A SPATIAL STUDY OF LAND AND FOREST FIRE-PRONE AREAS IN SITUBONDO REGENCY, EAST JAVA PROVINCE
on
A Spatial Study of Land and Forest Fire-Prone Areas...,
[Haeropan Daniko Dwiputra, dkk]
A SPATIAL STUDY OF LAND AND FOREST FIRE-PRONE AREAS IN SITUBONDO REGENCY, EAST JAVA PROVINCE
Haeropan Daniko Dwiputra 1), Masita Dwi Mandini Manessa 1)*, Rokhmatullah 1), Anisya Feby Efriana1), Muhammad Haidar2,3)
-
1)Geography Department, Faculty of Mathematics and Science, Universitas Indonesia, Depok, West Java, Indonesia
-
2)Takeuchi Laboratory, Remote Sensing of Environment and Disaster, Institute of Industrial Science, The University of Tokyo University of Tokyo, Tokyo, Japan 3)Geospatial Information Institute, Cibinong, West Java, Indonesia
* Email: [email protected]
ABSTRACT
The increasing area of land burned in 2021 makes the government urgent to map areas prone to forest fires in Situbondo Regency. This study analyzes areas prone to forest and land fires using the SMCA method. The research analysis used variables of land cover type, the greenness of vegetation, vegetation humidity, land surface temperature, and human factors. The human elements in question are accessibility (distance from the road network) and distance from human activities (distance from settlements, fields, and plantations). The conclusion analysis of forest fire-prone areas is divided into three classes that are high, medium, and low. From the vulnerability model that has emerged, it was found that most of Situbondo Regency have a high grade of forest fire vulnerability with an area of 652.66 km² (39.08%). The areas with the level of vulnerability of the middle, low, and non-vulnerable classes, respectively, are 532.12 km² (31.87%), 306.46 km² (18.35%), and 178.65 km² (10, 70%). The results of statistical tests using the ordinal logistic regression method show that natural factors for forest and land fires had a higher level of influence (ψ = 4.824) on forest and land fire vulnerability compared to human factors (ψ = 1.051).
Keywords: Forest and Land Fires; GMA method; Natural Factors; Human Factor
According to Regulation of the Minister of Environment and Forestry No. 32 of 2016, forest and land fires are defined as incidents in which a forest and/or land are burned, either accidentally or intentionally, resulting in environmental damage and potential losses in terms of ecology, economy, sociocultural diversity, and politics. In Indonesia, forest and land fires happen yearly and frequently as a result of 2 (two) main causes: natural causes and human-caused causes (Mareta et al., 2020). El
Nino's effect and the presence of a protracted dry season are examples of natural factors that can result in forest and land fires, while intentional burning to clear land using the slash-slash-slash method is an example of a human activity component that can result in forest and land fires burn (Rasyid, 2014).
On the island of Java, the East Java Province experienced the most forest and land fires overall in 2021. 15,458 hectares of land were reported to have burned last year, with Situbondo Regency as the most damaged regency having a burned area of 8,186 hectares, or over three times as
much as the area in 2020 (Sipongi, 2021). It is also an urgent task to create a map of forest and land fire-prone areas that can be used by different parties as a means of prevention and alertness to forest and land fire disasters in the area since the amount of land and forest burned in the Situbondo Regency area in 2021 is expected to increase.
The National Disaster Management follows the elements of the catastrophe threat index contained in the National Disaster Management Perka No. 2 of 2012 Concerning General Disaster Risk Assessment Guidelines when creating forest and land fire hazard maps using the present method. Three categories of variables, including soil types, forest and land types, and climate, were employed by National Disaster Management in their mapping of the forest and land fires tragedy. The National Disaster Management technique still does not take into account local human elements that may contribute to forest and land fire tragedies. Whether deliberate or not, human activity is one of the leading
causes of forest and land fires, accounting for about 99% of all fires in these areas (Darwiati & Tuheteru, 2010). Land clearance is a common cause of forest and land fires throughout the dry season in Indonesia, making it challenging to control these types of flames (Langmann & Heil, 2004).
The causes that cause forest and land fires because of human activity are also taken into account in the research that will be done for this thesis. Finding the most suitable equation for mapping forests and areas vulnerable to forest fires in Situbondo Regency, East Java Province, involves weighing the natural and human elements that contribute to forest and land fires. Additionally, statistical analyses will be performed to assess the relative importance of the two variables. The findings of this study are planned to be utilized as a guide to identify regions in Situbondo Regency that are vulnerable to forest and land fires as well as a guide for future research.
-
2. Methodology
2.1 Data and Location
Figure 1.
Map Showing the Location of the Study Area
This research was conducted in Situbondo (Figure 1), East Java, Indonesia and the variables that will be considered in this study are land cover characteristics, vegetation's greenness, humidity level, temperature, and human aspects like accessibility (distance from a road) and proximity to human activities. The distance from human activities in question includes distance from towns, Table 1. Research Data
No |
Data |
Data Source |
Description |
1 |
Landsat-8 Imagery |
USGS, Google Earth Engine |
Resolution 30M. filter date: 1/1/2021-31/12/2021 |
2 |
City & Regency administrative boundaries |
Situbondo Regency’s RBI Map Data Processing |
Scale 1:25.000 |
3 |
Land Cover |
Landsat-8 Image Data Processing |
Resolution 10 M. filter date: 1/1/2021-31/12/2021 |
4 |
NDVI Vegetation Index |
Landsat-8 Image Data Processing |
Resolution 30 M/ filter date: 1/1/2021-31/12/2021 |
5 |
NDMI Humidity Index |
Landsat-8 Image Data Processing |
Resolution 30 M/ filter date: 1/1/2021-31/12/2021 |
6 |
Land Surface Temperature |
Situbondo Regency RBI Map Data Processing |
Resolution 100M. filter date: 1/1/2021-31/12/2021 Cloud cover: <5% |
7 |
Situbondo Regency Road Network Distance from Human |
Situbondo Regency RBI Map Data Processing |
Scale 1:25.000 |
8 |
Activities Sites (Settlements, Fields, and Plantations) |
Situbondo Regency Space Pattern Plan Data Processing |
Scale 1:25.000 |
9 |
Forest and land fires area 2016-2017, 2019-2020 |
Ministry of Environment and Forestry |
- |
Source: Data Processing, 2022
In this study, Multi-Criteria Analysis (MCA) is a decision-making method that analyzes several influential variables (Wibowo & Semedi, 2011) to produce a map of forest and land fire hazards that can be used for the decision-making process. The data processing method was used to prepare the data for the final classification by the weight of the Spatial Multi-criteria Analysis (SMCA)
parameters. GEE and the Arcgis application program are both used to process data on parameters of forest and land fire-prone parameters in the Situbondo Regency. GEE is used to
distance from farms, and distance from plantations. These influential variables are related to the concept of the fire triangle and affect the vulnerability of forest and land fires in the research location (Yuliana et al., 2015). Table 1 will show the research data that were used as parameters for land and forest fire vulnerability modeling.
categorize surface temperature, vegetation index, humidity index, and data on land cover. The Arcgis program is used to process accessibility data variables (distance from the road network) as well as distance data from places of human activities that are likely to start forest and land fires and to carry out additional analysis. Table 2 shows how each variable that will be used in the SMCA was weighted.
From the previous study, a model of a forest area that is vulnerable to forest and land fires could be created using the SMCA analysis and is based on two separate equations. The first equation gives greater weight to the human factor,
while the second equation gives greater weight to the natural factor (Amalina et al., 2015). A third equation, which offers a fair weight to each factor, is also used in this current study to investigate whether giving a fair weight to both factors results in a more suitable model.
y=0.1*(x1+x2+x3+x4)+0.9*(x5+x6+x7+x8) y=0.9*(x1+x2+x3+x4)+0.1*(x5+x6+x7+x8) y=0.5*(x1+x2+x3+x4)+0.5*(x5+x6+x7+x8)
x1 = Types of Land Cover
x2 = NDVI Score
x3 = NDMI Score At least one
x4 = Land Surface Temperature
x5 = Distance from Road Network x6 = Distance from Settlement x7 = Distance from Field x8 = Distance from Plantation
The SMCA analysis process is carried out using 3 different equations as an alternative form of simulation modeling for forest and forest fire-prone areas in Situbondo Regency. The SMCA analysis will then produce 3 different vulnerability models which will be analyzed further. The weighting process of the two equations can be seen in Table 3.
Table 2. Weight of Each Variable for SMCA | |||||
No |
Variable |
Data Type/Variable |
Score |
Vulnerability Level |
Source |
1 |
Types of |
Savanna, Dry Land Farm |
5 |
Most Vulnerable | |
Land Cover |
Plantation Forest and Dryland Forest |
4 |
Vulnerable |
Sabaraji, | |
Mangrove forest |
2005. | ||||
Water Body, Settlements, Rice Fields |
2 |
Not Vulnerable |
Adapted. | ||
1 |
Least Vulnerable | ||||
2 |
NDVI |
<0.15 |
2 | ||
0.15 – 0.25 0.25 – 0.35 |
3 4 |
Least Vulnerable |
Nurdiana | ||
Not Vulnerable |
& | ||||
>0.35 |
5 |
Vulnerable |
Risdiyant | ||
Most Vulnerable |
o, 2015 | ||||
3 |
NDMI |
<0.15 |
5 |
Most Vulnerable |
Nurdiana |
0.15 – 0.25 |
4 |
Vulnerable |
& | ||
0.25 – 0.35 |
3 |
Not Vulnerable |
Risdiyant | ||
>0.35 |
2 |
Least Vulnerable |
o, 2015 | ||
4 |
Land Surface |
>35°C |
5 |
Most Vulnerable |
Setyawan |
Temperature |
30°C - 35°C |
4 |
vulnerable |
, 2005 | |
25°C - 30°C |
3 |
Medium | |||
20°C - 25°C |
2 |
Vulnerability | |||
<20°C |
1 |
Not Vulnerable Least Vulnerable | |||
5 |
Distance |
<100 meter |
5 |
Most Vulnerable |
Erten et |
from Road |
100 – 200 meter |
4 |
vulnerable |
al., 2002 | |
Network |
200 – 300 meter |
3 |
Medium | ||
300 – 400 meter |
2 |
Vulnerability | |||
>400 meter |
1 |
Not Vulnerable Least Vulnerable | |||
6 |
Distance |
<1000 meter |
5 |
Most Vulnerable |
Jaiswal et |
from |
1000 – 2000 meter |
4 |
Vulnerable |
al., 2002 | |
Settlement, |
2000 – 3000 meter |
3 |
Not Vulnerable | ||
Field, and Plantation |
>3000 meter |
2 |
Least Vulnerable |
Source: Data Processing, 2022
Table 3. Forest Fire Vulnerability Classification
Class |
Vulnerability Level |
Range |
0 |
Not Vulnerable |
Settlement Land Use |
1 |
Low Vulnerability |
y < (mean – 0.5 Stdev) |
2 |
Medium Vulnerability |
Mean – 0.5 Stdev < y < mean + 0.5 Stdev |
3 |
High Vulnerability |
Y > mean + 0.5 Stdev |
Source: Data Processing, 2022
The Ministry of Environment and Forestry's historical data on forest and land fires from 2016–2017 and 2019– 2020 are then compared with the three models produced by the SMCA analysis. The three models that were created using the SMCA analysis are compared against each other to validate their applicability. This comparison is carried out as a form of validation of the suitability of the three models that have been generated using the SMCA algorithm. From the validation results, it can be seen which model is the most suitable for use in this study. The descriptive analysis method was carried out on a model of land and forest fire-prone areas that had been made to identify the characteristics of the condition of areas prone to forest and land fires in Situbondo Regency based on the variable aspects of the occurrence of forest and land fires.
Determination of the sample point for statistical tests using the ordinal logistic regression method was carried out using the random sampling method. The number of sample points to be tested is determined using the slovin formula. The equation of the slovin formula used is as follows.
(1)
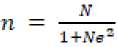
n = Number of Samples
N = Number of Population
e = Margin of Error
The area of Situbondo Regency will be used as a population size and the Margin
of Error that will be used in determining the sample point for statistical tests is 5%. The sample points for the influence test are distributed evenly in the Situbondo Regency area.
The level of influence of the variables on natural and human factors was then assessed statistically using the ordinal logistic regression method (on the validated forest and forest fire vulnerability model). Ordinal logistic regression could be used to determine the relationship between the dependent variable and the independent variable, where the dependent variable in the ordinal logistic regression method is polychotomous and also ordinal (Puspita, 2015). First, the connection between the independent variables (natural and human factors) and the dependent variable was examined using ordinal logistic regression (the level of vulnerability to forest and land fires in Situbondo Regency). The likelihood ratio test and, to a lesser extent, the Wald test were used concurrently to conduct the relationship test. The two-variable relationship tests' equation is as follows.
Statistical analysis using the ordinal logistic regression method was then carried out to determine the level of influence of the variables on natural factors and human factors (on the validated forest and forest fire vulnerability model). Ordinal logistic regression was carried out by first testing the relationship of the independent variables (natural factors and human factors) to the dependent variable (level of vulnerability to forest and land fires in
Situbondo Regency). The relationship test was carried out simultaneously using the likelihood ratio test and also partially using the Wald test. The following is the equation used in the two variable relationship tests.
Likelihood Ratio Test
Hq ■ Pi ~ P2 ~ '
H1 : Setidaknya ada satu
- O
βj ≠0∙J= V,-,?
(2)
Wald Statistic Test
H0 = ¾ = O
Hl = βj ≠ 0; j = 1,2.....p
1f"⅛, where SE^j) = ^var β (3)
The purpose of the regression model appropriateness test was to establish whether there was a major discrepancy between the regression model's anticipated outcomes and the actual results that were observed. If there is no discernible discrepancy between the observed and anticipated results, a regression model might be deemed suitable. The following are the chi-square deviance test's hypotheses and algorithms.
Chi-Square Deviance Test
Hq ■ There is no significant difference between the results of observations and model predictions. The model is declared appropriate.
H1 : There is a significant difference between the results of the observations and the predictions of the model. The model is declared unsuitable.
D = -2∑"^1 ^yij In In {^ + (1 - yij)ln (^}] (4)
To ascertain the changes in the coefficients that occur in the dependent variable (degree of vulnerability) as a result of any changes in a single unit of the dependent variable, the regression model's interpretation is carried out (natural factors and human factors). The value of the odds ratio (ψ) for a variable can be used to interpret its coefficients. If the odds ratio is more than 1, it means that the independent variable and the dependent variable are positively correlated, and if it is lower than 1, it means that the independent variable and the dependent variable are negatively correlated. The following equation is used to calculate the odds ratio value of a dependent variable.
Odds Ratio Equation ψ^' W - eχp (βv+βbW
= exp{exp (βa∣ + βa (a) - β<,i + ft,(⅛))
= exp[βl(a- b)] (5)
The following maps are the result of processing all the thematic map parameters that were later used in the SMCA process.
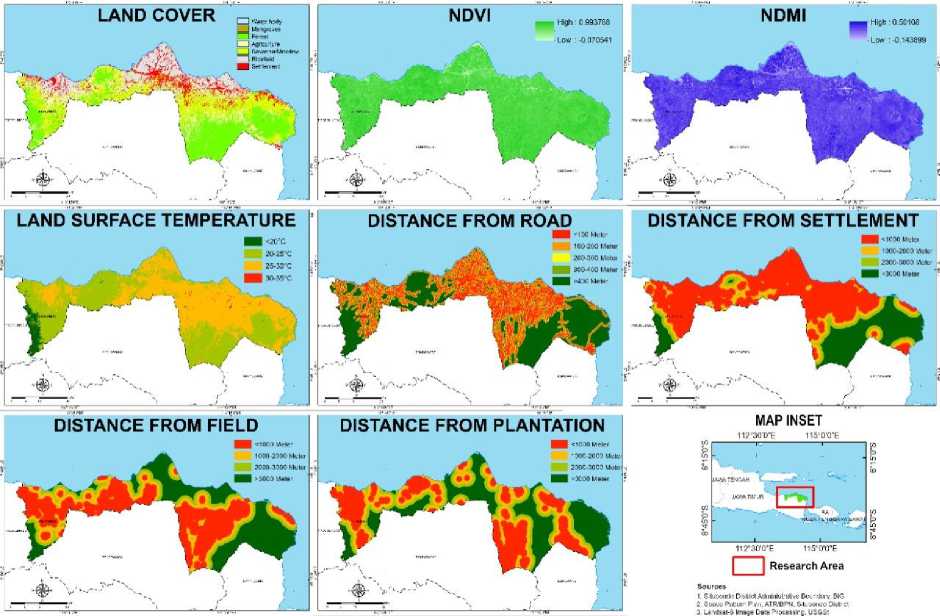
Figure 2.
Thematic Map for Types of Land Cover, NDVI, NDMI, Land Surface Temperature, Distance from Road Network, Settlement, Field, and Plantation
The investigation utilizing the Situbondo method resulted in the generation of three models of the vulnerability to forest and land fires in Situbondo Regency. According to the table of susceptible regions for each model, the first model and the third model
are comparable in that their largest areas of vulnerability are both very close together (666.19 km2 for the first and 611.25 km² for second models) and have modest levels of vulnerability. With an area of 652.66 km2, the majority of the model 2 areas vulnerable to forest and land fires are also areas with a high level of vulnerability (Table 4).
Table 4. Area of the Land and Forest Fire Vulnerability Model in Situbondo Regency
Susceptibility Equation Model 1 Area Equation Model 2 Level (km2) Area (km2) |
Equation Model 3 Area (km2) |
Not Prone 178,65 178,65 Low 666,19 306,46 Vulnerability Medium 452,36 532,12 Vulnerability High 372,69 652,66 Vulnerability |
178,65 611,25 489,90 390,10 |
Source: Data Processing, 2022
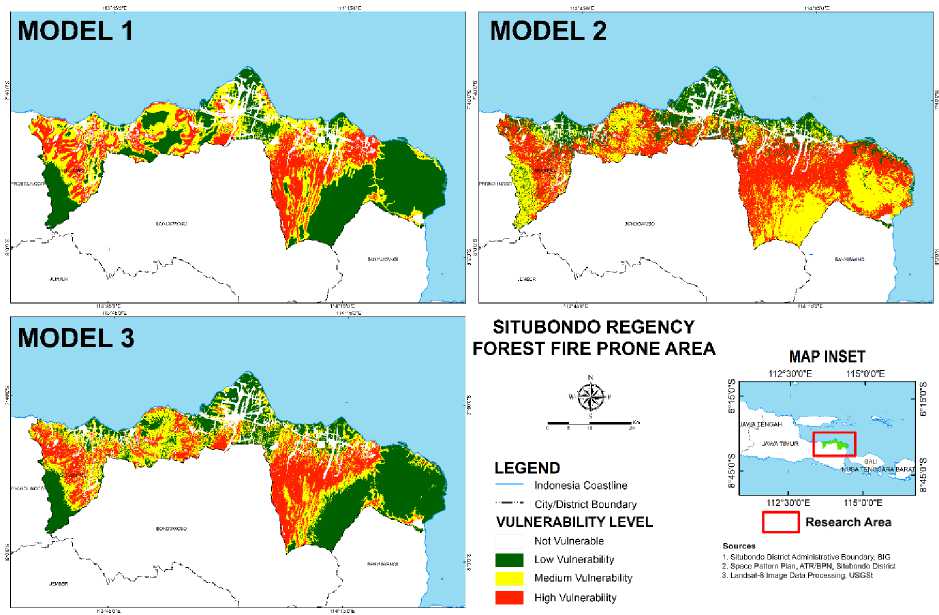
Figure 3.
Model of Forest and Land Fire Vulnerability in Situbondo Regency
The forest and land fires susceptibility model will next be geographically examined using information about forest and land fires obtained from the Ministry of Environment and Forestry (locations of the forest fires in 2016, 2017, 2019, and 2020). The comparison is done by clipping each model with the data from the Ministry of Environment and Forestry. According to those comparisons, The first and third models' burned areas were largely in regions with low vulnerability levels. In contrast, the majority of the burned land in the second model is located in vulnerable locations. According to Table 5, only 1737.29 hectares (16.68%) of regions with a high level of vulnerability were burned in the first model, compared to only 1199.52 hectares
(11.52%) in the second model. exhausted with the third model. In contrast, the second model's vulnerable region, which burns, covers an area of 7037.25 hectares (67.57%).
The equation used in the first model, which gives a higher weight to the human factor, and the third model, which gives equal weight to both types of factors that cause forest and land fires, are considered to have a lower level of conformity when compared to the second model equation, according to the results of the validity test using forest and land fire data sourced from the Ministry of Environment and Forestry. As a result, the second equation will be used to simulate forest and land fire-prone areas.
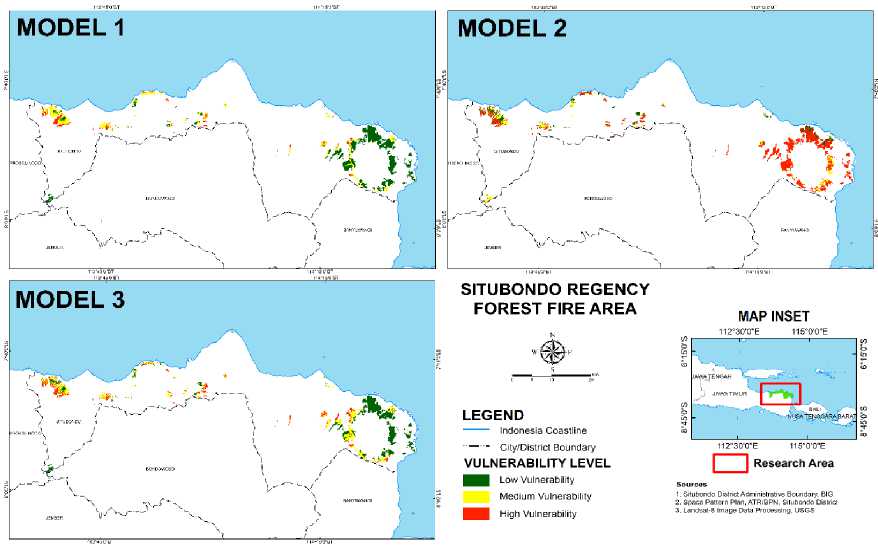
Figure 4.
Model of Forest and Land Fire Vulnerability in Situbondo Regency
Table 5. Area of Burned Area Based on Vulnerability Level
Susceptibi lity Level |
Equation Model 1 |
Equation Model 2 |
Equation Model 3 | |||
Area (Ha) |
% |
Area (km2) |
% |
Area (km2) |
% | |
Low |
6204,58 |
59,57 |
1475,14 |
14,16 |
5130,28 |
49,26 |
Vulnerabil | ||||||
ity | ||||||
Medium Vulnerabil |
3011,11 |
28,91 |
1902,81 |
18,27 |
3547,64 |
34,06 |
ity High Vulnerabil |
1199,52 |
11,52 |
7037,25 |
67,57 |
1737,29 | |
ity |
16,68 | |||||
Total |
10415,21 |
100 |
10415,21 |
100 |
10415,2 |
100 |
Source: Data Processing, 2022
The Situbondo Regency region with a high level of forest and land fire vulnerability has a high index level of vegetation (NDVI > 0.35), low and medium vegetation humidity (NDMI: 0.15-0.35), land surface temperatures in the range of 25 to 30°C, land cover in the form of savanna, dry land agriculture, and a small amount of forest. It also has high
accessibility due to its proximity to the road network (<100 meters), and is only about <1000 meters away from where human activity is taking place. The southern region of Situbondo Regency, which is near plantations and farms, is where regions with a high level of vulnerability are most prevalent.
The Situbondo Regency region with a moderate level of forest and land fire vulnerability is characterized by regions with high levels of greenery (NDVI: >
0.35), medium levels of vegetation humidity (NDMI: 0.25-0.35), and land surface temperatures in the range of 20 to 25°C. This region also has low accessibility due to its long distance from the road network (>400 meters), has a short distance from the plantation (<1000 meters) but has a long distance from settlements and fields (>3000 meters). The western portion of Situbondo Regency, which borders Mount Argapura, the area around Mount Ringgit, the Asembagus District area, as well as the area around the National Park and Mount Baluran, are all examples of locations with a moderate level of forest and land fire vulnerability.
The area of Situbondo Regency with low forest and land fire vulnerability is dominated by areas with a high level of green vegetation (NDVI: >0.35), low and medium-level vegetation humidity (NDMI: 0.25-0.35), soil surface temperature in the range of 20 -30°C (where areas closer to settlements tend to have higher land surface temperatures),
land cover in the form of rice fields, has high accessibility because it is close to the road network (< 100 meters), and is far from plantation sites and fields (>3000 meters), but close to settlements (<1000 meters). The low vulnerability level has the smallest area of the three levels of vulnerability and its distribution can mostly be found along the north coast of Situbondo Regency.
Ordinal logistic regression statistical tests were used to assess the degree of the effects of human and environmental factors on forest vulnerability in the Situbondo Regency area. It is clear from the likelihood ratio test (Table 6) that at least one independent variable influences the level vulnerability variable because the simultaneous test's p-value was 0.000 or less, which is less than the level of significance employed (0.05).
Table 6. Likelihood Ratio Test
Model |
-2 Log Likelihood |
Chi-Square |
df |
Sig. |
Intercept Only |
637,224 | |||
Final |
403,290 |
233,934 |
2 |
0.000 |
Source: Data Processing, 2022
^O : βl~ β2~ "’ = βj ~ Q
H1 : At least there is one βj ≠ 0;j = 1,2, ...,p
According to the Wald test (Table 7), the natural factor variable has a p-value of 0.000 while the human factor variable has a p-value of 0.366. This shows that the H0 hypothesis for the natural factor variable has been rejected, whereas the H0 hypothesis for the human factor variable has been accepted. Only natural elements have a significant role in determining how vulnerable a region is to forest and land fires. It is possible to analyze the coefficients using the odds ratio value, which is displayed in the table above.
The odd ratio value of the natural factor variable that starts forest and land fires is 4.824, whereas the odd ratio value of the human factor variable is 1.051. The odd ratio value of the two variables' interpretation is as follows: Every time the value of natural factors causing forest and land fires is increased by one interval, there is an increasing trend of 4.824 times that an area will have a higher level of vulnerability to forest and land fires. Likewise, every time the value of human factors causing the occurrence of forest and land fires is increased by one interval,
there is an increasing trend of 1.051 that an area will have a higher level of vulnerability to forest and land fires. The lengthening of the gap suggests that the
conditions in a region are becoming more suitable, increasing the region's vulnerability to forest and land fires.
Table 7. Wald Statistic Test
Variable |
Estimator Std. Wald df Sig. Exp(B) (B) Error |
[Vulnerability Level = 1,00] [Vulnerability Level = 2,00] Natural Factor Human Factor |
18,049 1,755 105,815 1 0,000 6.90E+07 21,151 1,923 120,975 1 0,000 1,574 0,145 117,844 1 0,000 4,824 0,050 0,055 0,819 1 0,366 1,051 |
Source: Data Processing, 2022
Table 8. Model Appropriateness Test
Chi-Square |
df |
Sig. | |
Deviance |
405.290 |
452 |
0,952 |
Source: Data Processing, 2022
Hvs ■ There is no significant difference between the results of the observations and the predictions of the model. The model is declared appropriate.
The model appropriateness test had a p-value of 0.952 or above (Table 8), which is considered to be significant (0.05). At a significance level of 0.05 and df 452, the derived Chi-Square value is similarly less than the Chi-Square table. As a result, the H0 hypothesis could not be ruled out, allowing the regression model that was utilized to be judged suitable and having no discernible differences. comparing predicted data with observational outcomes.
The results of statistical studies indicate that natural variables that are vulnerable to forest and land fires have a greater impact on the degree of sensitivity to forest and land fires in the Situbondo Regency area. Due to the vulnerability of Situbondo Regency's natural conditions to forest and forest fire disasters, the higher influence of natural factors that are prone to forest and land fires indicates that forest
H1 : There is a significant difference between the results of the observations and the predictions of the model. The model is declared unsuitable.
and land fires can occur even though the intensity of activity and negligence caused by human activities is not too high. To prevent the start of forest and land fires, all human activities performed near the land and forest regions of Situbondo Regency need to be carefully monitored.
From the results of modeling using the SMCA analysis method, it was found that most areas of Situbondo Regency have a high level of vulnerability to forest and land fires (39.08%). Areas with a high level of vulnerability to forest and land fires are characterized by dry vegetation conditions because most of the area is a savanna area that has low humidity levels and surface temperatures that tend to be higher. The area with a high level of
vulnerability to forest and land fires also has a high level of road accessibility and is not far from the location of human activities. Wetter vegetation has a lower level of accessibility and is farther away from the location of human activities, mostly has a lower level of vulnerability to forest and land fires.
From the results of ordinal logistic regression analysis, it was found that the natural factor variable had a greater level of influence (ψ = 4.824) on the level of forest and forest fire vulnerability compared to the human factor variable (ψ = 1.051). It can be interpreted that the possibility of an area having a higher level of vulnerability to forest and land fires will increase more when there is an increase in the suitability of the area to natural factors that cause forest and land fires compared to an increase in the suitability of the area to human factors. The higher influence of natural factors on forest and land fires indicates that forest and land fires can occur even though the intensity of activity and negligence caused by human activities is not too high due to the vulnerability of Situbondo Regency's natural conditions to forest and forest fire disasters.
Acknowledgments
This study was supported by PUTI Grand by the University of Indonesia NKB-660/UN2.RST/HKP.05.00/2022
References
Amalina, P., Prasetyo, L., & Rushayati, S. 2016. Forest Fire Vulnerability Mapping in Way Kambas National Park. Procedia Environmental Sciences 33: 239–252.
Darwiati, W., & Tuheteru, F. D. 2010. Dampak Kebakaran Hutan Terhadap
Pertumbuhan Vegetasi. Jurnal Tekno Hutan Tanaman 3(1): 27–32.
Erten, E., Kurgun, V., & Musaoglu, N. 2002. Forest Fire Risk Zone Mapping From Satellite Imagery And GIS: A Case Study.
Jaiswal, R., Mukherjee, S., Raju, D., & Saxena, R. 2002. Forest fire risk zone mapping from satellite imagery and GIS. International Journal of Applied Earth Observation and Geoinformation 4: 1–10.
Langmann, & Heil, A. 2004. Release and dispersion of vegetation and peat fire emissions in the atmosphere over Indonesia 1997/1998.
Atmospheric Chemistry and Physics 4: 2145-2160.
Mareta, L., Hidayat, R., Hidayati, R., & Latifah, A. 2020. Pengaruh Faktor Alami dan Antropogenik Terhadap Luas Kebakaran Hutan dan Lahan di Kalimantan. Jurnal Tanah Dan Iklim 43(2): 147-155.
Nurdiana, A., & Risdiyanto, I. 2015.
Indicator Determination of Forest and Land Fires Vulnerability Using Landsat-5 TM Data (Case Study: Jambi Province). Procedia
Environmental Sciences 24: 141
151.
Puspita, E. 2015. Analisis Regresi Logistik Ordinal Pada Faktor Risiko yang Mempengaruhi Tingkat Keparahan Korban Kecelakaan Lalu Lintas di Kota Surabaya. Tugas Akhir. Program Studi S1 Statistika Institut Teknologi Sepuluh
November. Surabaya.
Rasyid, F. 2014. Permasalahan dan Dampak Kebakaran Hutan. Jurnal Lingkar Widyaiswara 1(4): 47–59.
Sabaraji, A. 2005. Identifikasi Zona Rawan Kebakaran Hutan dan Lahan dengan Aplikasi SIG di Kabupaten Kutai Timur. Universitas
Mulawarman. Samarinda
Setyawan D. 2015. Pemodelan Spasial arah penyebaran kebakaran hutan dengan menggunakan penginderaan jauh dan sistem informasi geografis di Taman Nasional Baluran Kabupaten Situbondo Provinsi Jawa Timur Bulan Oktober Tahun 2014. Disertasi. Program Studi Geografi Universitas Muhammadiyah.
Surakarta
Wibowo, A., & Semedi, J. M. 2011.
Model Spasial Dengan SMCE untuk Kesesuaian Kawasan Industri (Studi Kasus Di Kota Serang). Majalah Ilmiah Globe 13(1): 50–59.
Yuliana, Pram & Rahayu, Sri. 2015. Pemetaan Lokasi Kebakaran Berdasarkan Prinsip Segitiga Api Pada Industri Tekstil. Seminar Nasional “Inovasi dalam Desain dan Teknologi.
ECOTROPHIC • 17(1): 1-13 p-ISSN:1907-5626,e-ISSN: 2503-3395
13
Discussion and feedback