D. M. (2018). Taking Advantage of Ramadan dan January in 323
Muslim Countries. Quarterly Review of Economics and Finance. https://doi.org/10.1016/j.qref.2018.05.018
Hou, K., & Robinson, D. T. (2006). Industry Contentration and Average Stock Returns. The Journal of Finance, LXI(4), 1927–1956.
Kato, K., & Schallheim, J. S. (1985). Seasonal and Size Anomalies in the Japanese Stock Market. The Journal of Financial and Quantitative Analysis, 20(2), 243–260.
Keim, B. (1983). Size-Related Anomalies and Stock Return Seasonality. Journal of Financial Economics, 12(1), 13–32.
Lamont, O. A. (2001). Economic Tracking Portfolios. Journal of Econometrics, 105(1), 161– 184.
Lintner, J. (1965). Security Prices, Risk, and Maximal Gain From Diversification. The Journal of Finance, 20(4), 587–615.
Merton, R. C. (1987). American Finance Association A Simple Model of Capital Market Equilibrium with Incomplete Information. The Journal of Finance, 42(3), 483–510.
Moller, N., & Zilca, S. (2008). The Evolution of The January Effect. Journal of Banking & Finance, 32(3), 447–457. https://doi.org/10.1016/j.jbankfin.2007.06.009
Mossin, J. (1966). Equilibrium in a Capital Asset Market. Econometrica, 34(4), 768–783.
Philip, P. C. B. ., & Perron, P. (1988). Testing for a Unit Root in Time Series Regression. Biometrika, 75(2), 335–346.
Porter, D. C., Powell, G. E., & Weaver, D. G. (1996). Portfolio Rebalancing, Institutional Ownership, and The Small Firm-January Effect. Review of Financial Economics, 5(1), 12–29.
Reinganum, M. R. (1983). The Anomalous Stock Market Behavior of Small Firms in January: Empirical Test for Tax-Loss Selling Effects. Journal of Financial Economics, 12(June), 89–104.
Ritter, J. R., & Chopra, N. (1989). Portfolio Rebalancing and the Turn‐of‐the‐Year Effect. The Journal of Finance, XLIV(1), 149–166.
Robins, R. P., & Smith, G. P. (2017). On Unmodeled Breaks in the Turn of the Year, Turn of the Month, and January Effects. The Financial Review, 52(4), 725–747.
Rozeff, S. M., & Kinney, R. J. (1976). Capital Market Seasonality: The Case of Stock Returns.
Journal of Financial Economics, 3(4), 379–402.
Seif, M., Docherty, P., & Shamsuddin, A. (2017). Seasonal Anomalies in Advanced Emerging Stock Markets. The Quarterly Review of Economics and Finance, 66(November), 169– 181. https://doi.org/10.1016/j.qref.2017.02.009
Sekaran, U., & Bougie, R. (2016). Research Methods For Business: A Skill Building Approach (7th ed.). Chicester: John Wiley & Sons.
Sharpe, W. F. (1964). Capital Asset Prices: A Theory of Market Equilibrium under Conditions of Risk. The Journal of Finance, 19(3), 425–442.
Sun, Q., & Tong, W. H. S. (2010). Risk and the January effect. Journal of Banking and Finance, 34(5), 965–974. https://doi.org/10.1016/j.jbankfin.2009.10.005
Thaler, R. H. (1987). Anomalies: The January Effect. Journal of Economic Perspectives, 1(1), 197–201. Retrieved from http://www.jstor.org/stable/1942958 .
Tinic, S. M., & West, R. R. (1984). Risk and Return: January vs. the Rest of the Year. Journal of Financial Economics, 13(4), 561–574.
Ullah, I., & Ullah, S. (2001). Market Efficiency Anomalies: A Study of January Effect In Karachi Stock Market. Journal of Managerial Sciences, X(1), 31–44.
Watchel, S. B. (1942). Certain Observations on Seasonal Movements in Stock Prices. The Journal of Business of the University of Chicago, 15(2), 184–193.
Yakob, N. A., Beal, D., & Delpachitra, S. (2005). Seasonality in the Asia Pacific stock markets.
Journal of Asset Management, 6(4), 298–318.
325
Discussion and feedback