EBLUP-SMALL AREA ESTIMATION METHOD FOR PER CAPITA EXPENDITURES IN BALI
on
E-Jurnal Matematika Vol. 11(1), Januari 2022, pp. 58-63
DOI: https://doi.org/10.24843/MTK.2022.v11.i01.p361
ISSN: 2303-1751
EBLUP-SMALL AREA ESTIMATION METHOD FOR PER CAPITA EXPENDITURES IN BALI
Luthfatul Amaliana1§, Made Laras Setyana Dewi2
1Jurusan Statistika, Fakultas MIPA – Universitas Brawijaya [Email: [email protected]] 2Jurusan Statistika, Fakultas MIPA – Universitas Brawijaya [Email: [email protected]] §Corresponding Author
ABSTRACT
Small Area Estimation (SAE) is a statistical technique for estimating the parameters of a subpopulation with a small sample size. SAE aims to improve the accuracy of parameter estimation, with indirect estimation. This study aims to determine the best method between empirical best linear unbiased prediction (EBLUP) and spatial EBLUP methods (with a queen contiguity weighted matrix) in estimating per capita expenditure per sub-district in Bali. The results of this study indicate that the best SAE method in estimating per capita expenditure per sub-district in Bali is the EBLUP method with the smaller mean squared error. The EBLUP estimation results are significantly influenced by three variables, namely the population, public primary schools, and families using PLN. The sub-district with the highest per capita expenditure in Bali is Denpasar Selatan sub-district. Meanwhile, the sub-district with the lowest per capita expenditure was Abang sub-district. Since the EBLUP model is better than SEBLUP model, this indicates that per capita expenditure per sub-district in Bali is not influenced by its neighbors.
Keywords: Per Capita Expenditures, Bali, EBLUP, SEBLUP, Small Areas Estimation
Survei sosial ekonomi nasional (SUSENAS) conducted by Badan Pusat Statistik (BPS) is a survey activity to collect information/data on population, health, education, family planning, housing, consumption, and expenditure. This survey is designed to have three datasets (modules) which held every three years. These modules are the household consumption/expenditure module, the social, cultural and educational module, and the housing and health module (BPS, 2013). The poverty in an area is a representation of the community welfare which can be seen on the expenditure module.
According to BPS (2019), measurement of poverty is carried out using the concept of the ability to meet basic needs. This approach views poverty as an economic inability to meet basic food and non-food needs as measured in terms of expenditure. Therefore, the poor are those who have an average expenditure per capita per month below the poverty line. One of the government's efforts to overcome the
poverty problem is to predict poor areas down to the level of small areas such as districts/cities, sub-districts and villages. The sample system in a population survey in a small area causes a limited number of survey objects. The limitation means the direct estimates cannot produce an accurate estimate. In order to produce better predictions, the indirect estimation method can be used in a small area (Rao, 2003).
Small area estimation (SAE) is an indirect estimation that utilizes information from the surrounding area related to parameters. This method conducted as an effort to suppress a large variety of direct estimates. This method is used as an attempt to suppress a large variety of direct estimates. One of the SAE methods that can be used in estimating small areas is the empirical best linear unbiased prediction (EBLUP) (Sidauruk, et al., 2013). The estimation using the EBLUP method on continuous data needs to be evaluated. This is because the estimator obtained in a small area
is a biased estimator with a minimum variance. The purpose of estimating the EBLUP method is to obtain an efficient estimator. Estimator accuracy can be obtained by measuring the mean square error (MSE). The smaller the MSE, the more accurate an estimator will be.
The indirect estimator of small area is obtained by weighting the random variable of an area to increase the effectiveness of the sample size and minimize the variance. Heterogeneity of an area can be influenced by the surrounding area. This heterogeneity is summarized in spatial effects of the area which can be contained in random effect variable. The EBLUP method that accommodates spatial effects in the model is known as the spatial EBLUP method (SEBLUP) (Pratesi and Salvati, 2008).
Mutualage (2012) conducted a study which show that the SEBLUP method is better than EBLUP for estimating per capita expenditure of villages in Jember District. While Sidauruk, et al. (2013) showed that the characteristics of the parameter β obtained by simulation data on SAE are biased estimators. However, the bias is very small with minimum variance, so that the EBLUP parameter estimates are close to the actual parameters. Another study of Nusrang, et al. (2017) supports Matualage's results which using simulation data showed that the violation of homoscedasticity assumption of random effect of error caused the EBLUP estimator to be in-optimum and biased, so the SEBLUP estimator would give a better prediction than the EBLUP estimator.
This study was conducted to estimate poverty based on per capita expenditure per sub-district in Bali by using the EBLUP and SEBLUP methods (with queen contiguity spatial weighted matrix). Bali is the province with the second lowest poverty rate in Indonesia. This research is expected to provide information about the welfare of the community which is reflected in per capita expenditure per district in Bali. In addition, the EBLUP model that is produced can also provide information on factors that are significant in determining per capita expenditure per sub-district in Bali.
The data used in this study is secondary data from BPS in 2018. The response variable
used in this study is per capita expenditure per sub-district which comes from Bali dalam Angka 2018. Meanwhile, the predictor variables including the population, public health centers, public primary schools, and families using PLN (Perusahaan Listrik Negara). The unit population in this study in sub-districts in Bali Province. The operational definition of each variable in this study is summarized in Table 1.
Table 1. Operational Definition of Variables | |
No. Variables |
Variable Definitions |
1. Per capita |
Average per capita |
expenditure |
expenditure per month |
(Vi) |
(share of monthly household expenditure (Rp) with the number of household members (people)) at the subdistrict level in Bali (in Rupiah) |
2. Population |
Total population per subdistrict in Bali (people) |
3. Public health |
Number of health centers |
centers |
per sub-district in Bali |
4. Public |
Number of public primary |
primary |
schools per sub-district in |
schools |
Bali |
5. Families |
Number of families using |
using PLN |
PLN per sub-district in Bali |
The steps of analysis in this study can be described as follows:
-
a. Preparing data as direct estimator. The direct estimator is per capita expenditure per sub-districts in Bali.
-
b. Preparing data as predictor variables. These variables are the population, public health centers, public primary schools, and families using PLN.
-
c. Preparing a spatial weighted matrix (W). This matrix only contains 0 and 1 values. If two sub-districts are side by side and intersect, then it is represented by the value wij = 1, while wij = 0 otherwise.
-
d. Estimating the parameter of EBLUP and SEBLUP models (βi) by using
generalized least square (GLS) method.
-
e. Estimating the random effects (Vj) of EBLUP and SEBLUP models by using GLS method.
-
f. Estimating per capita expenditure based on the EBLUP and SEBLUP models for each sub-district in Bali.
-
g. Estimating the MSE of EBLUP and SEBLUP for each sub-district in Bali.
DOI: https://doi.org/10.24843/MTK.2022.v11.i01.p361
-
h. Comparing the MSE of EBLUP and SEBLUP models.
Descriptive analysis is used to determine an overview of Bali data in this study, especially about per capita expenditure. The following descriptive analysis for per capita expenditure is presented in Table 2.
Table 2. Descriptive Statistics
Variable |
Min |
Max |
Average |
Per capita expenditure |
104,257 (Marga) |
6,476,787 (Denpasar Selatan) |
1,403,789 |
Table 2 shows that the lowest per capita expenditure is Rp. 104,257 namely Marga Subdistrict. Meanwhile, the subdistrict with the highest per capita expenditure was Denpasar Selatan Sub-district, which was Rp. 6,476,787.
The parameters (βj) and the random effects (Vj) of EBLUP model need to be estimated before estimating per capita expenditure per sub-district in Bali. Because of the difference units in each variable, it is necessary to standardize data. According to these estimators of βi and Vi, the EBLUP model can be written as (1).
⅛ = χjβ^+ Vj ^
-
= ×ιiβι + x2iβ2 + χ3iβ3 + x4i∕^4 + Vi
-
= 105,148.687 x1i + 11.548 x2i +
-
3 7.373 x3i + 14,733,142 x4i + Vi (1)
The estimation results according to EBLUP model in (1) is summarized in Table 3.
Table 3. Per Capita Expenditure (EBLUP Model)
Subdistrict |
EBLUP Estimator |
Subdistrict |
EBLUP Estimator |
Abiansemal |
2,321,936.84 |
Tegallalang |
998,436.67 |
Kuta |
266,218.10 |
Ubud |
962,849.27 |
Kuta Selatan |
3,118,038.68 |
Jembrana |
341,284.48 |
Kuta Utara |
2,313,222.30 |
Melaya |
741,689.80 |
Mengwi |
3,055,738.75 |
Mendoyo |
831,176.54 |
Petang |
875,073.27 |
Negara |
1,240,263.42 |
Bangli |
1,650,999.04 |
Pekutatan |
583,939.04 |
Kintamani |
2,935,934.20 |
Abang |
221,932.16 |
Susut |
1,339,721.96 |
Bebandem |
724,849.85 |
Tembuku |
975,019.49 |
Karangase |
935,533.37 |
m |
Subdistrict |
EBLUP Estimator |
Subdistrict |
EBLUP Estimator |
Banjar |
1,018,800.59 |
Kubu |
756,423.30 |
Buleleng |
4,281,029.59 |
Manggis |
722,404.21 |
Busungbiu |
502,282.07 |
Rendang |
708,230.12 |
Gerokgak |
1,837,704.31 |
Selat |
696,221.52 |
Kubutambah an |
971,817.08 |
Sidemen |
516,300.02 |
Sawan |
1,628,527.15 |
Banjarangkan |
762,227.76 |
Seririt |
1,889,214.28 |
Dawan |
769,065.49 |
Sukasada |
1,371,585.21 |
Klungkung |
1,408,766.29 |
Tejakula |
907,178.57 |
Nusa Penida |
416,153.77 |
Denpasar Barat |
5,337,337.53 |
Baturiti |
598,907.80 |
Denpasar Selatan |
6,006,767.68 |
Kediri |
1,381,440.27 |
Denpasar Timur |
3,037,163.89 |
Kerambitan |
612,911.72 |
Blahbatuh |
1,066,433.44 |
Marga |
262,339.42 |
Gianyar |
1,505,502.85 |
Penebel |
388,734.31 |
Payangan |
917,244.08 |
Pupuan |
929,970.16 |
Sukawati |
3,128,579.90 |
Selemadeg |
429,519.16 |
Tampaksiring |
1,015,423.75 |
Tabanan |
646,380.00 |
Table 3 shows that the highest per capita expenditure in Bali is Rp. 6,006,747.77, namely South Denpasar Sub-district. Meanwhile, the lowest per capita expenditure is Rp. 221,932,16, namely Abang Sub-district. Testing the error normality assumption is carried out using the Anderson Darling test (α = 5%) with the null hypothesis: e ~ N(0, σf) againts the alternative hypothesis: e ≠N(0,σ2). The result presented in Table 4.
Table 4. Normality Assumption of Error EBLUP Model
Test Statistics |
p-value |
Decision |
0.2407 |
0.7634 |
Accept H0 |
Table 5. Significance of Model EBLUP Parameters | |||
Variable |
∙. β |
p-value |
Decision |
Population |
0.69813 |
3.89×10-8 |
Reject H0 |
Public health centres Public |
0.09688 |
2.36×10-1 |
Accept H0 |
primary schools |
-0.26566 |
1.69×10-2 |
Reject H0 |
Families using PLN |
0.29973 |
1.13×10-2 |
Reject H0 |
According to Table 4, it can be concluded that the assumption of normality of error is
fulfilled, in other word, the error of EBLUP model spreads normally. Whereas Table 5 summarized the results of testing the significance of the EBLUP model parameters using the t-test (a = 5%). The null hypothesis of this test is: βi = 0 againts the alternative hypothesis: βi ≠ 0.
Table 5 shows that the total population, number of public primary schools, and number of families using PLN variables have a significant effect on per capita expenditure in Bali based on the EBLUP-SAE model.
Spatial EBLUP model is a development of EBLUP model that accommodate spatial effect in the model. In the SEBLUP model, a spatial weighting matrix is needed to represent the spatial heterogeneity of each sub-district. The spatial weighting matrix in this study is the queen contiguity matrix as written in (2).
0 |
0 |
0 |
0 |
1/8 |
1/8 |
0 |
0 |
— 0 | ||
0 |
0 |
1/3 |
1/3 |
0 |
0 |
0 |
0 |
- 0 | ||
0 |
1 |
0 |
0 |
0 |
0 |
0 |
0 |
— 0 | ||
0 |
1/3 |
0 |
0 |
1/3 |
0 |
0 |
0 |
- 0 | ||
W = |
1/5 1/8 |
0 0 |
0 0 |
1/5 0 |
0 0 |
0 0 |
0 0 |
0 1/8 |
- 0 - 0 |
(2) |
0 |
0 |
0 |
0 |
0 |
0 |
0 |
1/7 |
-0 | ||
0 ⋮ |
0 |
0 |
0 |
0 |
1/9 |
1/9 |
0 |
-0 | ||
0 |
0 |
0 |
0 |
0 |
0 |
0 |
0 |
- 0. |
The SEBLUP model can be written as in (3).
y = Xβ + Zv + e (3)
where v = (I - pW)-1u. The SEBLUP in (3) can be rewritten as in (4).
y = Xβ + Z((I - PWy1U) + e (4)
The estimation of per capita expenditure per district using the SEBLUP method is carried out by first estimating the SEBLUP parameter (βi), random effect (v) and autoregression coefficient (p) in the model. The estimation of random effect can be obtained by (4), where p = 0.18107 and W is the weighting matrix. Based on the results of the parameter, random effect, and autoregression coefficient of the SEBLUP model, as well as the previous weighting matrix, the SEBLUP model is obtained as written in (5).
yιs = χιβ + ⅛
= X1iβ1 + X2iβ2 + X3iβ3 + X4iβ4 + Vi
= 105.162,321 X1i + 11,567 X2i +
37,432 X3i + 14.723,051 X4i + vis (5)
The estimation results of SEBLUP model in (5) is presented in Table 6.
Table 6. Per Capita Expenditure (EBLUP Model)
Sub-district |
SEBLUP Estimator |
Sub-district |
SEBLUP Estimator |
Abiansemal |
2,353,593.08 |
Tegallalang |
991,349.75 |
Kuta |
274,613.08 |
Ubud |
992,184.90 |
Kuta Selatan |
3,185,553.82 |
Jembrana |
341,042.58 |
Kuta Utara |
2,350,961.26 |
Melaya |
698,124.94 |
Mengwi |
3,071,225.43 |
Mendoyo |
798,673.75 |
Petang |
844,348.70 |
Negara |
1,173,692.51 |
Bangli |
1,631,276.25 |
Pekutatan |
574,391.14 |
Kintamani |
2,951,015.13 |
Abang |
219,787.57 |
Susut |
1,292,811.97 |
Bebandem |
727,355.52 |
Tembuku |
987,930.60 |
Karangasem |
974,942.36 |
Banjar |
1,013,212.11 |
Kubu |
782,559.55 |
Buleleng |
4,278,713.23 |
Manggis |
741,793.61 |
Busungbiu |
501,019.12 |
Rendang |
710,933.05 |
Gerokgak |
1,746,660.94 |
Selat |
710,357.22 |
Kubutambahan |
968,205.47 |
Sidemen |
510,994.89 |
Sawan |
1,610,255.39 |
Banjarangkan |
754,409.17 |
Seririt |
1,898,166.46 |
Dawan |
772,784.20 |
Sukasada |
1,359,359.06 |
Klungkung |
1,423,095.71 |
Tejakula |
894,481.93 |
Nusa Penida |
430,679.82 |
Denpasar Barat |
5,370,533.76 |
Baturiti |
602,569.04 |
Denpasar Selatan |
6,050,927.72 |
Kediri |
1,392,496.16 |
Denpasar Timur |
3,096,984.86 |
Kerambitan |
603,108.84 |
Blahbatuh |
1,071,081.56 |
Marga |
248,671.99 |
Gianyar |
1,504,283.46 |
Penebel |
388,322.74 |
Payangan |
898,585.25 |
Pupuan |
936,196.76 |
Sukawati |
3,083,671.57 |
Selemadeg |
430,699.41 |
Tampaksiring |
977,279.91 |
Tabanan |
622,408.46 |
Table 6 shows that the highest per capita expenditure in Bali is Rp. 6,050,970.46 and the lowest per capita expenditure is Rp. 219,787,57. Based on the amount of per capita expenditure, the people in the South Denpasar Sub-district are much more prosperous than those in the Abang Sub-district, because of their higher per capita expenditure.
The normality assumption of error in SEBLUP model is carried out using the Anderson Darling test (a = 5%) with the null hypothesis: e~N(0,σ∕) againts the alternative hypothesis: e ^ N(0,σf ). The result is represented in Table 7.
According to Table 7, the assumption of normality of error is fulfilled or the error of SEBLUP model spreads normally. While testing the significance of the SEBLUP model parameters with the null hypothesis: βi = 0 againts the alternative hypothesis: βι ≠ 0 on the t-test (a = 5%) is summarized in Table 8.
Table 7. Normality Assumption of Error SEBLUP
Model
Test Statistics |
p-value |
Decision | |
0.27294 |
0.6546 |
Accept H0 | |
Table 8. Significance of Model SEBLUP Parameters | |||
Variable |
—■ β |
p-value |
Decision |
Population |
0.698396 |
1.04×10-15 |
Reject H0 |
Public health centers |
0.100963 |
1.23×10-1 |
Accept H0 |
Public primary schools |
-0.26089 |
6.97×10-4 |
Reject H0 |
Families using PLN |
0.298222 |
2.59×10-4 |
Reject H0 |
The population, public primary schools, and families using PLN variables in Table 8, have a significant effect on per capita expenditure in Bali based on the SEBLUP-SAE model.
Estimation using the EBLUP and the SEBLUP methods produce the estimated per capita expenditure and MSE of each method. Based on the previous discussion, the estimation result of the EBLUP and SEBLUP methods show the same sub-district in the highest per capita expenditure, namely the South Denpasar Sub-district. It also shows the same sub-district with the lowest per capita expenditure, namely Abang Sub-district. The best model is chosen through the mean square error of both models, where the model with the smallest MSE is best one. The comparison of MSE of both EBLUP and SEBLUP models is presented in Figure 1.
Figure 1 also shows that the MSE of the EBLUP method is smaller than the MSE of the SEBLUP method. In addition, the MSE average of the EBLUP method is lower, which is 0.1233 compared to the SEBLUP method, which is 0.1376. Therefore, the EBLUP-SAE method is better in estimating per capita expenditure in Bali than the SEBLUP-SAE one. Indeed, this condition shows that there is no spatial dependency on per capita expenditure among the subdistricts in Bali Province.
The Comparison of MSE (EBLUP and SEBLUP)
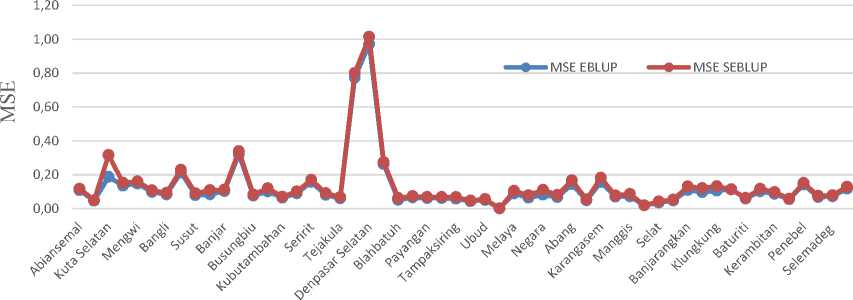
Sub-district
Figure 1. The Comparison of MSE EBLUP and SEBLUP
Based on the results of this study, per capita expenditure per sub-district in Bali, both the EBLUP and SEBLUP methods, is significant according to population, number of public elementary schools, and number of families using PLN. It is known that the MSE of the EBLUP method is smaller than the MSE of the SEBLUP method with a difference that is not much different. Thus, it can be concluded that the estimated per capita expenditure per district in Bali using the EBLUP method is better than the SEBLUP method. In addition, it can also be said that per capita expenditure per district in Bali has no influence between one sub-district and another.
The authors thank to: (1) the Faculty of Mathematics and Natural Science, Brawijaya University; and (2) BPS Bali for providing data.
REFERENCES
BPS. 2013. Survei Sosial Ekonomi Nasional
2013 Kor Gabungan.
https://microdata.bps.go.id/mikrodata/inde x.php/catalog/220, access on 25 September 2020.
BPS. 2018. Provinsi Bali dalam Angka. Badan Pusat Statistik Provinsi Bali.
BPS. 2019. Kemiskinan dan Ketimpagan. https://www.bps.go.id/subject/23/kemiski nan-dan-ketimpangan.html, accessed on 19 September 2020.
Matualage, D. 2012. Metode Prediksi Tak Bias Linear Terbaik Empiris Spasial pada Area Kecil untuk Pendugaan Pengeluaran per Kapita. IPB Postgraduate School, Bogor.
Nusrang, M., Annas, S., Asfar, A., Hastuty, H., and Jajang, J. 2017. Spatial EBLUP dalam Pendugaan Area Kecil. Sainsmat, 6(1), 59-66.
Pratesi, M., & Salvati, N. 2008. Small area estimation: the EBLUP estimator based on spatially correlated random area effects. Statistical methods and applications, 17(1), 113-141.
Rao, J.N.K. 2003. Small Area Estimation. John Wiley and Sons, US.
Sidauruk, M. A., & Sari, D. K. 2013.
Karakteristik Pendugaan Emperical Best Linear Unbiased Prediction (EBLUP) Pada Pendugaan Area Kecil. Prosiding SEMIRATA 2013, 1(1).
63
Discussion and feedback