Determinants and Consequences of Student Learning Satisfaction During Covid-19 Pandemic
on
Jurnal Ilmiah Akuntansi dan Bisnis
Vol. 16 No. 1, January 2021
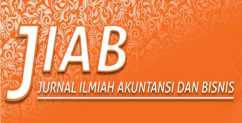
Determinants and Consequences of Student Learning Satisfaction During Covid-19 Pandemic
AFFILIATION:
1,2,3 Faculty of Administrative Sciences, Universitas Indonesia, Indonesia
*CORRESPONDENCE: [email protected]
THIS ARTICLE IS AVAILABLE IN: https://ojs.unud.ac.id/index.php/jiab
DOI:
10.24843/JIAB.2021.v16.i01.p01
CITATION:
Saragih, A. H., Adwie, J., & Hendrawan, A. (2021).
Determinants and Consequences of Students Learning Satisfaction During Pandemic Covid-19. Jurnal Ilmiah Akuntansi dan Bisnis, 16(1), 1-19.
ARTICLE HISTORY
Received:
07 November 2020
Revised:
22 December 2020
Accepted:
05 January 2021
Arfah Habib Saragih1*, Joansyah Adwie2, Adang Hendrawan3
Abstract
This study examines the effect of student perception in online learning on student satisfaction and its impact on student learning performance and intention to use in the future. The regression analysis method was used based on the survey results from 157 students. Results indicate that students' perceptions of online learning had a positive effect on student satisfaction, intention to use, and student learning performance. Further, student satisfaction has a positive effect on student learning performance but does not affect the intention to use. The implications for lecturers, students, and faculties are also discussed.
Keywords: student perception, student satisfaction, student performance, intention to use, covid-19 pandemic
Introduction
In 2020, Corona Virus Disease 2019 (Covid-19) begins to spread in Indonesian regions thus the Indonesian government issues several policies to prevent and constraint the spread of the virus. These policies are ranging from lockdowns in several areas to Large-Scale Social Restrictions (LSSR) recommended by the Ministry of Health. Regarding to this spread of Covid-19, the Ministry of Education and Culture provides advice on limiting face-to-face learning. Then, it is replaced by online learning which is implemented at the level of primary school to higher education. Several universities are quite responsive in responding to the policy of Ministry of Education and Culture, including Universitas Indonesia (UI). UI has issued some policies that need to be addressed and implemented to suppress the spread of Covid-19 starting from March 2020. One of these policies contains teaching and learning activities held by online (distance education). The implementation of distance education is carried out with the support of several applications such as Google Meet and Zoom. Keengwe & Georgina (2012) stated that the procedures for implementing teaching and learning activities can be influenced by technological advances. Thus, in the online learning process, students and lecturers play a crucial role in supporting the process. Students act as knowledge constructors while lecturers act as facilitators.
There are several studies that have investigated students' perceptions of online learning. Research reveals that online education is a process of providing education through computer media and online services (Chang et al., 2011). Online learning has a positive impact on student convenience and flexibility. Then refer to Davis (1985) who stated that ease of use and usability are important factors in assessing user acceptance. Davis argues that users' feelings about the usefulness of this system can have a positive effect on user performance. Then, the study was also conducted by Lee (2010) which states that users who are experienced in the use of technology such as computers and the internet show a higher level of satisfaction. Liaw & Huang (2013) also stated that students who are internet users feel a more positive impact on the implementation of learning. Furthermore, Lee & Mendlinger (2011) reported that chat rooms and online boards are some of the best tools that can increase student satisfaction when implementing online learning. The results of other studies show that positive feelings towards the acceptance of online learning by students can have a positive effect on student achievement (Lust et al., 2012).
This rapid change in the learning process that is driven by the Covid-19 pandemic conditions motivates the authors to conduct this research. This is important because it will have an impact on student performance and satisfaction in learning as well as the intention to use online learning in the future. This study offers a novelty in terms of the investigation of determinants and consequences of online learning during the Covid-19 pandemic which is still relatively rare in Indonesia. Therefore, there are several research questions regarding student responses related to perceptions of online learning and its impact on student satisfaction and performance. This study aims to give the description of student perceptions of online learning which includes five specific objectives: describing the effect of online learning perceptions on student learning satisfaction, intention to use online learning in the future, and student performance; then investigating the effect of student satisfaction on student performance and intention to use. This research has several contributions. First, this study adds the literature regarding online learning especially during Covid-19 pandemic. Second, this research is expected to provide additional empirical results as well as improve existing research using similar theories and hypotheses. Finally, the results of this study can be an input for educational institutions, faculty managers, lecturers and students.
Student satisfaction is one of the important factors in online learning. The level of satisfaction can be influenced by several factors such as class structure, educational activities, curriculum, lecturer competence, and facilities (Eichelberger & Ngo, 2018). Chow & Shi (2014) examined that students' perceptions of online learning, especially flexibility and motivation, have an important influence on online learning. Besides, Paechter et al. (2010) suggested that the interaction between students and lecturers is an important factor in online student satisfaction. Other research states that the level of student satisfaction in online learning is influenced by the convenience of using the internet, where online discussions using certain media are very popular with students (Wei & Chou, 2020).
Because there are many important factors that can affect student satisfaction in online learning, this study accommodates satisfaction construct. Furthermore, this factor will be used as a basis for examining the relationship between perceptions of student readiness and satisfaction in the online learning process. Thus, the first hypothesis is as follows.
-
H1: Student perceptions of online learning have a positive effect on student satisfaction
The acceptance of technology in online learning can be assessed using the Technology Acceptance Model (TAM) which can explain the causal relationship between beliefs and behavior. This relationship is measured by the usefulness and convenience of a technology (Davis, 1985). The easier the technology is accessed, the higher the tendency for individuals to use it. This utility according to Fadare et al. (2016) has a direct impact on an individual's intention to use technology in the future.
There are several factors that represent an assessment of how useful educational technology in online learning that can influence the intention to use the technology in the future. The principle of the convenience of a technology can be considered easy if it does not require hard effort to use it (Davis, 1989). When the technology is considered easy, then the use of the technology is also in accordance with its function thus encouraging the intention to use it. It depends on the perceived ease of use of technology. If its use is considered easy, the tendency to use will be higher (Barhoumi, 2016). Thus, the second hypothesis is constructed as follows.
-
H2: Students' perceptions of online learning have a positive effect on the intention to use online learning
Students' perceptions of online learning vary widely depending on their attitudes towards using the internet and computers. Research conducted by Wei & Chou (2020) showed that students' attitudes towards technology greatly affected its usefulness in the future. The use of search engines on the website can affect the information obtained online.
Several studies have also been conducted to increase students' interest and abilities in online learning. Liaw & Huang (2013) reported that there are four important dimensions in online learning, such as online learning as an independent learning environment, learning effectiveness, multimedia learning, and being supported by lecturers. Wei & Chou (2020) found that online learning development can run well if there are flexibility, adaptability, comfort, and interaction. Encouraging students' selfmotivation to do online learning is also important (Tseng, 2020).
Furthermore, whether online learning can affect student performance from increasing the score of independent assignments, group assignments, midterm and final semester exams, Ke & Kwak (2013) revealed that several key components can affect student performance. Bertea (2005) further reported that the use of the internet by students can affect student performance in online learning. Yang & Lin (2010) also observed that when discussing online, students who have good internet connections will be more active in discussions. Wei & Chou (2020) have also examined the relationship between student perceptions of online learning, study habits, student achievement in online learning. From the research results, it is known that flexibility and self-motivation have a major effect on improving student understanding and test scores.
From some of the previous research, it can be understood that the perception of online learning can affect student performance. In the online learning process, comfort is needed to motivate students to be more active during its implementation. Therefore, this study focuses on finding out more about the relationship between perceptions of online learning, online learning performance, and learning comfort. Thus, the third hypothesis is constructed as follows.
-
H3: Students' perceptions of online learning have a positive effect on student performance and achievement
Research on the relationship between online learning satisfaction and student performance has been conducted in previous studies. Chitkushev et al. (2014) stated that students who get good grades tend to be taught by lecturers who are interested in paying attention to the course. In other words, students feel satisfied with what they get in class, when there is a supportive class atmosphere or an interesting lecturing. Then the scores obtained by these students tend to be quite high. The class atmosphere and lecturer competence have a positive influence on student performance which can be seen from the scores obtained by students (Benta et al., 2014).
In this case, the grades of individual assignments, groups, midterm exams and final exams are a benchmark for student performance. The class atmosphere as well as the lecturer competence and creativity are important factors that will impact student performance. Thus, the fourth hypothesis is as follows.
-
H4: Student satisfaction has a positive effect on student performance and achievement
There are several studies that have been conducted regarding online learning satisfaction on reuse intention. Liaw & Huang (2013) argued that there is a relationship between the satisfaction obtained when using online learning and intention to use in the future. Furthermore, Liaw et al. (2007) found that individual satisfaction with online learning systems can influence the intention to use online-based learning. In addition, there are many other factors that can indirectly influence the outcomes (Chang et al., 2011).
To investigate the effect of this satisfaction, the researcher used several factors to reflect an indication of the intention to use online learning which was influenced by student satisfaction. For example, the teaching style of lecturers, class content and structure, lecturer performance and discussion forums. It is expected that all these factors can clearly reflect the intention to use online learning. Thus, the fifth hypothesis is as follows.
-
H5: Student satisfaction has a positive effect on the intention to use online learning
Figure 1. Research Framework
Source: Processed Data, 2020
Research Method
The research method used in this survey is a quantitative method. Quantitative research is a study that is used to examine a specific sample. The sampling technique used was purposive sampling. The distribution of the questionnaire was carried out online with Google Form, by providing a link to the questionnaire to the target sample and then filling it out independently by the respondents. The sample is determined by the characteristics of the respondent, namely a. student of undergraduate majoring in taxation/fiscal, b. students who have taken online taxation/fiscal courses and c. minimum in the second semester. The demographics of respondents who have filled out the questionnaire can be seen in Appendix 1.
This research is a quantitative study and uses primary data obtained from surveys. The list of questions used consisted of 40 pieces arranged on a 5 Likert scale. This scale starts from 1 = Strongly Disagree to 5 = Strongly Agree. The higher the number shown, the more significant the impact of online learning perceptions will be. Then the 40 questions represent several indicators.
Perceptions of online learning can provide an overview of the conditions and perceptions of students related to the online learning process that is being carried out. Wei & Chou (2020) assumed that the development of online learning can run well not only with flexibility, adaptability, comfort, and interaction, but also must know the selfmotivation of students to do online learning. Therefore, the indicators used in this study are divided into 5 dimensions, namely accessibility, interactivity, adaptability, knowledge acquisition, and ease of loading (Appendix 2-Appendix 6).
Student satisfaction relates to the hopes or expectations of students for online learning have been fulfilled or not. The level of satisfaction can be influenced by several factors such as class structure, educational activities, curriculum, teacher competence, and facilities (Eichelberger & Ngo, 2018). Every factor in the teaching process plays an important part in influencing the level of student satisfaction. The questions in the questionnaire about this are listed in Appendix 7.
Learning performance and student achievement can be reflected on the tests scores obtained by students. Wei & Chou (2020) have also examined the relationship between student perceptions of online learning, study habits and student achievement in online learning. From the research results, it is known that flexibility and selfmotivation have a major effect on improving student understanding and tests scores. The questions in the questionnaire about this are in Appendix 8.
In this case, students as users of online learning services feel their hopes are fulfilled. Then there will be an intention to use the same or similar services in the future. Chow & Shi (2014) examined that student satisfaction in online learning has an effect on the tendency to use in the future. This is based on flexibility and motivation for students to be easier and increased due to the online system that helps students in the learning process. The questions in the questionnaire about this are listed in Appendix 9.
Hypothesis testing is executed by using Partial Least Square Structural Equation Modeling (PLS-SEM). PLS-SEM is SEM with a causal-predictive approach that emphasizes predictions in statistical estimation models which structure is designed to explain the causal relationship (Hair et al., 2012). PLS-SEM is also appropriate for research that tests predictive theoretical frameworks, has a complex model structure with many constructs, and a small population (Hair et al., 2019). In this study, the data were processed using the WarpPLS version 7.0 application, which involved resampling Bootstraping.
Result and Discussion
The first step is testing the reflective model which is carried out by looking at the loading factor value. Hair et al. (2012) indicated that average variance extracted (AVE) between 0.443 to 0.653 is a good scale. The loading value > 0.5 can still be tolerated.
-
Table 1. Indicator Loading
US IU SP POL
US1 |
0.848 |
US2 |
0.864 |
US3 |
0.818 |
US4 |
0.741 |
US5 |
0.685 |
IU1 |
0.873 |
IU2 |
0.907 |
IU3 |
0.933 |
SP1 |
0.607 |
SP2 |
0.675 |
SP3 |
0.852 |
SP4 |
0.856 |
ACC1 |
0.343 |
ACC2 |
0.307 |
ACC3 |
0.676 |
ACC4 |
0.647 |
INT1 |
0.571 |
INT2 |
0.646 |
INT3 |
0.424 |
INT4 |
0.582 |
INT5 |
0.623 |
INT6 |
0.585 |
ADP1 |
0.484 |
ADP2 |
0.401 |
ADP3 |
0.344 |
ADP4 |
0.391 |
KA1 |
0.575 |
KA2 |
0.646 |
KA3 |
0.599 |
KA4 |
0.615 |
KA5 |
0.505 |
EOL1 |
0.426 |
EOL2 |
0.495 |
EOL3 |
0.289 |
EOL4 |
0.532 |
Sources: Processed Data, 2020
(p-value <0,01)
Where: IU=Intention of Use, SP= Student Perform & Achievement, US= User Satisfaction, EOL=Ease of Loading, KA=Knowledge Acquisition, ADP=Adaptability, INT=Interactivity, ACC=Accessibility, POL= Perception of Online Learning
-
Table 2. Composite Reliability
US IU SP POL
Composite 0.894 0.931 0.839 0.891
Reliability
Sources: Processed Data, 2020
Table 1. shows all the loading values for each reflective indicator. Indicators that have a high loading factor indicate a high contribution relative to those with a low loading factor in explaining the constructs. The highest contributors for each construct are as follows: the item of US2 with a value of 0.864 for the latent construct of User Satisfaction (US); the item of IU3 with a value of 0.933 for the latent construct of Intention of Use (IU); the item of SP4 with a value of 0.856 for the latent construct Student Performance (SP); and the item of ACC3 with the highest value of 0.676 for the latent construct of Perception of Online Learning (POL).
Internal consistency is an indicator that functions to measure the reliability of a variable (Hair et al., 2019). The higher the value shown, the higher the reliability. Good scores range from 0.70 to 0.90. In exploratory research, the value ranges from 0.60 to 0.70 is still acceptable. Hair et al. (2019) revealed that if there is a value > 0.95 it indicates that the item is repeated. However, if there are no repeated items, then it is acceptable. Based on the test result, it can be concluded that the level of reliability is quite high. It is indicated by the composite reliability value of 0.894 for US; 0.931 for IU; 0.839 for SP; and 0.891 for POL. This shows that the accuracy, precision and consistency of a measuring instrument in making measurements is quite good (Table 2.).
Convergent Validity test is done by checking the Average Variance Extracted (AVE) value. According to Fornell & Larcker (1981) the minimum value for AVE is 0.5. Table 3. shows the correlation between the component score and the construct score. The value of 0.630 for US is considered to be sufficient; the value of 0.819 for IU is considered to be high; and the value of 0.571 for SP is considered to be sufficient. However, the value of 0.273 for POL is considered to be low.
This discriminant validity is tested by the criteria table from Fornell & Larcker (1981) which states that it is seen from the value of the square root of AVE on latent variables > the correlation value with other variables. Table 4. shows the summary of the discriminant validity, the higher the reflective value of the indicator, the better the correlation between the indicator and its latent construction. It is indicated that the value of 0.794 for US; 0.905 for IU; and 0.756 for SP are considered to be sufficient. However, the value of 0.523 for POL is considered to be a low correlation relative to the others.
This test is carried out so that there is no bias in the regression results (Hair et al., 2019). Collinearity problems will arise if VIF > 5. Based on the results of this test, it can be seen that all variable values are less than 5. Thus, there is no bias in the
-
Table 3. Convergent Validity
US IU SP POL
0.630 0.819 0.571 0.273
Table 4. Discriminant Validity
US |
IU |
SP |
POL | |
US |
0.794 |
0.317 |
0.417 |
0.602 |
IU |
0.317 |
0.905 |
0.185 |
0.465 |
SP |
0.417 |
0.185 |
0.756 |
0.448 |
POL |
0.602 |
0.465 |
0.448 |
0.523 |
Sources: Processed Data, 2020
regression results which are shown at the value of 1.643 for US; 1.282 for IU; 1.309 for SP; and 1.945 for POL (Table 5.).
The coefficient of determination is a value that shows the ability of the independent variable to represent the explanation of the dependent variable (Hair et al., 2012). Schuberth et al. (2018) stated that the standard value of this coefficient is 0.25; 0.50; and 0.75 which reflects weak, moderate, and substantial abilities. Based on test results, it can be concluded that the constructs of US, IU, and SP can explain the variation on the dependent variable about 38.40%; 21.6%, and 24.2% respectively.
This coefficient is a measurement used to assess the relevance of each latent variable (Kock, 2014), where the value of more than 0.1 is considered to be relevant. it can be seen that the coefficients for US, IU, and SP are considered to be relevant, which is shown at a value of more than 0.1, namely 0.387 for US, 0.229 for IU, and 0.256 for SP construct.
Hypothesis test is carried out by checking at the path coefficient and p-value of the coefficient path which is shown in Table 6. The path value of the coefficient is in the interval -1 to 1. Thus, the value closest to -1 or 1 has the strongest effect, whereas if the value closest to 0 has the weakest effect (Hair et al., 2019).
It can be assessed that the path coefficient value of POL>US is 0.623 with a p value <0.001, it means that POL has a positive and significant effect on US. Then the path coefficient value POL>IU is 0.439 with a p-value <0.01, which means that POL has a positive and significant effect on IU. The path coefficient value POL>SP is 0.347 and has a p-value <0.01 so it can be interpreted that POL has a positive and significant effect on SP. Furthermore, the path coefficient of US>SP has a value of 0.204 with a p-value of 0.01, so US has a positive and significant effect on IU. And the path coefficient between US>IU has a value of 0.056 with a p-value <0.239, which means that US has no influence on IU. Hypothesis testing can also be done by checking at the T-ratios and confidence
interval. The hypothesis is accepted if the T-ratios value>Critical T-ratios, and there is no value of 0 on the confidence interval. Based on Table 6., with a confidence level of 0.95, H5 is not supported.
Based on Table 7. it can also be seen that the relationship between POL>US, POL>IU, POL>SP, and US>SP which is indicated by the confidence interval is supported. However, the relationship of US>IU is unsupported.
The results of this study indicate that the perception of online learning has a
Table 5. VIF
US |
IU |
SP |
POL |
1.643 |
1.282 |
1.309 |
1.945 |
Table 6. Hypotheses Testing
US (H1) |
IU (H2) |
SP (H3) |
US (H4) |
US (H5) | |
POL |
0.623* |
0.439* |
0.347* | ||
IU |
0.056** | ||||
SP |
0.204* | ||||
POL |
Source: Processed Data, 2020
Where: * = P-value < 0,01
** = P-value > 0,239
positive effect on student satisfaction. This result is supported by the hypothesis testing above. These results can be interpreted that students are satisfied when this online learning is implemented. Satisfaction really depends on the manifestation of students' beliefs and expectations as users of this learning media. The expectation of online learning in the form of easy access and adaptability is an important part of influencing student satisfaction. Students who are able to use this learning media well, especially those who have the ability to surf the internet, will feel satisfied with online learning. Because all activities are carried out online and are very dependent on internet skills. This response is supported by the level of information accessibility in online learning that is easy for students to do. This can be seen in the question item ACC2 which asks about the providing of online resources and information, about 84 students (53.5%) respond agreement. Moreover, based on the respond to the question item ACC1, about the availability of various online information media as many as 98 students (62.4%) agree with this statement.
Then, the adaptability that students have also plays an important role in determining the level of student satisfaction. This is due to significant changes in the learning activities carried out by students. Because before Covid-19 hit, most students in Indonesia studied face-to-face or offline. Therefore, this rapid change influences students' level of adaptability. Students who are easy to adapt tend to be able to organize their learning activities independently, such as time and place to study. This adaptability affects student learning satisfaction. If students have difficulty to adapt, their beliefs and hopes in online learning will be difficult and not in accordance with student expectations. The level of student adaptability can be seen from self-efficacy regarding time management and student learning locations is more flexible and regular. This is supported by 52 students (33.1%) who agree with that conditions. Therefore, the
Table 7. T-ratios & Confidence Interval
Relationship |
Critical T ratios |
T-ratios |
Confidence Interval |
Hypothesis |
POL > US |
1.645 |
8.880 |
0.485 – 0.760 |
Supported |
POL > IU |
1.645 |
6.018 |
0.296 – 0.582 |
Supported |
POL > SP |
1.645 |
4.660 |
0.201 – 0.493 |
Supported |
US > SP |
1.645 |
2.655 |
0.053 – 0.355 |
Supported |
US > IU |
1.645 |
0.712 |
(0.099) – 0.212 |
Unsupported |
perception of online learning on student satisfaction is considered significant, supported by responses from respondents who agree with some of the statements in the questionnaire.
Furthermore, this study is in accordance with the research of Eichelberger & Ngo (2018) that lecturers’ competence and class structure are important factors in giving confidence to students to feel satisfied with the learning material obtained. It is proven in question item US2 which states that 75 students (47.8%) agree to be satisfied with the content received and the class structure of online learning. Besides, the question item US3 about lecturer performance, 82 students (52.2%) are satisfied with lecturer performance in online learning.
The results of this study indicate that online learning perceptions of students has a positive impact on intention to use in the future. This is supported by the hypothesis testing result. Perceptions of online learning have an influence on the intention to use which can be assessed from the ease and usefulness of the technology. The easier it is to use the technology, in this case, online learning media, the higher the intention to use. The ease of accessing, interacting, adapting and obtaining knowledge is the main point in this case. These results are in accordance with research conducted by Fadare et al. (2016) which state that convenience and usability are the main factors in increasing the intention to use the technology. This is supported by question item ACC1, 2, and 4 regarding the accessibility of online learning. About 98 students (62.4%) agree that online learning provides many learning media. Moreover, 84 students (53.5%) agree that online learning provides various online resources and information. Then 58 students (36.9%) agree that online learning makes it easy to share and exchange the learning materials and information. From these results it can be seen that the ease can be felt by students when using online learning. Then in question item ADP3, it was found that 65 students (41.4%) agree that online learning allows them to repeat learning material many times as needed. Furthermore, in ADP4, 44 students (28%) strongly agree that this learning can overcome the constraints of learning time and place.
Students also appreciate the usefulness and convenience, especially in terms of accessibility, adaptability, and knowledge acquisition. It is hoped that this will lead to an intention to use online learning in the future. Thus, the usefulness and convenience can still be felt and help students to get and achieve their target.
From the research results it can be interpreted that the perception of online learning has a positive effect on student performance and is supported by the hypothesis testing above. In this case, adaptability and accessibility as well as student test scores are important points in assessing the effect of perceptions on student learning performance. Due to the adaptability of students, starting from managing the time and place of study for lectures in general and independent study. Students who are able to manage this tend to have good performance and test scores. Then the ease of access to information and use of the internet also affects student learning performance. Students who are accustomed to using the internet and searching for information on the internet tend to have good test scores.
These results are consistent with research conducted by Bertea (2009) that good internet use will affect performance in online learning. Student accessibility to internet
access tends to be high, which means that students are quite proficient in using the internet. This indirectly improves student performance in online learning. Then these results are also in accordance with the research conducted by Wei & Chou (2020) that flexibility and self-motivation have a big effect on increasing student understanding and test scores. This was supported by 67 students (42.7%) agreeing to be able to decide the location freely in online learning. About 50 students (31.8%) felt that the online learning environment was effective enough to reduce student learning load. Then the test scores were also asked in the questionnaire to respondents to reflect the state of student learning performance when doing online learning. From the results of the study, it was found that 50 students (31.8%) agreed to be satisfied with their mid test scores and 41 students (26.1%) agreed to be satisfied with their final test scores. Further, most of the students also felt quite satisfied with the scores obtained on individual and group assignments.
Because perception of online learning has a positive influence on student performance, it is hoped that online learning can be considered in the future with some adjustments. It will be better if the continuity of online learning is also supported by better tools, infrastructures and flexibility. It becomes a burden if the signal quality is not good when students are in online exams. This should be a concern that needs to be addressed in the future.
The results show that student satisfaction has a positive effect on student performance and achievement, the hypothesis is supported. In this case, the interactivity and the ease of obtaining knowledge are the main parts that can affect student learning performance. The interaction between students and lecturers as facilitators in online learning has a significant effect. The ease of interaction offered in online learning via the internet and its supporting media can affect student learning performance. Paechter et al. (2010) suggest that the interaction between students and lecturers is an important factor in online student satisfaction. This is in accordance with research conducted by Chitkusev et al (2014) that an attractive lecturer will motivate the students to learn and increase the grades obtained by students. It was proven that 82 students (52.2%) agreed to be satisfied with the lecturer's performance during online learning. And 75 students (47.8%) agreed to be satisfied with the learning content provided and the class structure. Then most respondents quite agree 39%-51% to be satisfied with the scores of assignments, midterm exams, final semester exams, and group assignments. Then also 65 students (41.4%) strongly agree that online learning makes it easier for them to review online learning materials.
However, it is necessary to understand that too often schedule changes can disturb the stress management designed by each student. About 54 respondents (34.4%) do not agree that the online learning environment is not too stressful. This has an indirect effect on student performance and achievement. Therefore, further direction is needed as an effort to improve students’ ease of loading.
The results show that student satisfaction does not have an impact on intention to use online learning in the future. In this case, the level of student satisfaction should be directly proportional to the intention to use which is influenced by the teaching style of lecturers, class content and structure, lecturer performance, and discussion forums
(Liaw & Huang, 2013). However, the results of hypothesis testing find that, in this study, the level of satisfaction did not support the intention to use. This occurs due to the very small effect of student satisfaction on the intention to use this online method in the future. Based on the survey, students' preferences on face-to-face learning methods are better compared to online learning.
In the questionnaire distributed to the respondents, the researcher inserted several qualitative questions about the problems regarding experiences during online learning. The main problem regarding online learning according to the respondents was the difficulty of getting internet signals and performance of devices that could support the learning process. Besides, about the exam time-duration, some respondents argued that they should be given an additional time because there were some unexpected problems due to signals and devices that did not support the exam process.
Then, about the comparison of online and face-to-face learning, the majority of respondents preferred a face-to-face learning because they perceived that with face-to-face learning, it will make it easier for them to communicate and interact with lecturer in the class. In addition, the ease of communication among students can be felt better during face-to-face learning. The majority of students strongly agreed that face-to-face learning provided more motivation for students to learn better.
Questions to further enrich the findings, such as the problems/obstacles in doing online learning; how the respondent follows up to solve the problem; and what things need to be improved in the implementation of online learning. We describe several answers from some respondents as well as provide implications for relevant stakeholders.
First, the problems that are often faced when online learning are dominated by the technical problems, such as a weak and unstable internet network, lags or errors on the devices used and electrical blackout. In addition, communication and interaction tends to be limited, not as dynamic and flexible as when the learning is conducted in the traditional classroom. Besides, a good class coordination is also very necessary so that all lecturers’ materials are well documented and can be accessed by students.
For subjects that involve calculation, such as taxes calculation, some students admit that they have difficulty in understanding the learning material during online classes. They argue that if the material is taught in the class, the calculation of taxes will be clearer and easier to understand. To reduce this difficulty, in this case, lecturers are strongly advised to use a virtual whiteboard, create a teaching video recording accompanied by a complete simulation of calculations, provide several video links relevant to the material, and provide more space and time for questions and answers regarding the simulations of the calculations to confirm students understanding. The students are also highly encouraged to be active in confirming their understanding about the difficult materials as well as in discussing and participating in the discussion. Providing of online assistance classes with lecturer assistants can also be an input to improve student understanding of difficult learning material.
In addition, there is also an input from students who state that online learnings are quite effective if the courses contain theories, concepts, and procedures; however online learnings are less effective when it is applied to the subjects that are very high
intensity with calculations, such as taxes calculations. This input can also be valuable for faculty or university managers in the future, when the pandemic is over, learning methods can be conducted in a hybrid one, the combination of online and offline method.
Secondly, to solve the problems regarding the implementation of online class, various alternative ways are taken by students to overcome the above obstacles, such as finding a place that is conducive to online learning, a stable signal, switching to use a smart phone and using personal cellular data if there is a problem with Wi-Fi. In addition, students who find it difficult to understand the material, take the initiative to conduct online discussion with the other students outside the lecturers’ online class, take the initiative to set a joint schedule for online group discussion, share learning materials from various sources, or replay lecturers’ video recordings.
Third, overall, students perceived that the performance of lecturers and the implementation of online learning are quite good. However, there are some points that need to be maintained and improved. For example, giving assignments and examinations that have considered the quantity, quality, level of difficulty and processing time; providing feedback on students’ assignments and exams; using online whiteboards, especially for material containing calculations such as taxes calculations; creating more conducive conditions for online classrooms in a more communicative and interactive way; achieving optimal use of e-learning media, especially for documenting all learning materials, collecting assignments, examinations; and not changing online learning schedules too often. In addition, the challenges for lecturers are about how to provide proper feedbacks to students on assignments or exam results to confirm students’ understanding and how to measure students' performance precisely.
Conclusion
This study aims to investigate the relationship between perceptions of online learning on student satisfaction which has an impact on the intention to use online learning methods and student performance, especially in taxation/fiscal subjects. The results of the tests conducted prove that students' perceptions of online learning have a positive effect on student satisfaction, the intention to use online learning, and student performance and achievement; student satisfaction has a positive effect on student performance and achievement. However, student satisfaction has no effect on the intention to use online learning in the future. In other words, when the Covid-19 pandemic is over, students prefer face-to-face learning rather than online, especially for subjects with high intensity of calculations. Face-to-face learnings allow more flexible communication and interaction for lecturers and students thus students’ understanding can be confirmed quickly, effectively, and efficiently.
From the results of this study it can also be understood how the condition of students, both mental readiness and supporting devices at the time of implementing online learning. The faculties, universities, and external parties can facilitate students in terms of the internet and various learning support media. The facility is one of the most important factors in successful online learning. If this factor can be fulfilled and the
functions are optimized, it is hoped that student satisfaction with online learning can increase as well as student performance.
The results of this study can be used as input, reference, and consideration for lecturers, faculties and universities, as well as the government as a regulator. For lecturers in general, the results of this study can provide information regarding the implementation of online learning, especially during the Covid-19 pandemic. Lecturers are encouraged to continue working in developing effective and efficient teaching methods, especially in online learning. For the faculty and university as facilitators, the results of this paper can support them in making decisions whether to continue learning online or hybrid (the combination of online and offline method) in the future, especially when the Covid-19 pandemic is over, by also adjusting the curriculum. Finally, the government as the regulator can use the results of this research to evaluate the implementation of online learning and provide real support for the needs of students, lecturers, faculties and universities to make online learning successful.
This study has several limitations. First, this study only focuses on the responses of students who are currently taking taxation courses. Further research may include responses from broader scope of students as well as the lecturers. Second, the number of respondents in this research tends to be small. Future studies may include a larger number of respondents. Finally, the measurement of student performance is based on perception. Further research can accommodate matched surveys where performance measurement using both perception data and actual study results.
References
Barhoumi, C. (2016). User acceptance of the e-information service as information resource: A new extension of the technology acceptance model. New Library World, 117(9–10), 626–643. https://doi.org/10.1108/NLW-06-2016-0045
Benta, D., Bologa, G., & Dzitac, I. (2014). E-learning platforms in higher education. Case
study. Procedia Computer Science, 31, 1170–1176.
https://doi.org/10.1016/j.procs.2014.05.373
Bertea, P. (2005). Measuring Students’ Attitude Towards E-Learning. a Case Study. The 5th International Scientific Conference ELearning and Software for Education, 8. http://citeseerx.ist.psu.edu/viewdoc/download?doi=10.1.1.623.6294&rep=rep1 &type=pdf
Chang, Y.-J., Chen, C.-H., Huang, W.-T., & Huang, W.-S. (2011). Investigating students’ perceived satisfaction, behavioral intention, and effectiveness of English learning using augmented reality. 2011 IEEE International Conference on Multimedia and Expo, 1–6. https://doi.org/10.1109/ICME.2011.6012177
Chitkushev, L., Vodenska, I., & Zlateva, T. (2014). Digital Learning Impact Factors:
Student Satisfaction and Performance in Online Courses. International Journal of Information and Education Technology, 4(4), 356–359.
https://doi.org/10.7763/ijiet.2014.v4.429
Chow, W. S., & Shi, S. (2014). Investigating Students’ Satisfaction and Continuance Intention toward E-learning: An Extension of the Expectation – Confirmation Model. Procedia - Social and Behavioral Sciences, 141, 1145–1149. https://doi.org/10.1016/j.sbspro.2014.05.193
Davis, F D. (1985). A technology acceptance model for empirically testing new end-user information systems: Theory and results. Management, Ph.D.(May), 291. https://doi.org/oclc/56932490
Davis, Fred D. (1989). Perceived usefulness, perceived ease of use, and user acceptance of information technology. MIS Quarterly: Management Information Systems, 13(3), 319–339. https://doi.org/10.2307/249008
Eichelberger, A., & Ngo, H. T. P. (2018). College Students’ Perception of an Online Course in Special Education. International Journal for Educational Media and Technology, 12(2), 11–19.
https://www.researchgate.net/publication/335777401
Fadare, O. A., Ibrahim, M. B., & Edogbanya, A. (2016). A survey on perceived risk and intention of adopting internet banking. Journal of Internet Banking and Commerce, 21(1), 1–21.
Fornell, C., & Larcker, D. F. (1981). Evaluating Structural Equation Models with Unobservable Variables and Measurement Error. Journal of Marketing Research, 18(1), 39. https://doi.org/10.2307/3151312
Hair, Joe F., Sarstedt, M., Ringle, C. M., & Mena, J. A. (2012). An assessment of the use of partial least squares structural equation modeling in marketing research. Journal of the Academy of Marketing Science, 40(3), 414–433.
https://doi.org/10.1007/s11747-011-0261-6
Hair, Joseph F., Risher, J. J., Sarstedt, M., & Ringle, C. M. (2019). When to use and how to report the results of PLS-SEM. European Business Review, 31(1), 2–24. https://doi.org/10.1108/EBR-11-2018-0203
Ke, F., & Kwak, D. (2013). Online learning across ethnicity and age: A study on learning interaction participation, perception, and learning satisfaction. Computers & Education, 61, 43–51. https://doi.org/10.1016/j.compedu.2012.09.003
Keengwe, J., & Georgina, D. (2012). The digital course training workshop for online learning and teaching. Education and Information Technologies, 17(4), 365–379. https://doi.org/10.1007/s10639-011-9164-x
Kock, N. (2014). Advanced Mediating Effects Tests, Multi-Group Analyses, and Measurement Model Assessments in PLS-Based SEM. International Journal of ECollaboration, 10(1), 1–13. https://doi.org/10.4018/ijec.2014010101
Lee, J.-W., & Mendlinger, S. (2011). Perceived Self-Efficacy and Its Effect on Online Learning Acceptance and Student Satisfaction. Journal of Service Science and Management, 04(03), 243–252. https://doi.org/10.4236/jssm.2011.43029
Lee, J. W. (2010). Online support service quality, online learning acceptance, and student satisfaction. Internet and Higher Education, 13(4), 277–283.
https://doi.org/10.1016/j.iheduc.2010.08.002
Liaw, S.-S., & Huang, H.-M. (2013). Perceived satisfaction, perceived usefulness and interactive learning environments as predictors to self-regulation in e-learning environments. Computers & Education, 60(1), 14–24.
https://doi.org/10.1016/j.compedu.2012.07.015
Liaw, S.-S., Huang, H.-M., & Chen, G.-D. (2007). Surveying instructor and learner attitudes toward e-learning. Computers & Education, 49(4), 1066–1080. https://doi.org/10.1016/j.compedu.2006.01.001
Lust, G., Elen, J., & Clarebout, G. (2012). Online Measurement Perspectives for Students’ Strategy Use: Tool Use within a Content Management System. Education Research International, 2012, 1–11. https://doi.org/10.1155/2012/702315
Paechter, M., Maier, B., & Macher, D. (2010). Students’ expectations of, and experiences in e-learning: Their relation to learning achievements and course satisfaction. Computers & Education, 54(1), 222–229.
https://doi.org/10.1016/j.compedu.2009.08.005
Schuberth, F., Henseler, J., & Dijkstra, T. K. (2018). Partial least squares path modeling using ordinal categorical indicators. Quality and Quantity, 52(1), 9–35.
https://doi.org/10.1007/s11135-016-0401-7
Tseng, H. (2020). An exploratory study of students’ perceptions of learning management system utilisation and learning community. Research in Learning Technology, 28(1063519), 1–18. https://doi.org/10.25304/rlt.v28.2423
Wei, H. C., & Chou, C. (2020). Online learning performance and satisfaction: do perceptions and readiness matter? Distance Education, 41(1), 48–69. https://doi.org/10.1080/01587919.2020.1724768
Yang, Y., & Lin, N. C. (2010). Internet perceptions, online participation and language learning in Moodle forums: A case study on nursing students in Taiwan. Procedia - Social and Behavioral Sciences, 2(2), 2647–2651.
https://doi.org/10.1016/j.sbspro.2010.03.388
Appendix 1. The Demographics of Respondents | ||
Gender | ||
Male Female Total |
52 105 157 | |
Age | ||
17-19 |
60 | |
20-22 |
96 | |
23-25 |
1 | |
Total |
157 | |
Level |
of | |
Education | ||
Undergraduate |
157 | |
Majoring | ||
Fiscal Administrative Science |
157 | |
Subject | ||
Introductory of Taxation |
10 | |
Corporate Income Tax |
9 | |
Introduction to Tax Law |
2 | |
Value Added Tax and Sales Tax on Luxury Goods |
3 | |
Withholding and Tax Collection System |
4 | |
Local taxes and Levies |
22 | |
Tax Investigation |
12 | |
Tax Management |
41 | |
Personal Income Tax |
51 | |
Tax Accounting |
2 | |
International Tax |
1 | |
Total |
157 | |
Semester | ||
Semester 3 |
64 | |
Semester 5 |
38 | |
Semester 7 |
54 | |
Semester 9 |
1 | |
Total |
157 | |
The Use |
of | |
Online Learning | ||
<6 months |
104 | |
6 months - <1 year |
52 | |
1 - 2 year |
1 | |
Total |
157 |
No. Code
Appendix 2. Accessibility Questionnaire
Question Accessibility
1 |
ACC1 |
Online learning provides various online learning media |
2 |
ACC2 |
Online learning provides a variety of online resources |
3 |
ACC3 |
Online learning allows students to get back and get more |
resources | ||
4 |
ACC4 |
Online learning allows students to share and exchange knowledge resources |
Source: Wei & Chou (2020) | ||
Appendix 3. Interactivity Questionnaire | ||
No. |
Code |
Question Interactivity |
1 |
INT1 |
Online learning allows students to interact directly with other students |
2 |
INT2 |
Online learning can encourage interaction between lecturers and students |
3 |
INT3 |
Online learning can shorten the distance between lecturers and students |
4 |
INT4 |
Online learning allows students to meet more classmates or peers with the same interests or habits |
5 |
INT5 |
Online learning provides students with ample discussion opportunities |
6 |
INT6 |
Online learning provides students with a convenient tool to communicate with other students |
Source: Wei & Chou (2020) | |
Appendix 4. Adaptability | |
Question | |
No. Code |
— |
Adaptability |
1 |
ADP1 |
Online learning allows students to decide the best time to study |
2 |
ADP2 |
Online learning allows students to decide the best location to study |
3 |
ADP3 |
Online learning allows students to repeat learning material repeatedly |
4 |
ADP4 |
Online learning can overcome the constraints of learning time and place |
Source: Wei & Chou (2020) | ||
Appendix 5. Knowledge Acquisition Questionnaire | ||
No. |
Code |
Question |
Knowledge Acquisition | ||
1 |
KA1 |
Online learning can broaden the general knowledge base of students |
2 |
KA2 |
Online learning allows students to learn more about the knowledge that students are interested in |
3 |
KA3 |
Online learning can expand students' academic knowledge capacity |
4 |
KA4 |
Online learning is an effective learning style |
5 |
KA5 |
Online learning allows abstract ideas or abstract concepts to be presented correctly |
Source: Wei & Chou (2020) |
Appendix 6. Ease of Loading Questionnaire | |
No. |
Question Code Ease of Loading |
1 |
EOL1 Online learning environments take the pressure off of pursuing class schedules |
2 3 |
EOL2 The online learning environment is less stressful EOL3 Online learning environments place less stress on exams and assessments |
4 |
EOL4 Online learning environment can effectively reduce student learning load |
Source: Wei & Chou (2020)
Appendix 7. Student Satisfaction Questionnaire | |
No. |
Question Kode Student Satisfaction |
1 2 3 4 5 |
US1 Students are satisfied with the teaching style of the lecturer US2 Students are satisfied with the learning content and class structure US3 Students are satisfied with the lecturer's performance US4 Students are satisfied using online discussion forums US5 Overall students are satisfied learning online |
Source: Wei & Chou (2020)
Appendix 8. Student Perform Questionnaire | |
No. |
Question Code Student Performance |
1 2 3 4 |
SP1 Students are satisfied with the Final Term Exam Score SP2 Students are satisfied with the Mid-Term Exam score SP3 Students are satisfied with the individual assignments SP4 Students are satisfied with the group project |
Source: Wei & Chou (2020)
Appendix 9. Intention of Use Questionnaire | |
No. |
Code Question o e Intention of Use |
1 2 |
IU1 Students intend to reuse online learning in the future IU2 Students will always use Online learning in the future |
3 |
Students think that they will use online learning regularly in the IU3 future |
Source: Chow & Shi (2014)
Jurnal Ilmiah Akuntansi dan Bisnis, 2021 | 19
Discussion and feedback