Analysis of Macroeconomic Variables on the Existence of Inflation in Indonesia Using the Vector Error Correction Model Approach
on
pISSN : 2301 – 8968
JEKT ♦ 17 [1] : 9-35 eISSN : 2303 – 0186
Analysis of Macroeconomic Variables on the Existence of Inflation in Indonesia
Using the Vector Error Correction Model Approach
ABSTRACT
This research investigates the impact of macroeconomic variables, namely money supply, exchange rate, interest rate, and the joint stock index, on inflation in Indonesia. Employing the Vector Error Correction Model (VECM) dynamic model approach, the study reveals a long-term relationship among each variable (Inflation Rate, Jakarta Composite Index, Interest Rate, Exchange Rate, and Money Supply). Granger Causality Test results indicate a unidirectional relationship of interest rate and money supply variables to inflation, interest rate to Jakarta Composite Index, and money supply to the exchange rate. Conversely, there is a bidirectional relationship between exchange rate and inflation variables. In the short term, Interest Rate significantly and positively influences inflation, whereas, in the long term, it exhibits a negative and insignificant effect. Money supply, in the long run, significantly and positively affects the inflation rate. This study stands out in the macroeconomic literature due to its distinctive choice of variables and the dynamic model employed.
Keywords: Macroeconomic Variables, Vector Error Correction Model
JEL Classification: B22, P24
INTRODUCTION
A nation's economic well-being is deemed sound and robust when its macroeconomic factors align with anticipated trends. This stability is evident through several indicators: a consistently low and diminishing inflation rate, a reduction in the money supply, reduced volatility in the foreign exchange rate with a strengthening or appreciation against other currencies, a declining benchmark interest rate, and a relatively stable performance of the stock market index, such as the Jakarta
Composite Index on the stock exchange. Conversely, the presence of contrasting conditions may signal an unfavorable economic state within a country (Adisetiawan, 2014).
The inflation rate stands as a pivotal macroeconomic indicator that garners significant attention across various economies. Virtually all developing nations exhibit a keen interest in monitoring and addressing inflationary conditions (Ahmed, Chen, Kumpamool, & Nguyen, 2023; Salisu, Isah, Oyewole, O, & Akanni, 2017; Hendajany, 2015). When
inflation displays an inclination toward escalation, it exerts substantial influence on the surge in commodity prices. Concurrently, an upswing in the price level, unless accompanied by a corresponding increase in individuals' income, can precipitate a decline in their purchasing power.
In the realm of the real sector, a diminishing purchasing power represents an adverse signal for industrial operations. In such circumstances, production finds limited absorption within the market, thereby causing a consequential uptick in a company's expenditures, potentially resulting in workforce downsizing. It becomes incumbent upon the government, both in the fiscal and monetary domains, to uphold price stability encompassing goods and services within the economic landscape. Moreover, the government bears the responsibility of safeguarding exchange rate stability (Yıldırım, 2017), including the valuation of the rupiah relative to foreign currencies. Notably, fluctuations in exchange rates carry implications for the genesis of inflation within a nation. A substantial
depreciation or weakening of the exchange rate signifies a nation's diminished competitive standing in the international market. Indonesia, operating under a free-floating exchange rate system, signifies that the value of our national currency, the rupiah, is profoundly contingent upon the dynamics of demand and supply in the financial marketplace. Consequently, it becomes imperative to maintain an ample foreign exchange reserve to preempt any potential depreciation of the rupiah.
Interest rates represent the cost of capital and exert significant influence over the oscillations in inflation and the volatility of exchange rates (Desvina & Lubis, 2019). Heightened interest rates also leave an imprint on the investment portfolios of banks, particularly affecting (Andiantyo et al., 2018). This line of inquiry finds corroboration in the work of Nisa & Sukmana (2017), who assert that an upsurge in interest rates tends to depress the Jakarta Composite Index (JCI/IHSG) within the capital market, consequently leading to a potential contraction in the demand for equities. Notably, the ebb and flow of inflation and
interest rates can precipitate reverberations across international landscapes, influencing the depreciation of foreign exchange rates. However, it is pertinent to underscore that inflation need not invariably be perceived as an ominous specter for the economy. When inflation rates remain subject to effective control, they possess the potential to invigorate and galvanize entrepreneurial endeavors, thereby fostering a climate conducive to heightened economic growth.
The money supply constitutes a macroeconomic variable akin to the circulatory system of an organism, necessitating vigilant regulation. Monetary policy is entrusted with the crucial role of preserving its equilibrium within the economy, particularly in its capacity to shape price levels and activities within the tangible sector (Natsir, 2018). An expansion of the money supply within the economy carries the potential to trigger uncontrollable inflation rates(Ishaq & Mohsin, 2015). Consequently, the central bank, through its monetary policy, endeavors to elevate its reference interest rate as a measure to
curtail the money supply within the economy.
The capital market serves as a venue for the exchange of long-term capital, wherein companies issue securities to represent demand, and investors represent the supply side of this market dynamic (Widoatmodjo, 2015). Within the global economy, the capital market assumes a significant role, primarily functioning as an avenue for investors to build their investment portfolios, encompassing the trading of both stocks and bonds. The overarching objective in this context is the attainment of returns, underscoring the intention for investments to endure over an extended period, characterized by robustness and equilibrium (Widoatmodjo, 2015)
The Indonesian stock exchange has undergone significant growth, emerging as a pivotal component of the Indonesian economy. It fulfills multiple functions, serving as a platform for fundraising, facilitating investor activities in the trading of shares and bond issuances, and also operating as a barometer reflecting the stability of macroeconomic conditions within the nation (Beik & Fatmawati,
2014). Inflation has the potential to exert influence on the fluctuations in stock prices. An increase in inflationary pressures does not bode well for investors, as indicated by the study conducted by Rusbariand & Herawati (2012) which asserts that inflation correlates negatively with the Jakarta Composite Index (JCI). Conversely, a different research endeavor by Krisna & Wirawati (2013) reveals a positive association between inflation and JCI, while another study by Astuti, Lapian, & Rate (2016) posits that inflation holds no significant impact on the JCI.
Investors are acutely aware of the inherent risks and rewards associated with their participation in financial markets. It is imperative for an investor to possess a comprehensive understanding of the financial sector's dynamics. The movements and fluctuations observed in stock prices within the stock exchange can be markedly influenced by macroeconomic variables. As underscored by Antonakakis, N., Gupta, R. & Tiwari, (2017)), stock prices hold a pivotal status as leading indicators of economic activity, underscoring the critical significance of
identifying the factors underpinning market behavior. Furthermore, it is important to note that stock prices are subject to the influence of both financial variables and macroeconomic factors (Wang, Huang, & Zhang, 2023).
According to Tandelilin (2010), the fluctuation witnessed within the capital market are inherently linked to fluctuations in a myriad of macroeconomic parameters. Tangjitprom (2012) delineates these macroeconomic variables into four distinct categories. The first category encapsulates facets of overall economic growth, encompassing metrics such as employment levels and indices of industrial production. The second category encompasses variables related to interest rates and the monetary policy apparatus, serving as instrumental factors in regulating the equilibrium between money supply and demand. The third category delves into aspects of the price level, encompassing both the overarching general price level and the inflation rate. Lastly, the fourth category encompasses variables intertwined with international dynamics, such as foreign
direct investment, exchange rates, and the global financial market's performance.
In consonance with the paradigm of economic globalization, it is noteworthy that the stock market is no longer solely shaped by the contours of domestic macroeconomic variables but is instead markedly susceptible to international economic perturbations and events. In the context of globalization, a nation's interconnectedness with the global community undergoes a substantial expansion. The United States, as an exemplar of an advanced nation boasting the world's largest capital market industry, assumes a pivotal role as a barometer for assessing the evolution and performance of other stock exchanges worldwide (Haryogo, 2013).
In light of the empirical evidence and the divergent outcomes reported in preceding studies pertaining to the discourse on macroeconomic variables, it is noteworthy that disparate and incongruous findings have been recurrently documented. For instance, Janah & Pujiati (2018) underscores this recurrent theme of discordant results. Similarly, Nugroho (2008) research
contributes to the complexity of this narrative by illuminating a discernible causal linkage between inflation and exchange rate variables. Furthermore, Nugroho's study underscores the influential role played by the BI-rate benchmark interest rate in shaping inflation dynamics, both in the immediate term and over extended horizons.
Research conducted by Luwihadi & Arka (2017) revealed a statistically significant negative relationship between inflation and both money supply and interest rates. However, the study found that money supply does not exert a significant influence on inflation, and exchange rates do not have a discernible impact on inflation. In a related study by Nofiatin (2013), evidence of cointegration was observed among inflation, interest rates, and exchange rates. Additionally, causality was detected between inflation and interest rates, as well as between money supply and interest rates.
Building upon the backdrop of frequently divergent and incongruous research outcomes, this study endeavors to scrutinize and comprehensively assess the impact of money supply variables,
exchange rates, interest rates, and the Indonesian joint stock index on the dynamics of inflation, employing the Vector Error Correction Model (VECM) as the analytical framework.
LITERATURE REVIEW
Inflation emerges as a consequence of multifaceted forces originating from both the supply and demand dimensions, in addition to inflationary expectations. The origins of cost-push inflation can be ascribed to several factors, including the depreciation of exchange rates, the influence of inflation abroad, particularly in nations engaged in trade partnerships, escalations in administered commodity prices, and negative supply shocks stemming from natural calamities and disruptions in distribution channels (Wildauer, Kohler, Aboobaker, & Guschanski, 2023). Demand-pull inflation, in essence, is precipitated by a scenario wherein the demand for goods and services surpasses their supply. In macroeconomic terms, this scenario is characterized by the actual output outstripping the potential output, or equivalently, the aggregate demand surpassing the economic capacity.
Concurrently, the factor of inflation expectations is underpinned by the conduct of the public and economic agents who factor the anticipated inflation rate into their decision-making processes concerning economic activities. It is noteworthy that inflation expectations may assume either an adaptive or forward-looking orientation.
The dynamics of inflation within an economy necessitate continual vigilance and supervision. This imperative arises from the proclivity of inflationary tendencies to induce price escalation, thereby unsettling the equilibrium between supply and demand within society. In essence, inflation signifies not only an apparent surge in prices but also, at its core, a depreciation of a given currency concerning the relative valuation of goods and services. As elucidated by Mankiw (2007), inflation's genesis is not confined solely to heightened demand; it can also be engendered by disruptions in distribution channels, natural calamities, and escalating production costs.
When the prices of goods and services experience an upward trajectory,
the concurrent consequence is a decline in the purchasing power of the currency. This downturn in purchasing power carries ramifications for both the broader economy and corporate profitability. Notably, it engenders a diminution in the competitiveness of stock price movements, as explained by (Prastowo, 2008).
Exchange rates, as explained by Mishkin (2010), represent the valuation of one currency in relation to another. These rates are bifurcated into two categories: nominal exchange rates and real exchange rates. The nominal exchange rate pertains to the price of a nation's currency concerning foreign counterparts, while the real exchange rate is derived by dividing the nominal exchange rate by the relative price levels within domestic and foreign markets (involving trading partner nations). The real exchange rate serves as a benchmark for assessing a country's competitive standing vis-à-vis other nations.
The fluctuations in exchange rates, involving the IDR/USD pairing, as highlighted by Tanuwidjaya (2008), exert discernible impacts on stock prices, both
at the individual level and within the JCI context. Fahmi (2012) posits that an appreciating exchange rate has a positive influence on overall stock prices over the short term. Furthermore, an increase in the domestic currency's value (appreciation) serves as a favorable signal for economic activity. Conversely, a depreciation of the domestic currency is indicative of adverse conditions.
Money supply denotes the aggregate quantity of currency universally accepted for transactions involving the acquisition of goods or services, as well as the settlement of debts. It encompasses the total volume of all monetary forms within an economy, encompassing various forms of currency and deposit money, collectively referred to as the money supply (Hasyim, 2017). This concept of money in circulation or money supply assumes dual connotations, namely a narrow interpretation and a broader interpretation (Amalia, Wijaya, Darma, Maria, & Lestari, 2020).
Primarily, within the narrow definition, money in circulation encompasses currency physically in
circulation, along with demand deposits held by individuals, corporations, and governmental entities. In contrast, the broader characterization of money in circulation encompasses three components: (1) the physical currency in circulation, (2) demand deposits, and (3) quasi money. Quasi money refers to monetary assets held by the domestic private sector, encompassing time deposits, savings accounts, and foreign currency deposits.
In an economic scenario characterized by an excess of money in circulation, the currency's value diminishes, thereby instigating a reduction in purchasing power due to the ensuing escalation in prices of goods and services. Consequently, this circumstance results in a surplus of goods and services within the market, leading to an imbalance where supply exceeds demand. The repercussions of this imbalance manifest in the form of diminished corporate profits, stemming from the challenges companies face in maintaining profitability under such conditions. A reduction in corporate performance, in
turn, exerts downward pressure on the company's stock price.
An interest rate, as stated by (Dornbusch, Fischer, & Starz, 2008; Easterly & Schmidt-Hebbel, 1993; Hamburger & Zwick, 1981), denotes the cost associated with money, representing the rate of compensation for borrowing or investment endeavors, typically conveyed as an annual percentage figure. Interest rates play a pivotal role in guiding the decisions of investors and individuals, influencing their choices between depositing funds in a bank, investing, or engaging in expenditure within the real sector. This role underscores that interest rates also function as temporal prices, pertaining to both the present and the future, exerting an impact on economic activities geared towards profit generation.
Furthermore, interest rates exert a profound influence on corporate profitability, given that interest represents a financial cost. Consequently, a heightened interest rate corresponds to a diminished profit margin for companies.
The presence of interest rates within the economic landscape holds the
potential to exert a discernible impact on stock prices, particularly common stocks. In instances of rising interest rates, business operators and investors often incline towards depositing their funds in financial institutions, as the alluringly competitive interest rates render such a choice enticing. Consequently, these economic agents are compelled to engage in a comprehensive assessment, weighing the prospective gains from real-sector investments against the returns offered by bank deposit interest rates.
As stated by Fred & F.Eugene (1994) interest rates wield significant sway over stock prices. Elevated interest rates contribute to a sluggish economic environment, leading to an escalation in the cost of capital, which in turn constricts corporate profitability. This confluence of factors prompts investors to divest their stock holdings and redirect their capital towards the bond market.
Interest rate fluctuation heightened the appeal in instances of an upward trajectory in interest rates. This circumstance renders savings, deposits, and bonds more alluring, consequently inducing capital market investors to
reconfigure their equity portfolios. An escalation in selling activity coupled with subdued demand engenders a decrement in stock prices, and conversely, a decline in interest rates may exert a countervailing influence, bolstering stock prices (Prastowo, 2008).
Furthermore, Hooker (2004) has also ascertained a deleterious relationship between interest rates and market returns, highlighting the adverse impact of interest rate fluctuations on market performance.
Jakarta Composite Index (JCI/IHSG), serves as a vital gauge within the realm of capital markets. Shares, representing ownership certificates in companies, confer control and influence in proportion to the quantity of shares held (Salim, 2010). In the context of market dynamics, indices assume a pivotal role as indicators of prevailing trends. A market constitutes a convergence point where sellers and buyers engage in transactions for goods or services.
The primary function of a stock index is to discern and articulate the prevailing market conditions, whether
they entail an ascent or descent. A downtrend in stock prices signifies a diminishing stock index, while an upturn in stock prices signifies a corresponding increment in the stock price index (Ardana, 2016). As outlined by Manurung (2008), stock prices are categorized into three distinct groups.
The first one is the consideration of the spot price as the focal point of investor attention. Secondly, the market price signifies the spread between the highest and lowest purchase prices. Thirdly, the limit order, or limit price, designates a predetermined price aligning with investor preferences. Consequently, investors must diligently oversee the evolution of their stock holdings within the secondary market.
The interplay of supply and demand for stock prices is contingent upon a myriad of determinants. These factors encompass stock-specific variables, notably the performance of the underlying company, alongside macroeconomic influences such as interest rates, inflation, exchange rates. Additionally, non-economic considerations, including social,
economic, and political conditions, and a spectrum of other contributing factors, collectively engender fluctuations in stock prices (Azis, Mintarti, & Nadir, 2015).
The Jakarta Composite Index (JCI) is computed employing the Market Value Weighted Average Index methodology, as explicated by Halim & Chandra (2011)and elucidated further by Mie & Agustina (2014). The JCI calculation proceeds as follows:
JCIt = — x 100
j nd
Notes :
JCIt : Composite Stock Price
Index on day t
NPt : Market value on day t,
from the number of shares listed on the exchange multiplied by the market price per share.
ND : Base value
Within the Indonesia Stock Exchange (IDX), alongside the Jakarta Composite Index (JCI), there exists the Islamic Stock Index (ISSI). The ISSI was established by the IDX on May 12, 2011, and it encompasses all stocks featured in the Sharia Securities List and traded on the IDX, as documented by Pasaribu & Firdaus (2013). It's worth noting that there
exist distinctions between the Jakarta Islamic Index (JII) and ISSI. Specifically, ISSI encompasses all Sharia-compliant stocks listed on the IDX, whereas JII comprises a subset of the Sharia-compliant companies within ISSI, as articulated by (Chotib & Huda, 2019).
Samsul (2006), in his scholarly work, expounds upon a multitude of elements that possess the potential to detrimentally impact the stock market. These encompass facets such as political stability, domestic security, and the economic well-being of society. Suciningtias & Khoiroh, (2013) assert that macroeconomics stands as a paramount consideration among participants in the stock exchange. Alterations within the macroeconomic landscape have the capacity to induce consequential shifts in capital market dynamics, delineated by fluctuations in stock prices. As posited by Candy & Winardy (2018), these price oscillations can be attributed to a constellation of factors, the repercussions of which are distinctly discernible within the domain of macroeconomic influences. As (Azis et al., 2015), the conventional gauge for assessing the uppermost and
lowermost bid prices pertaining to stocks listed on the JATS encompasses both regular market stock trading and the cash market. This measure comprises two key components: (1) the opening market price, and (2) the closing market price within the regular market on the preceding trading day, referred to as the "previous Price" in instances where the opening Price is yet to be established.
METHODS
This study adopts a quantitative methodology, employing time series data spanning quarterly intervals within the timeframe spanning from 2000.1 to 2020.4. Data for this research has been gathered from diverse sources, including online platforms such as www.idx.go.id, Bank Indonesia's official website (www.bi.go.id), the Central Bureau of Statistics (www.bps.go.id), and reports furnished by the Indonesia Stock Exchange.
The variables used in this study are as follows:
-
1. The Jakarta Composite Index (JCI) represents the aggregate of all listed stock prices utilized by investors as a pivotal instrument for stock exchange
investments. The utilized data consists of the adjusted month-end closing prices of the JCI presented in index form.
-
2. Inflation is a broad upswing in the prices of goods or services, leading to a decline in purchasing power and subsequently impacting the overall cost of living, measured in percentage terms.
-
3. The rupiah exchange rate signifies the valuation of the rupiah relative to the US dollar, denominated in rupiah.
-
4. The Deposit Interest Rate in this study refers to the quarterly interest rate applied to deposits, expressed in percentage terms.
-
5. Money supply denotes the total amount of money in circulation, encompassing both currency held by the public and demand deposits in banks, measured in rupiah.
-
3.1 Model Analysis
The analytical framework employed for scrutinizing the dynamics of variables in this study is the Vector Error Correction Model (VECM), pioneered by Engle and Granger to rectify short-term imbalances in relation to the
long run. Essentially, the VECM is designed to estimate data that lacks stability (stationarity) at the level (O) but is cointegrated. In essence, this model represents a constrained form of VAR (Vector Autoregression). Its configuration involves detrending the long-term relationship of endogenous variables to converge towards their cointegrating relationship. When data from the VAR model is cointegrated, the VECM is applicable, earning its designation as a VAR model for non-stationary time series data characterized by a cointegrating relationship, hence commonly referred to as a restricted VAR (Sembiring, 2016).
The initial step in employing the VECM model approach involves the Stationarity Test, which can be conducted through various methods. Time series plots are one such method, where constancy in both average and variance over time indicates data tendency toward stationarity. Additionally, the unit root test, encompassing the Augmented Dickey-Fuller (ADF) test, the Phillips Perron (PP) test, and the Kwiatkowski Phillips Schmidt Shin (KPSS) test, is utilized for testing data stationarity. The
determination of stationarity is based on the significance level, where data is considered stationary if the p-value is less than the specified significance level. In this study, time series data undergoes validation or stability assessment through the Augmented Dickey-Fuller (ADF) unit root test. : .
k
DXt = αn + a}BX, Y b,B1DXt t 0 1t i t
i+1
k
DXt = cn + c, T + cBXf + V d,B1DXt t 01 t i t
i—1
-
3.2 Optimal Lag Assesment
Following the unit root test, the determination of the optimal lag length becomes imperative to ensure a dynamic, comprehensive, and efficient explanation by the model. The optimal lag length should strike a balance, avoiding being excessively short or overly long. A lag length that is too short compromises the model's ability to thoroughly capture its dynamics, while an excessively long lag hampers the efficiency of the model's measurement.
-
3.3 VAR Stability Test
Following the completion of the unit root test and the determination of the Optimal Lag, the subsequent step
involves the VAR Stability Test. This test is imperative to pave the way for the subsequent forecasting stage utilizing Impulse Response Function (IRF) and Variance Decomposition (VD).
-
3.4 Cointegration Test
The purpose of this test is to ascertain whether each variable attains equilibrium in the long run. The trace statistic serves as the criterion for cointegration testing, where if the trace statistic exceeds the critical value of 5%, it indicates cointegration within the equation. The cointegration test elucidates the number of equations cointegrated in the system. In the presence of cointegration in the system, the analysis proceeds to the subsequent stage utilizing the Vector Error Correction Method (VECM). Conversely, if no cointegration is identified, the analysis continues with the VAR approach.
-
3.5 Granger Causality Test
The objective of this test is to examine whether endogenous variables can function as exogenous variables. In situations where the relationship between two variables is unknown—whether one variable impact another, whether both
variables influence each other, or if there is no mutual influence—this test is employed to shed light on such causal relationships.
-
3.6 VECM Regression Model
The utilization of the VECM analysis tool offers the advantage of discerning the response of each variable to shocks induced by other variables in the study. This is achieved through the Impulse Response Function (IRF) which illuminates how variables react to shocks, and the Variance Decomposition (VD) which quantifies the contribution of each variable. The VECM model provides insights into both short-term and longterm components, as elucidated by (Sulistiana, 2017).
-
3.7 Impulse Response Function (IRF)
The Impulse Response Function serves as a crucial analytical tool within VECM, offering insights into the magnitude of shocks to a variable and their impact on other variables over a specified time span. Furthermore, it provides information on the duration of the effect of a variable shock on other variables until the impact reverts to the
equilibrium point, as outlined by (Gujarati, D. N., & Porter, 2009).
-
3.8 Variance Decomposition (VD)
Variance Decomposition, an analytical tool within VECM, serves to quantify the extent of influence that each variable exerts on other variables, including itself. By examining the results of Variance Decomposition, researchers gain insights into the magnitude of differences before and after a shock originating from other variables and the variable itself, as elucidated by (Gujarati, D. N., & Porter, 2009).
RESULTS AND DISCUSSION
This section unveils the outcomes of the conducted estimations. The initial step in this research involves a stationarity test applied to all variables to ascertain the integration level of each variable. The unit root test proposed by Dickey and Fuller, specifically the Augmented Dickey Fuller (ADF) test, is employed for this purpose. The results of the stationarity test at level (O) indicate that none of the variables exhibit stationarity at α = 1%, 5%, or 10%, as illustrated in the subsequent table:
Table 1. Estimated Unit Root Test at Level (0)
Variabel |
Prob |
Conclusion |
Inf |
0.3667 |
p> 0,05 (not stationary at level) |
Ihsg |
0.1163 |
p> 0,05 (not stationary at level) |
Ir |
0.1820 |
p> 0,05 (not stationary at level) |
Kurs |
0.4246 |
p> 0,05 (not stationary at level) |
Ms |
1.0000 |
p> 0,05 (not stationary at level) |
Source: Data Processing Results
Subsequently, stationarity testing is conducted at the first difference (1) level. The test
results are outlined in the following table:
Table 2. Estimated Unit Root Test at First Difference (1)
Variable |
Prob |
Conclusion |
Inf |
0.0000 |
p< 0,05 (stationary at first difference) |
Ihsg |
0.0000 |
p< 0,05 (stationary at first difference) |
Ir |
0.0012 |
p< 0,05 (stationary at first difference) |
Kurs |
0.0000 |
p< 0,05 (stationary at first difference) |
ms |
0.0000 |
p< 0,05 (stationary at first difference) |
Source: Data Processing Results on the Akaike Information Criterion (AIC)
Table 2 reveals that all variables demonstrate stationarity at the first difference (1) level. This is evident as all variables possess probabilities below the alpha level of 0.05, signifying the existence of a long-term relationship.
Determination of Lag Length
Following the attainment of stationarity at the 1st Difference level for all variables, the VAR Lag Length Criteria test is executed. The results of this estimation test (refer to Table 4.3) dictate that the optimal lag length, chosen based
criteria, is lag one for the subsequent VECM equation estimation process. As outlined by Gujarati, D. N., & Porter (2009) the Akaike Information Criterion (AIC) offers the advantage of selecting the most fitting regression for the forecasting process, adept at elucidating the model's compatibility with both in-sample forecasting (fit with historical data) and out-of-sample forecasting (future predictions).
Tabel 3. Estimasi Uji Lag Optimum | ||||
Lag LogL LR |
AIC |
SC |
HQ | |
0 -58.02224 NA |
1.575556 1.724433* |
1.635245* | ||
1 -26.16618 58.93370* |
1.404154* 2.297414 |
1.762288 | ||
2 -16.72149 16.29209 |
1.793037 3.430681 |
2.449615 | ||
3 4.754534 34.36164 |
1.881137 4.263163 |
2.836159 | ||
*Lag Optimum Source: Data Processing Results |
within the unit |
circle, or |
when their | |
Estimation of VAR Stability Test |
absolute values |
(modulus) |
are smaller | |
The VAR stability test involves the |
than one. This |
renders the VAR test | ||
calculation of unit roots in the polynomial |
estimation valid. |
The ensuing outcomes | ||
function. A VAR is deemed stable when |
present the results of |
the VAR | ||
all roots of the polynomial function reside |
Stabilisation |
Test |
Estimation: |
Tabel 4. Estimation of VAR Stability Test
Root |
Modulus |
0.655103 |
0.655103 |
-0.202349 - 0.026339i |
0.204056 |
-0.202349 + 0.026339i |
0.204056 |
-0.028153 - 0.073488i |
0.078696 |
-0.028153 + 0.073488i |
0.078696 |
No root lies outside the unit circle. |
VAR satisfies the stability condition. Source: Data Processing Results
On the VAR test estimation results presented in Table 4, it is evident that the VAR model exhibits stability and successfully passes the stability test. This is confirmed by all modulus values being smaller than 1. Graphically, as illustrated
in Figure 1, all points fall within the circle. This condition signifies the stability of the estimated VAR model, thereby enabling the continuation of the Impulse Response Function (IRF) and Variance Decomposition (VD) analyses.
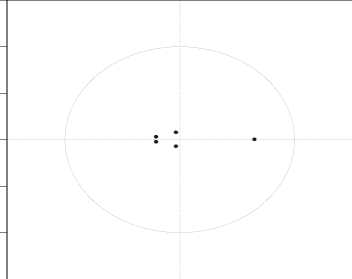
Inverse Roots of AR Characteristic Polynomial 1.5
1.0
0.5
0.0
-0.5
-1.0
-
-1.5 n----------------1----------------τ----------------i----------------1----------------1----------------1
-
-1.5 -1.0 -0.5 0.0 0.5 1.0 1.5
Figure 1. VAR Stability Test Graph
1.5 -1.0 -0.5 0.0 0.5 1.0 1.
The Granger Causality Test
The Granger Causality Test is implemented to discern any reciprocal relationships among the variables under investigation (IF, IHSG, IR, KURS, and MS). Employing the VAR Pairwise Granger Causality Test approach with a significance level of 5%, causality between
variables is established if the probability is less than alpha 5%. The rejection of H0 implies that a variable significantly influences other variables. The ensuing outcomes delineate the results of the causality test estimation.
Table 5. Granger Causality Test Estimation
Null Hypothesis: |
Obs F-Statistic |
Prob. |
Conclusion | |
IHSG does not Granger Cause IF |
83 |
1.30739 |
0.2563 | |
IF does not Granger Cause IHSG |
0.60438 |
0.4392 | ||
IR does not Granger Cause IF |
83 |
8.89785 |
0.0038 |
One way |
IF does not Granger Cause IR |
2.28134 |
0.1349 | ||
KURS does not Granger Cause IF |
83 |
4.18340 |
0.0441 |
Two way |
IF does not Granger Cause KURS |
7.43795 |
0.0078 | ||
MS does not Granger Cause IF |
83 |
8.84408 |
0.0039 |
One way |
IF does not Granger Cause MS |
0.08445 |
0.7721 | ||
IR does not Granger Cause IHSG |
83 |
4.28198 |
0.0417 |
One way |
IHSG does not Granger Cause IR |
0.05359 |
0.8175 | ||
KURS does not Granger Cause IHSG |
83 |
0.37460 |
0.5422 | |
IHSG does not Granger Cause KURS |
0.41103 |
0.5233 | ||
MS does not Granger Cause IHSG |
83 |
0.63044 |
0.4295 | |
IHSG does not Granger Cause MS |
1.03840 |
0.3113 | ||
KURS does not Granger Cause IR |
83 |
0.88034 |
0.3509 | |
IR does not Granger Cause KURS |
3.21925 |
0.0766 |
MS does not Granger Cause IR |
83 1.34514 |
0.2496 |
IR does not Granger Cause MS |
1.40247 |
0.2398 |
MS does not Granger Cause KURS |
83 7.33090 |
0.0083 One way |
KURS does not Granger Cause MS |
0.66466 |
0.4173 |
Source: Data Processing Results
The results of the estimation reveal the
following relationships:
-
1. IF significantly influences IR in a unidirectional manner, with a p-value of 0.0038 < 0.05.
Conversely, IR does not exert a significant effect on IF.
-
2. KURS significantly impacts IF in a bidirectional relationship, with p-values for both variables below 0.05.
-
3. MS significantly affects IF in a unidirectional manner, with a p-value of 0.0039 < 0.05. However, IF does not have a significant effect on MS.
-
4. IR significantly influences IHSG in a unidirectional manner, with
a p-value of 0.0417 < 0.05. Meanwhile, IHSG does not have a significant effect on IR.
-
5. MS significantly affects KURS in a unidirectional manner, with a p-value of 0.0417 < 0.05. Nevertheless, KURS does not have a significant effect on MS.
Johansen Co-integration Test
This test was carried out using the Johansen Cointegration Test method and Cointegration Test uses lag 1. The test criteria involve assessing the comparison between the Trace Statistic value and the Critical Value (0.05), along with examining the probability value. The estimation results are presented in Table 6 below:
Table 6. Johansen Cointegration Test Estimation
Hypothesized |
Trace |
0.05 | ||
No. of CE(s) |
Eigenvalue |
Statistic |
Critical ValueProb.** | |
None * |
0.441914 |
170.5259 |
69.81889 |
0.0000 |
At most 1 * |
0.408580 |
123.2832 |
47.85613 |
0.0000 |
At most 2 * |
0.340133 |
80.73966 |
29.79707 |
0.0000 |
At most 3 * |
0.311136 |
47.06664 |
15.49471 |
0.0000 |
At most 4 * |
0.188083 |
16.87695 |
3.841466 |
0.0000 |
Trace test indicates 5 cointegrating eqn(s) at the 0.05 level Source: Data Processing Results
The estimation results of the Cointegration test in Table 4.6 indicate that the 5 variables are cointegrated in this study, with the p-value < 5% alpha or trace statistic value of 170.5259 > 69.81889 at 5% alpha. In econometric analysis, this condition signifies a stable long-term equilibrium relationship among the variables studied (IF, IHSG, IR, KURS, and MS). In the short term, all variables still adjust to each other, suggesting that the long-term relationship is more robust than the short term, allowing for the application of the VECM model.
VECM Model Estimation
The VECM Model estimation results in Table 7 reveal that the CointEq1 value (-
-
5.81798) is significant, meeting the criteria as a model applicable in this study. The VECM Model generally comprises two parts: part 1 depicting long-term relationships and part 2 showing shortterm relationships.
In the short-term estimation, it is observed that only the interest rate (IR) significantly and positively influences inflation (IF) with a coefficient is 1.558465. This implies that every 1% increase in interest rates (IR) will lead to a 1.558465% rise in inflation (IF).
Table 7. VECM Model Estimation
1. Long Term |
2. Short term | ||
Cointegrating Eq: |
CointEq1 |
Error Correction: D(IF) | |
IF(-1) |
1.000000 |
CointEq1 |
-0.377016 [-5.81798]* |
LOG(IHSG(-1)) |
0.192923 | ||
(0.16862) | |||
[ 1.14415] |
D(IF(-1)) |
-0.086905 | |
IR(-1) |
-0.003535 |
(0.12960) | |
(0.25530) |
[-0.67055] | ||
[-0.01384] |
D(LOG(IHSG(-1))) |
0.011725 | |
LOG(KURS(-1)) |
-1.555316 |
(0.16492) | |
(4.08616) |
[ 0.07109] |
[-0.38063] |
D(IR(-1)) |
1.558465 | |
LOG(MS(-1)) |
4.439841 |
(0.31953) | |
(1.65757) |
[ 4.87740]* | ||
[ 2.67853]* |
D(LOG(KURS(-1))) |
-1.890369 | |
C |
-58.91245 |
(3.40655) | |
[-0.55492] | |||
D(LOG(MS(-1))) |
0.115515 | ||
(4.53075) | |||
[ 0.02550] | |||
C |
0.125734 | ||
(0.21664) | |||
[ 0.58039] |
Source: Data Processing Results
In the long run, only the variable money supply (MS) significantly and positively affects the level of inflation (IF) with a coefficient of 4.439841. This implies that every 1% increase in money supply (MS) will result in a 4.439841% increase in inflation. This finding aligns with the theory and hypothesis suggesting that an increase in money supply (MS) in the economy leads to an increase in inflation (IF).
Impulse Response Function (IRF) Estimation
Impulse Response Function (IRF) can be derived after passing through the VAR and VECM stages, with the aim of observing the impact (shock) of a variable on other variables in both the short and
long term. The IRF illustrates how a variable reacts to a shock, whether originating from the variable itself or other variables. This analysis provides insights into both short-term (early period) and long-term dynamics.
Table 8 depicts the response to an interest rate shock (IF), revealing a dynamic and fluctuating pattern. In period 1, there is a positive response (1.458467%), but it starts to decline from period 5 (-0.066296) and reaches a negative response of -0.119587 by period 10. This pattern suggests that expansionary monetary and fiscal polJCIes have the potential to dampen
inflationary movements in the short term, contributing to anticipated support for macroeconomic growth in the future.
Table 8. Impulse Response Function (IRF)
Response of | ||||
IF Period |
IF LOG(IHSG) IR |
LOG(KURS) LOG(MS) | ||
1 |
1.458467 0.000000 |
0.000000 |
0.000000 |
0.000000 |
2 |
1.071053 -0.086459 |
0.693497 |
-0.060315 |
-0.054603 |
3 |
0.659585 -0.088477 |
0.905679 |
-0.035718 |
-0.163660 |
4 |
0.246396 -0.131277 |
0.834065 |
0.017112 |
-0.197102 |
5 |
-0.066296 -0.169490 |
0.596222 |
0.066691 |
-0.208997 |
6 |
-0.246836 -0.201738 |
0.314143 |
0.108000 |
-0.195958 |
7 |
-0.306381 -0.221513 |
0.069045 |
0.133240 |
-0.173105 |
8 |
-0.278879 -0.229594 |
-0.097670 |
0.143428 |
-0.148953 |
9 |
-0.204898 -0.228654 |
-0.178881 |
0.142057 |
-0.129646 |
10 |
-0.119587 -0.222478 |
-0.189734 |
0.134015 |
-0.117648 |
Source: Data Processing Results. Interestingly, the contribution of
Data Processing Results indicate that in period 5, the impact of the interest rate (IF) shock diminishes, as evidenced by the movement of JCI (IHSG) decreasing by -0.169490. However, the contribution of interest rate (IR) increased by 0.596222%, and exchange rate (KURS) showed a slight increase (0.066691), while money supply (MS) experienced a decrease (-0.208997). By period 10, inflation continues to decline (-0.119587), along with JCI (IHSG) (0.222478), interest rate (IR) (-0.189734), and money supply (MS) (-0.117648).
exchange rate (KURS) increases (0.134015).
Variance Decomposition (VD) Estimation
The Variance Decomposition (VD) Test Estimation, an analytical tool in VECM, is used to quantify each variable's influence on others and itself from period 1 to period 10.
Table 9. Variance Decomposition of D (INFLASI)
Variance Decomposition of IF: | |||||
Period |
IF |
LOG(IHSG) |
IR |
LOG(KURS)LOG(MS) | |
1 |
100.0000 |
0.000000 |
0.000000 |
0.000000 |
0.000000 |
2 |
86.86677 |
0.198318 |
12.75930 |
0.096515 |
0.079099 |
3 |
73.29962 |
0.302409 |
25.71267 |
0.097100 |
0.588201 |
4 |
64.18998 |
0.553985 |
33.99912 |
0.088649 |
1.168265 |
5 |
59.81688 |
0.970901 |
37.27958 |
0.153001 |
1.779639 |
6 |
58.46271 |
1.554209 |
37.36108 |
0.324955 |
2.297043 |
7 |
58.16104 |
2.235562 |
36.35091 |
0.578337 |
2.674146 |
8 |
57.74978 |
2.936384 |
35.53079 |
0.859582 |
2.923463 |
9 |
57.01342 |
3.605069 |
35.16460 |
1.123976 |
3.092931 |
10 |
56.17388 |
4.223758 |
35.02253 |
1.351821 |
3.228010 |
Source: Data Processing Results declines to 4.223758%, interest rate (IR) The findings from the Data contribution decreases by 35.02253%, |
Processing Results indicate that in the initial period (Period 1), Inflation independently contributed 100% to its own variation, unaffected by external variables such as JCI (IHSG), interest rate (IR), exchange rate (KURS), and money supply (MS). By Period 5, the contribution of Inflation (IF) begins to decline (59.81688%), but other variables start to exhibit their impact. JCI (IHSG) contributes 0.970901% to the formation of inflation (IF), and Interest Rate (IR) significantly contributes with 37.27958%, while exchange rate (KURS) contributes 0.153001%, and money supply (MS) sees an increase to 1.779639%. In the 10th
exchange rate (KURS) contribution remains relatively stable at 1.351821%, and money supply (MS) contribution increases to 3.228010%.
The observed movement of macroeconomic variables influencing inflation in each period suggests the effectiveness of government (fiscal) and Bank Indonesia (monetary) policies in addressing inflation. Over the next 10 periods, the downward trend in the inflation rate implies favorable economic growth with a continuous positive trajectory.
CONCLUSION AND POLICY IMPLICATIONS
period, the contribution of IF continues
Based on the outcomes of the data
to decrease (56.17388%), JCI (IHSG)
analysis, it is evident that all variables
under scrutiny exhibit stationarity upon differencing, thereby rendering the Vector Error Correction Model applicable and consequently employed as the fundamental model within this study. The results of the cointegration test, conducted via the Johansen CoIntegration test, elucidate the existence of a long-term relationship among each variable, namely the inflation rate, Jakarta Composite Index, interest rate, exchange rate, and money supply. Furthermore, the Granger Causality Test reveals a unidirectional causal relationship, with the inflation rate significantly influencing the interest rate, money supply significantly affecting the inflation rate, interest rate significantly affecting the Jakarta Composite Index, money supply significantly impacting the exchange rate, and a reciprocal causal connection between the exchange rate and inflation rate emerging within the short-term context.
The interest rate exhibits a notable and positive impact on inflation; however, its long-term influence on inflation turns negative and statistically insignificant. Conversely, over the long
run, the money supply exerts a significant and positive effect on the inflation rate. Analyzing the Impulse Response Function reveals a considerable degree of volatility in the response of the interest rate over a 10-period horizon, albeit with an overall positive trend. The results of Variance Decomposition indicate that in the subsequent 10 periods, the Jakarta Composite Index will contribute 4.223758%, the interest rate 35.02253%, the exchange rate 1.351821%, and the money supply 3.228010% to the shaping of the inflation rate within the economy.
RECOMMENDATION
Drawing from the findings of this investigation, which underscore a causative linkage between exchange rates and the inflation rate, it becomes imperative for the government to exercise prudence in formulating policies, particularly in the context of expansive monetary policy, to preserve the stability of inflation. The propensity for volatile fluctuations in inflation to substantially impact the exchange rate accentuates the importance of such vigilance. This study aspires to
contribute to the research community by offering insights into econometric model development, with the prospect of integrating additional pertinent variables that were not encompassed within this study's scope.
REFERENCES
Adisetiawan, R. (2014). Keseimbangan Jangka Panjang antara Variabel Makro Ekonomi dengan Indeks Harga Saham. Trikonomika, 2(10).
Ahmed, R., Chen, X. H., Kumpamool, C., & Nguyen, D. T. K. (2023). Inflation, oil prices, and economic activity in recent crisis: Evidence from the UK. Energy Economics, 126(July), 106918. https://doi.org/10.1016/j.eneco.202 3.106918
Amalia, S., Wijaya, A., Darma, D. C., Maria, S., & Lestari, D. (2020). Underground Economy Teori & Catatan Kelam. Yayasan Kita Menulis.
Andiantyo, P., Sihombing, P., Kusumastuti, S. Y., Ekonomi, M. I., Trisakti, U., Ekonomi, F., … Model, C. (2018). PERGERAKAN INDEKS HARGA SAHAM SEKTOR PERTANIAN DI BURSA. Seminar Nasional Cendekiawan Ke 4, 1137– 1148.
Antonakakis, N., Gupta, R., & Tiwari, A. K. (2017). Has the correlation of inflation and stock prices changed in the United States over the last two centuries? Research in International Business and Finance, (42).
Ardana, Y. (2016). Pengaruh Variabel
Makroekonomi terhadap Indeks Saham Syariah di Indonesia: Model ECM. Esensi Jurnal Bisnis Dan Manajemen, 6(1).
https://doi.org/https://doi.org/10. 15408/ess.v6i1.3118.
Astuti, R., Lapian, J., & Rate, P. Van. (2016). Pengaruh Faktor Makro Ekonomi Terhadap Indeks Harga Saham Gabungan (IHSG) di Bursa Efek Indonesia (BEI) periode 20062015. Jurnal Berkala Ilmiah Efisiensi, 16(2).
Azis, M., Mintarti, S., & Nadir, M. (2015). Manajemen Investasi Fundamental, Teknikal, Perilaku Investor dan Return Saham. CV. Budi Utama.
Beik, I. S., & Fatmawati, S. W. (2014). Pengaruh Indeks Harga Saham Syariah Internasional dan Variabel Makroekonomi terhadap Jakarta Islamic Index. Al-Iqtishad, 4(2).
Candy, C., & Winardy, A. (2018).
Pengaruh Faktor Ekonomi Makro Terhadap Stock Return Pada Indeks Saham LQ45. Jurnal Ekonomi & Ekonomi Syariah, 2(1).
https://doi.org/https://doi.org/10. 36778/jesya.v2i1.35
Chotib, E., & Huda, N. (2019). Analisis Pengaruh Variabel Ekonomi Makro terhadap Indeks Saham Syariah Indonesia ( ISSI ) periode 2016 – 2019. Journal of Economics and Business Aseanomics ( JEBA ), 4(1), 1– 20.
Desvina, A. P., & Lubis, P. S. (2019). Pendekatan VECM untuk Menganalisis Hubungan IHSG , BI Rate , Kurs ( USD / IDR ), dan Jumlah Uang yang Beredar ( M2 ). Jurnal Sains Matematika Dan Statistika, 5(1), 107–119.
Dornbusch, R., Fischer, S., & Starz, R. (2008). Makro Ekonomi (S. Roy Indra Mirazudin, ed.). Jakarta: PT Media Global Edukasi.
Easterly, W., & Schmidt-Hebbel, K. (1993). FISCAL DEFJCITS AND MACROECONOMIC
PERFORMANCE IN DEVELOPING COUNTRIES. The World Bank Research Observe, 8(2), 211–237.
https://doi.org/https://doi.org/10. 1093/wbro/8.2.211
Fahmi, I. (2012). Pengantar Pasar Modal. Bandung: Alfabeta.
Fred, W. J., & F.Eugene, B. (1994).
Essentiol of Managerial Finance (10th ed.). New York: The Dryden Press.
Gujarati, D. N., & Porter, D. C. (2009). Basic Econometrics (5th ed.). McGraw-Hill.
Halim, S., & Chandra, A. (2011). Pemodelan Time Series Multivariat secara Automatis. Jurnal Teknik Industri, 13(1), 19–26.
Hamburger, M. J., & Zwick, B. (1981). DefJCIts, money and inflation. Journal of Monetary Economics Volume 7, Issue 1, 1981, Pages 141-150, 7(1), 141–150.
https://doi.org/https://doi.org/10. 1016/0304-3932(81)90057-X
Haryogo, A. (2013). Pengaruh Nilai Tukar dan Indeks Down Jones terhadap Composite Index di Bursa Efek Indonesia. FINESTA, 1(1).
Hasyim, A. I. (2017). EKONOMI MAKRO. Kencana.
Hendajany, N. (2015). Tingkat Inflasi, Suku Bunga Bank Indonesia, Jumlah Uang Beredar dan Kurs. Eko-Regional, 10(1), 39–47.
Hooker, M. A. (2004). Macroeconomic Factors and Emerging Market
Equity Returns: A Bayesian Model Selection Approach. Emerging Markets Review, 5, 379‐387.
Ishaq, T., & Mohsin, H. M. (2015). DefJCIts and inflation; Are monetary and financial institutions worthy to consider or not? Borsa Istanbul Review, 15(3), 180–191.
https://doi.org/10.1016/j.bir.2015.0 3.002
Janah, I. U., & Pujiati, A. (2018). Analisis Mekanisme Transmisi Kebijakan Moneter Jalur Ekspektasi dalam Mempengaruhi Inflasi di Indonesia. Economics Development Analysis Journal, 7(4), 384–394.
Krisna, A. A. G. A., & Wirawati, N. G. P. (2013). PENGARUH INFLASI, NILAI TUKAR RUPIAH, SUKU BUNGA SBI PADA INDEKS HARGA SAHAM GABUNGAN DI BEI. E-Jurnal Akuntansi Universitas Udayana, 3(2), 421–435.
Luwihadi, N. L. G. A., & Arka, S. (2017). Determinan Jumlah Uang Beredar dan Tingkat Inflasi di Indonesia Periode 1984-2014. E-Jurnal Ekonomi Pembangunan Universitas Udayana, 6(4).
Mankiw, N. G. (2007). Makroekonomi (6th ed.). Jakarta: Erlangga.
Manurung, A. H. (2008). Strategi Menangkan Transaksi Saham di Bursa. PT Elex Media Koputindo.
Mie, M., & Agustina. (2014). Analisis Pengaruh Indeks Harga Saham Gabungan Asing Terhadap Indeks Harga Saham Gabungan Indonesia. Jurnal Wira Ekonomi Mikroskil, 4(2), 81–90.
https://doi.org/10.55601/jwem.v4i 2.216
Mishkin, F. S. (2010). 2010. Ekonomi Uang,
Perbankan, dan Pasar Keuangan. Jakarta: Salemba Empat. Jakarta: Salemba Empat.
Natsir, M. N. (2018). Analisis Empiris Efektivitas Mekanisme Transmisi Kebijakan Moneter di Indonesia Melalui Jalur Ekspektasi Inflasi. EKUITAS (Jurnal Ekonomi Dan Keuangan), 13(3), 288–307.
Nisa, N. K., & Sukmana, R. (2017). ANALISIS PENGARUH VARIABEL – VARIABEL MAKROEKONOMI TERHADAP JAKARTA ISLAMJCINDEKS (JII) Periode 20072015. Jurnal Ekonomi Syariah Teori Dan Terapan, 4(6), 449–463.
Nofiatin, I. (2013). Hubungan Inflasi, Suku Bunga, Produk Domestik Bruto, Nilai Tukar, Jumlah Uang Beredar, dan Indeks Harga Saham Gabungan (IHSG) Periode 2005– 2011. Jurnal Aplikasi Manajemen, 11(2), 215–222.
Nugroho, H. (2008). ANALISIS PENGARUH INFLASI, SUKU BUNGA, KURS DAN JUMLAH UANG BEREDAR TERHADAP INDEKS LQ45. Diponegoro.
Pasaribu, R. B. F., & Firdaus, M. (2013). Analisis Pengaruh Variabel Makroekonomi Terhadap Indeks Saham Syariah Indonesia. Jurnal Ekonomi Dan Bisnis (JEB), 7(2), 117– 132.
Prastowo, N. J. (2008). Dampak BI Rate Terhadap Pasar Keuangan: Mengukur Signifikansi Respon Instrumen Pasar Keuangan terhadap Kebijakan Moneter. Working Paper No.21. Bank Indonesia. Bank Indonesia.
Rusbariand, S. P., & Herawati, S. (2012). Analisis Pengaruh Tingkat Inflasi,
Harga Minyak Dunia, Harga Emas Dunia, Dan Kurs Rupiah Terhadap Pergerakan Jakarta Islamic Index Di Bursa Efek Indonesia. Forum Bisnis & Keuangan, 1(1), 724–740.
Salim, J. (2010). Cara gampang bermain saham. Transmedia Pustaka.
Salisu, A. A., Isah, K. ., Oyewole, O, J., & Akanni, L. (2017). Modelling oil price-inflation nexus: the role of asymemetries. Energy, 125, 97–106.
Samsul, M. (2006). PASAR MODAL DAN MANAJEMEN PORTOFOLIO. Erlangga.
Sembiring, M. (2016). Analisis Vector Autoregresion (VAR) Terhadap Interrelationship Antara IPM Dan Pertumbuhan Ekonomi Di Sumatera Utara. EKONOMIKAWAN: Jurnal Ilmu Ekonomi Dan Studi Pembangunan, 16(2), 114–123.
https://doi.org/10.30596/ekonomik awan.v16i2.939
Suciningtias, S. A., & Khoiroh, R. (2013). Analisis Dampak Variabel Makro Ekonomi Terhadap Indeks Saham Syariah Indonesia. Conference in Business, Accounting, and Management, 2(1), 398–412.
Sulistiana, I. (2017). Model Vector Auto Regression (Var) and Vector Error Correction Model (Vecm) Approach for Inflation Relations Analysis, Gross Regional Domestic Product (Gdp), World Tin Price, Bi Rate and Rupiah Exchange Rate. Integrated Journal of Business and Economics, 1(2), 17–32.
https://doi.org/https://doi.org/10. 5281/zenodo.1147673
Tandelilin, E. (2010). Portofolio dan Investasi Teori dan Aplikasi (1st ed.). Yogyakarta: Kanisius.
Tangjitprom, N. (2012). The Review of Macroeconomic Factor and Stock Return. International Bussines
Research, 5(8).
Tanuwidjaya, W. (2008). Siasat Investasi
Cerdik: Bagi Investor Pemula.
MedPres.
Wang, X., Huang, Q., & Zhang, S. (2023). Effects of macroeconomic factors on stock prices for BRICS using the variational mode decomposition and quantile method. The North American Journal of Economics and Finance, 67. https://doi.org/https://doi.org/10. 1016/j.najef.2023.101939
Widoatmodjo, S. (2015). Pengetahuan
Pasar Modal untuk Konteks Indonesia.
PT. Elex Media Komputindo.
Wildauer, R., Kohler, K., Aboobaker, A.,
& Guschanski, A. (2023). Energy price shocks , conflict inflation , and income distribution in a three-sector
model ☆. Energy Economics, 127(PB), 106982.
https://doi.org/10.1016/j.eneco.202 3.106982
Yıldırım, D. (2017). Empirical investigation of purchasing power parity for Turkey: Evidence from recent nonlinear unit root tests.
Central Bank Review, 17(2), 39–45. https://doi.org/10.1016/j.cbrev.201 7.03.001
35
Discussion and feedback