Strategic Planning for Green Logistics Implementation in Potato Agro-Industry
on
Jurnal Ilmiah Teknologi Pertanian AGROTECHNO
Volume 6, Nomor 1, April 2021
ISSN: 2503-0523 ■ e-ISSN: 2548-8023
Strategic Planning for Green Logistics Implementation in Potato Agro-Industry
Rindra Yusianto1, Marimin2, Suprihatin3, Hartrisari Hardjomidjojo4
1Graduate Program of Agro-industrial Engineering, Faculty of Agricultural Technology, IPB University (Bogor Agricultural University), PO Box 220 Bogor 16002, Indonesia
-
*email: rindra@staff.dinus.ac.id
-
2,3,4 Department of Agro-industrial Engineering, Faculty of Agricultural Technology, IPB University (Bogor Agricultural University), PO Box 220 Bogor 16002, Indonesia
Abstract
Indonesia is the largest potato producer in Southeast Asia. Annual production averaged 1.09 million tons. Likewise, productivity is still low, ranging from 16.4 - 18.22 tons/ha. The objective of this study was to propose a strategic planning of the Green Logistic Distribution Center (GLDC) in the potato agro-industry using Interpretative Structure Modeling (ISM). A contribution of this research was that we used ISM to analyze the sustainability policy of the green logistics program which includes social, economic, and environmental issues. The result showed that the objectives such as availability of raw materials (1), supplier of potato (3), market place (5), traffic transportation (7), economic factor (8), and environmental issue (9) include variable linkages from the system. Every action on these objectives will result in a successful GLDC program, while the lack of attention to these objectives will lead to program failure. In this case, the driver power is large but has little dependence on the program. While the other sub-elements of purpose are categorized as dependent, this is interpreted more as a result of other objective actions. With two forms of information, ISM and DP-D matrix diagrams, the deepening of the GLDC program is made possible to support strategic planning.
Keywords: green logistics; strategic planning; potato agro-industry; ISM
Abstrak
Indonesia adalah penghasil kentang terbesar di Asia Tenggara. Produksi tahunan rata-rata 1,09 juta ton. Begitu pula produktivitasnya masih rendah, berkisar 16,4 - 18,22 ton / ha. Penelitian ini bertujuan untuk mengusulkan perencanaan strategis Green Logistic Distribution Center (GLDC) pada agroindustri kentang dengan menggunakan Interpretative Structure Modelling (ISM). Kontribusi penelitian ini adalah kami menggunakan ISM untuk menganalisis kebijakan keberlanjutan program logistik hijau yang mencakup masalah sosial, ekonomi, dan lingkungan. Hasil penelitian menunjukkan bahwa tujuan seperti ketersediaan bahan baku (1), pemasok kentang (3), pasar (5), transportasi lalu lintas (7), faktor ekonomi (8), dan masalah lingkungan (9) meliputi keterkaitan variabel. dari sistem. Setiap tindakan terhadap tujuan tersebut akan menghasilkan program GLDC yang berhasil, sedangkan kurangnya perhatian terhadap tujuan tersebut akan menyebabkan kegagalan program. Dalam hal ini, daya penggeraknya besar tetapi memiliki sedikit ketergantungan pada program. Sementara sub-elemen tujuan lainnya dikategorikan sebagai tergantung, ini ditafsirkan lebih sebagai hasil dari tindakan obyektif lainnya. Dengan dua bentuk informasi, diagram matriks ISM dan DP-D, pendalaman program GLDC dimungkinkan untuk mendukung perencanaan strategis.
Kata Kunci: logistik hijau; perencanaan strategis; agroindustri kentang; ISM
INTRODUCTION
Indonesia is the largest potato producer in Southeast Asia. Annual production averaged 1.09 million tons. However, nationally, potato production is relatively no increase, ranging from 1,094,232 - 1,235,180 tons/year. Likewise, productivity is still relatively low, ranging from 15.4-16.4 tons/ha (FAO 2009; Yusianto et al. 2020). Decision making is a human ability that distinguishes them from other beings.
There are many different methods for discussing strategic decision makings, such as genetic algorithms (GA), Analytic Hierarchy Process (AHP), and fuzzy algorithm (Zanjirani et al. 2010; Marimin & Maghfiroh 2011). Each of these methods and approaches is generally only considered a qualitative or quantitative approach. Meanwhile, Interpretative Structure Modeling (ISM) combines the two approaches. Besides, ISM has relatively few uncertainty components but has more benefits when
Rindra Yusianto, Marimin, Suprihatin, Hartrisari Hardjomidjojo. 2021. Strategic Planning for Green Logistics Implementation in Potato Agro-Industry. Jurnal Ilmiah Teknologi Pertanian Agrotechno, Vol. 6, No. 1, 2021. Hal. 9-16
compared to other quantitative methods. ISM is a conceptual model that can change unstructured and unclear ideas into better and structured visualization models (Huang et al. 2012). The model is arranged systematically so that ISM is a good interactional learning process. ISM provides a vision of the relationship between one element and another in a system and can suggest a structure by the interests and explain the influence of each element. ISM can simplify the complex ridge structure into simpler and more organized modules. ISM has been widely implemented in various research midwives including the agro-industry sector (Gupta et al. 2017; Yusianto et al. 2019).
Green environmental issues have attracted the attention of researchers in various fields. Various important factors for implementing the Green Logistics Distribution Center (GLDC) have been identified. Contextual relationships between these factors can be identified (Luthra et al. 2012). Structural models of these factors have been established using the ISM approach (Ajalli et al. 2016).
ISM was introduced by professor J. White Felter Warfield in 1973, its function is to describe complex systems into subsystems (elements), then hierarchical processing of these elements, so that they can show the relationships that occur between elements (Zhai & Li 2017). The ISM feature can be used to describe the complexity of a system into multiple subsystems using practical community experience. With computer tools, in the end, can build a more progressive multi-level structural system model (Huang et al. 2012; Ajalli et al. 2016). Finally, the structure is described in a digraph (Huang et al. 2012). The system structure is mapped with a hierarchical graph direction as shown in Figure 1.
Figure 1. Directional hierarchy graph
In Figure 2, m shows the number of system elements, Si shows row system elements, Sj shows column system elements, and assigns aij to 1 matrix element while there is a relationship between Si and Sj, or sets the matrix element aij to 0. i∈ [1, m], j∈ [1, m].
Figure 2 Adjacency matrix graph
Kulkarni et al. (2018) identified 13 drivers and analyzed to develop ISM-based models. Drivers are identified based on the opinions of experts from the construction industry who have an average of 10 years of work experience in the construction sector. 13 drivers are considered important for supply chain management and green logistics. Based on research conducted by Luthra et al. (2012), there are 13 key factors, including green logistics, green design, environmental performance, and life cycle management. Whereas in the Bag & Anand (2014) study stated that the main driver in GSCM is regulatory pressure and market pressure.
Research Objective, Contribution, and Scope
The objective of this study was to propose a strategic planning for the application of GLDC in the potato agro-industry using ISM. The contribution of this research was that we used ISM to analyze the sustainability policy of the green logistics program which includes social, economic, and environmental issues. The research scope was the potato cultivation and agro-industry in Wonosobo, Central Java, Indonesia.
Research Gap
Agro-industry contributes significantly to increasing the agricultural commodities added value, absorbing labor, and developing regional and national economies. But on the other hand, agro-industry also has the potential to pollute the environment, because this industry produces waste (solid, liquid, and gas) in large quantities and contains high concentrations of organic pollutants (Suprihatin 2009). The issue of environmental strategies has become a critical concern around the world (Vidova et al. 2012; Moreno et al. 2016). Therefore, the interest of organizations to develop policies related to green logistics is increasing dramatically (Pillay & Mbhele 2015). Research by Seroka-Stolka (2014), describes the determinants that influence green logistics as an element of sustainable development. Yusianto et al. (2019) has researched the green logistics model for the potato agro-industry. However, the ISM method was not used in this study. The qualitative analysis of ISM is used to understand the mutual influence between various elements. Research by Mathiyazhagan et al. (2013) has identified which barriers are most dominant for the application of green logistics and these results help the industry to
facilitate the application of green concepts in their logistics. However, their research did not discuss economic factors and the agro-industrial sector.
As customers become more environmentally conscious and governments make tighter regulations, the industry needs to reduce the environmental impact of their logistics chains (Pillay & Mbhele 2015). Jayant & Azhar (2014), states that green logistics is important. The relationship between barriers and to identify barriers that have the most influence on green logistics was analyzed using MICMAC. The structural model using the ISM produces twenty relevant barriers. This is also stated by Bag & Anand (2014), which under market pressure and proactive government policies realize the importance of improving the environmental image. The results of the ISM analysis in Rukmayadi et al. (2016) research, show that the objectives of the green logistic model are reducing the environmental impact of the rubber agro-industry business process, improving the quality of packaging design according to consumer expectations, and being environmentally friendly, and improving institutions. However, in this study, economic factors were not discussed. In research by Zhang et al. (2020), it is explained that the identification of key factors by applying the ISM approach can influence environmentally friendly green logistics. In this study, it is shown that the government's strategic policies, public support, education, and green technology innovation, and environmentally friendly consumer demand have a greater influence than other factors. However, the agro-industry sector was not discussed in this study. Research related to government policies and regulations was researched by Singhal et al. (2018).
The results show that the production process is encouraged to be interconnected and policy-makers must formulate clear policies and information. However, this study did not explain the social and environmental factors.
Green logistics connects social, economic, and environmental aspects. However, in many cases, it is difficult to strike a balance between different needs because of the varied nature of logistics disciplines. In research El-Berishy et al. (2013), exploration of sustainability ideas applied to green logistics to study the interdependence between logistics pillars as well as the concept of integrated corporate sustainability displays social, economic, and environmental challenges. However, the implementation of ISM in green logistics is not discussed in the agro-industry sector.
Most of the previous studies did not discuss the relationship between social, economic, and environmental factors. We think that it is necessary to elaborate on the factors of raw materials, suppliers of potatoes, market place, traffic transportation, economic factors, and environmental issues using ISM in green logistics in the agro-industrial sector.
METHOD
In our proposed research, we focused on policy analysis and strategic planning for the application of GLDC in the potato agro-industry using ISM. We used ISM to analyze the sustainability policy of the green logistics program which includes social, economic, and environmental issues.
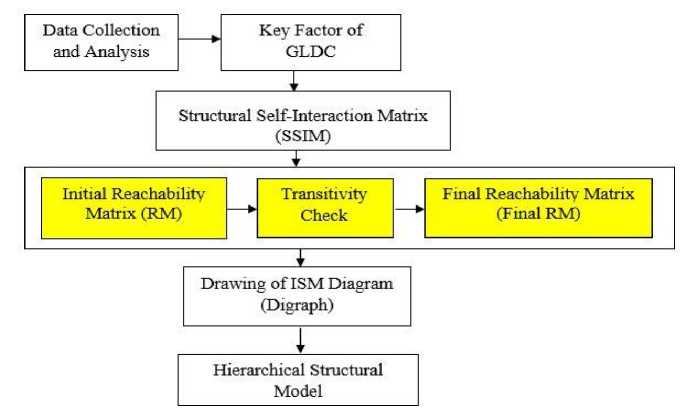
Figure 3. The main working steps of ISM
Research Framework
The ISM is an interpretation as an assessment of a group of experts involved to decide how these elements are related to one another (Hussain et al. 2016). We collect and analyze the elements that affect GLDC. The research framework is shown in Figure 3:
Data Collection and Analysis
We think that social, economic, and environmental factors are very influential on GLDC planning. We break down these factors into the availability of raw materials (S1), characteristics of potato (S2), supplier of potato (S3), topography (S4), market place (S5), logistic infrastructure (S6), traffic transportation (S7), economic factor (S8), environmental issue (S9), organizational factor (S10), technological factor (S11), and human force factor (S12). Where S = {S1, S2, ..., S12}, as shown in Table 1.
Table 1 Key factors of GLDC | ||||
No |
Key Factor |
No |
Key Factor | |
S1 |
Availability raw materials |
of |
S7 |
Traffic transportation |
S2 |
Characteristic potato |
of |
S8 |
Economic Factor |
S3 |
Supplier potato |
of |
S9 |
Environmental Issue |
S4 |
Topography |
S10 |
Organizational factor | |
S5 |
Market place |
S11 |
Technological Factor | |
S6 |
Logistic infrastructure |
S12 |
Human force factor |
Structural Self-Interaction Matrix (SSIM)
Based on previous research, the elements that influence GLDC are identified and validated by experts. We involve 5 experts from the agro-industry and academia. The 12 most influential elements were then resolved and analyzed.
Table 2 The SSIM Matrix
S12 Sll SlO S9 S8 S7 S6 S5 S4 S3 S2 Sl
Sl V A A V V V V V V A O
S2 V A AAAAXAAA
S3 V A AOAVOVO
S4 V A AOOOVO
S5 V A AVVAO
S6 V A AOOA
S7 VAAVV
S8 VAAA
S9 V A A
SIO V A
Sll V
S12
We facilitate experts to coordinate against different opinions. This is intended to reduce bias that may occur. We provide SSIM sheets without notation to each expert, to compile contextual relationships between different GLDCs. The results are then discussed and a final matrix is created that reflects expert consensus on each assessment. To analyze and develop the SSIM of the 12 factors that influence GLDC, we use the following four symbols.
-
• V - GLDC i will help achieve GLDC j
-
• A - GLDC j will help achieve GLDC i
-
• X - GLDC i and j will help to achieve each
other
O - GLDC i and j are not related.
Initial Reachability Matrix
The SSIM is then converted the symbols V, A, X, and O with the binary matrix 1 and 0. The converting of the four symbols with 1 and 0. If there is a possible transitive link, then there is a need to check transitivity.
RESULTS AND DISCUSSIONS
In this section, we discussed the final reachability matrix, drawing of the ISM diagram, establishing a hierarchical structure model, research limitation, and manager implication.
Table 3 Initial reachability matrix
Sl |
S2 |
S3 |
S4 |
S5 |
S6 |
S7 |
S8 |
S9 |
SlO |
Sll |
S12 | |
Sl |
1 |
0 |
0 |
1 |
1 |
1 |
1 |
1 |
1 |
0 |
0 |
1 |
S2 |
0 |
1 |
0 |
0 |
0 |
1 |
0 |
0 |
0 |
0 |
0 |
1 |
S3 |
1 |
1 |
1 |
0 |
1 |
0 |
1 |
0 |
0 |
0 |
0 |
1 |
S4 |
0 |
1 |
0 |
1 |
0 |
1 |
0 |
0 |
0 |
0 |
0 |
1 |
S5 |
0 |
1 |
0 |
0 |
1 |
0 |
0 |
1 |
1 |
0 |
0 |
1 |
S6 |
0 |
1 |
0 |
0 |
0 |
1 |
0 |
0 |
0 |
0 |
0 |
1 |
S7 |
0 |
1 |
0 |
0 |
1 |
1 |
1 |
1 |
1 |
0 |
0 |
1 |
S8 |
0 |
1 |
1 |
0 |
0 |
0 |
0 |
1 |
0 |
0 |
0 |
1 |
S9 |
0 |
1 |
0 |
0 |
0 |
0 |
0 |
1 |
1 |
0 |
0 |
1 |
SlO |
1 |
1 |
1 |
1 |
1 |
1 |
1 |
1 |
1 |
1 |
0 |
1 |
Sll |
1 |
1 |
1 |
1 |
1 |
1 |
1 |
1 |
1 |
1 |
1 |
1 |
S12 |
0 |
0 |
0 |
0 |
0 |
0 |
0 |
0 |
0 |
0 |
0 |
1 |
Final Reachability Matrix
After obtaining the RM matrix and checking the transitivity, the next step is to compile the Final RM.
Table 4 Final reachability matrix
Sl |
S2 |
S3 |
S4 |
S5 |
S6 |
S7 |
S8 |
S9 |
SlO |
Sll |
S12 |
DP |
R | |
Sl |
1 |
1 |
1 |
1 |
1 |
1 |
1 |
1 |
1 |
0 |
0 |
1 |
10 |
3 |
S2 |
0 |
1 |
0 |
0 |
0 |
1 |
0 |
0 |
0 |
0 |
0 |
1 |
3 |
5 |
S3 |
1 |
1 |
1 |
1 |
1 |
1 |
1 |
1 |
1 |
0 |
0 |
1 |
10 |
3 |
S4 |
0 |
1 |
0 |
1 |
0 |
1 |
0 |
0 |
0 |
0 |
0 |
1 |
4 |
4 |
S5 |
1 |
1 |
1 |
1 |
1 |
1 |
1 |
1 |
1 |
0 |
0 |
1 |
10 |
3 |
S6 |
0 |
1 |
0 |
0 |
0 |
1 |
0 |
0 |
0 |
0 |
0 |
1 |
3 |
5 |
S7 |
1 |
1 |
1 |
1 |
1 |
1 |
1 |
1 |
1 |
0 |
0 |
1 |
10 |
3 |
S8 |
1 |
1 |
1 |
1 |
1 |
1 |
1 |
1 |
1 |
0 |
0 |
1 |
10 |
3 |
S9 |
1 |
1 |
1 |
1 |
1 |
1 |
1 |
1 |
1 |
0 |
0 |
1 |
10 |
3 |
SlO |
1 |
1 |
1 |
1 |
1 |
1 |
1 |
1 |
1 |
1 |
0 |
1 |
11 |
2 |
Sll |
1 |
1 |
1 |
1 |
1 |
1 |
1 |
1 |
1 |
1 |
1 |
1 |
12 |
1 |
S12 |
0 |
0 |
0 |
0 |
0 |
0 |
0 |
0 |
0 |
0 |
0 |
1 |
1 |
6 |
D |
8 |
11 |
8 |
9 |
8 |
H |
8 |
8 |
8 |
2 |
1 |
12 | ||
L |
4 |
2 |
4 |
3 |
4 |
2 |
4 |
4 |
4 |
5 |
6 |
1 |
DP: Driver Power D: Dependence L: Level R: Ranking
Drawing of ISM Diagram
The results of the analysis are presented in the form of a diagram (digraph). Digraph consists of 4 quadrants, where quadrant 1) autonomous variables, namely variables that have nothing to do with the system, have a small thrust, and the level of dependence is low; 2) the dependent variable, namely
the variable with a small thrust and a high level of dependence; 3) linkage variable, which is a variable with a large driving force and a high level of dependence; and 4) independent variables, namely variables that have high driving power and low dependence. We compiled the partition level using the final RM matrix.
Table 5 Level of GLDC | |
Rauk |
Level Sector Diiver Power Dependence |
Sl 3 S2 5 S3 3 S4 4 S5 3 S6 5 S7 3 S8 3 S9 3 SlO 2 Sil 1 S12 6 |
4 3 10 8 2 2 3 11 4 3 10 8 3 2 4 9 4 3 10 8 2 2 3 11 4 3 10 8 4 3 10 8 4 3 10 8
12 1 12 |
Based on Figure 4, it can be seen that the sub-element that has the highest driving force is the streamlining of technological factors. Furthermore, these elements will affect the supporting elements of technological factor, increasing the organizational factor, increasing availability of raw materials, supplier of potato, market place, traffic transportation, economic factor, and environmental issue.
The result showed that the objectives such as availability of raw materials (1), supplier of potato
(3), market place (5), traffic transportation (7), economic factor (8), and environmental issue (9) include variable linkages from the system. Every action on these objectives will result in a successful GLDC program of potato agriculture in Central Java, while the lack of attention to these objectives will lead to program failure. Further analysis in sector IV (independent), states that objectives such as organization factor (10), and technological factor (11) are included as independent variables.
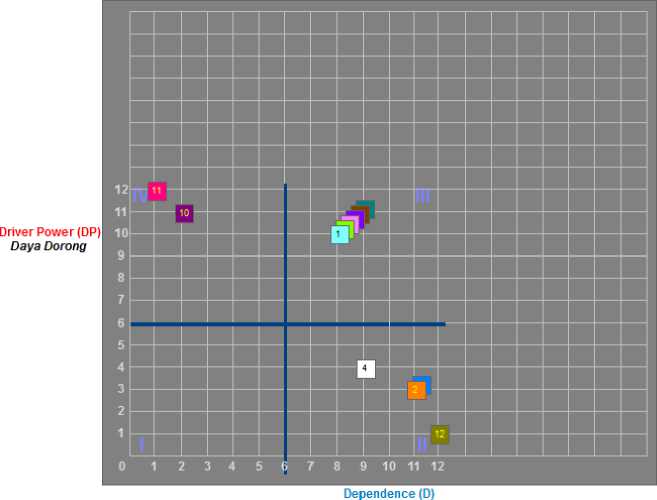
Ketergantungan
Figure 4 Digraph of GLDC
Legend
[^i~∣ Availabilityofrawmaterials
-
□ Characteristic of potato
-
3] Supplierofpotato
∏ Topography
[i^∣ Ivlarketplace
Il Logistic infrastructure
n Traffic transportation
B EconomicFactor
B Environmental Issue
B Organizational factor
B Technological Factor
B Human force factor
Hierarchical Structural Model
Based on the classification of sub-elements into four sectors of the power-dependent driver sub-elements that are included in the dependent sector are subelements of providing information on the human force factor. The sub-element depends on the other sub-elements. Whereas the supporting elements of as availability of raw materials (1), supplier of potato (3), market place (5), traffic transportation (7), economic factor (8), and environmental issue (9) are in the third linkage sector sub these elements need to be carefully studied, while the streamlining technological factor (11) element is in the independent sector where the sub-elements are not influenced by other sub-elements. Based on the high level of thrust and driver power, the streamlining of technological factors is a key element in the subobjective elements of tight agro-industry development.
Research Limitations
The results of this study are the proposed planning strategies for implementing GLDC in the potato
agro-industry using ISM. We only discuss the elements of the objectives in the ISM, we do not discuss the other elements of the ISM. The sustainability of the green logistics program which includes social, economic, and environmental issues is discussed in the 12 GLDM factors in this study, but we do not classify in detail these problems.
Managerial Implications
The key related factors affecting program success have been discussed in detail in this study. Policy analysis and planning strategies for implementing GLDC in the potato agro-industry can assist decision-makers in developing sustainable green logistics programs.
CONCLUSION
By calculating the Power Driver (DP) and Dependence (D) of each sub-element, the DP-D matrix can be arranged by placing it on each ordinate (x, y). Based on Figure 4, it can be seen that the
objectives such as availability of raw materials (1), supplier of potato (3), marketplace (5), traffic transportation (7), economic factor (8), and environmental issue (9) include variable linkages from the system. Every action on these objectives will result in a successful GLDC program of potato agriculture in Central Java, while the lack of attention to these objectives will lead to program failure. Further analysis in sector IV (independent), states that objectives such as organization factor (10), and
technological factor (11) are included as independent variables. In this case, the driver power is large but has little dependence on the program. While the other sub-elements of purpose are categorized as dependent, this is interpreted more as a result of other objective actions. With two forms of information (ISM and DP-D matrix diagrams) the deepening of the GLDC program of potato agriculture in Central Java is made possible to support strategic planning.
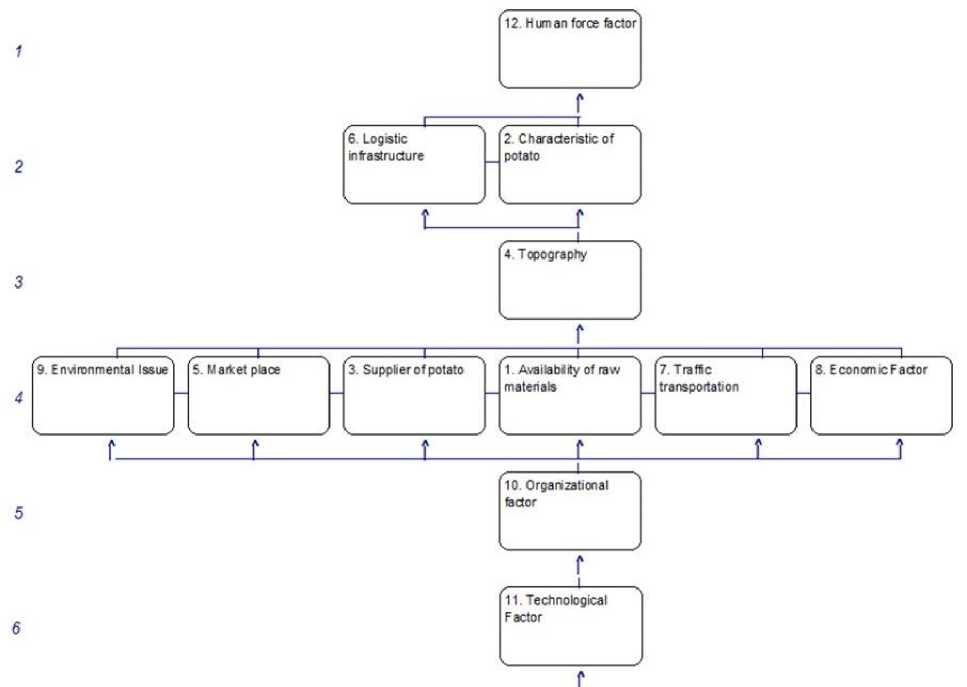
Figure 5 GLDC Hierarchical structure model
Recommendations
Sustainable development related to social, economic, and environmental factors is an interesting issue, including in the agro-industry sector. The final recommendation is given to the Central Java provincial government where the results of the policy analysis and strategic planning for green logistics implementation in potato agro-industry using ISM are expected to be useful for promoting the sustainable development of green logistics agroindustry.
References
Ajalli M, Asgharizadeh E, Jannatifar H, Abbasi A.
2016. A Fuzzy ISM Approach for Analyzing the Implementation Obstacles of Electronic Government in. Proceedings of International
Conference on Science, Technology, Humanities and Business Management, (7):p.29–30.
Bag S, Anand N. 2014. Modeling Green Supply Chain Management framework using ISM and MICMAC analysis. African Journal of Business Management 8(22):p.1053–1065.
El-Berishy N, Rügge I, Scholz-Reiter B. 2013. The interrelation between sustainability and green logistics, IFAC.
FAO. 2009. New Light on a Hidden Treasure: End of Year Review
Gupta A, Singh RK, Suri P. 2017. Modeling the barriers of Indian logistics service providers : ISM approach. 9(1):p.3–4.
Huang L, Yu J, Huang X. 2012. Modeling agricultural logistics distribution center
location based on ISM. Journal of Software 7(3):p.638–643.
Hussain M, Awasthi A, Tiwari MK. 2016. Interpretive structural modeling-analytic network process integrated framework for evaluating sustainable supply chain management alternatives. Applied
Mathematical Modelling 40(5–6):p.3671– 3687.
Jayant A, Azhar M. 2014. Analysis of the barriers for implementing green supply chain management (GSCM) Practices: An Interpretive Structural Modeling (ISM) Approach. Procedia Engineering 97(1):p.2157–2166.
Kulkarni PB, Ravi K, Patil SB. 2018a. Interpretive Structural Modeling (ISM) for Implementation of Green Supply Chain Management in Construction Sector within Maharashtra . International Research Journal of Engineering and Technology (IRJET):p.2460– 2472.
Kulkarni PB, Ravi K, Patil SB. 2018b. Interpretive Structural Modeling (ISM) for Implementation of Green Supply Chain Management in Construction Sector within Maharashtra . :p.2460–2472.
Luthra S, Garg D, Kumar S, Haleem A. 2012. Implementation of The Green Supply Chain Management in Manufaturing Industry In India Using Interpretive Structural Modeling Technique. BPR Technologia: A Journal of Science, Technology & Management 1 1(1):p.1–17.
Marimin, Maghfiroh N. 2011. Aplikasi Teknik Pengambilan Keputusan dalam Manajemen Rantai Pasok
Mathiyazhagan K, Govindan K, NoorulHaq A, Geng Y. 2013. An ISM approach for the barrier analysis in implementing green supply chain management. Journal of Cleaner Production 47(May):p.283–297.
Moreno P, Lozano S, Adenso-Diaz B, Garcia-Carbajal S. 2016. Influence of the environmental impact of logistics operations on the centralization strategy. In International Conference on Industrial Engineering and Systems Management, IEEE IESM 2015. pp. 556–560.
Pillay K, Mbhele TP. 2015. The Challenges of Green Logistics in the Durban Road Freight Industry. Environmental Economics 6(1):p.64–73.
Rukmayadi D, Marimin, Haris U, Yani M. 2016. Rubber agro-industry green logistic conceptual model. International Journal of Supply Chain Management 5(3):p.192–204.
Seroka-Stolka O. 2014. The Development of Green Logistics for Implementation Sustainable
Development Strategy in Companies. Procedia - Social and Behavioral Sciences 151(10):p.302–309.
Singhal D, Tripathy S, Jena SK, Nayak KK, Dash A. 2018. Interpretive structural modelling (ISM) of obstacles hindering the remanufacturing practices in India. Procedia Manufacturing 20:p.452–457.
Suprihatin. 2009. Manfaat Ekologis dan Finansial Pemanfaatan Limbah Cair Agroindustri sebagai Bahan Baku dalam Produksi Biogas untuk Mereduksi Gas Rumah Kaca. Journal Agromet 23(9):p.101–111.
Vidova H, Babcanova D, Witkowski K, Saniuk S. 2012. Logistics and Its Environmental Impacts. In International Scientific Conference Business and Management. pp. 1007–1014.
Yusianto R., Marimin, Suprihatin, Hardjomidjojo H. 2019. Green Logistics Approach in Bioethanol Conversion from Potato Starch in Central Java. IOP Conference Series: Materials Science and Engineering 598(1):p.1–9.
Yusianto R., Sundana S, Marimin, Djatna T. 2020. Method and mapping of trust and trustworthiness in agroindustry logistic and supply chain: A systematic review.
International Journal of Supply Chain Management 9(1).
Yusianto Rindra, Marimin, Suprihatin,
Hardjomodjojo H. 2020. Spatial Analysis for Crop Land Suitability Evaluation: A Case Study of Potatoes Cultivation in Wonosobo, Indonesia. International Seminar on Application for Technology of Information and Communication (iSemantic) 1(1):p.313–319.
Zanjirani R, Steadieseifi M, Asgari N. 2010. Multiple criteria facility location problems : A survey. Applied Mathematical Modelling
34(7):p.1689–1709.
Zhai Y, Li Y. 2017. The Research on Influencing Factors of Medical Logistics Cost Based on ISM Model. MATEC Web of Conferences 100:p.02030.
Zhang M, Sun M, Bi D, Liu T. 2020. Green Logistics Development Decision-Making: Factor
Identification and Hierarchical Framework Construction. IEEE Access 8(1):p.127897– 127912.
16
Discussion and feedback