Impact Evaluation Program Keluarga Harapan (PKH) On Household Expenditure For Education in Java
on
ISSN : 2301-8968
Vol. 14 No.1, Februari 2021
EKONOMI
KUANTITATIF
TERAPAN
Volume 14
Nomor 1
JEKT
Analisis Pengaruh Financial Indicators, Corporate Governance dan Variabel Ekonomi Makro Terhadap Financial Distress
Made Reina Candradewi, Henny Rahyuda
Satu Dekade Anggaran Pendidikan 20 Persen : Bagaimana Kualitasnya Dengan Keberhasilan Pendidikan dan Kemiskinan
Can Cryptocurrency, Gold and Bonds Play a Role as a Safe Haven for the Indonesian Capital Market? Paulus Hartono, Robiyanto
Keterbukaan Perdagangan, Aglomerasi Ekonomi Dan Permintaan Tenaga Kerja Manufaktur
Bukti Empiris Di Indonesia
Khairunnisah
Denpasar
Februari 2021
ISSN 2301-8968
Halaman
1-241
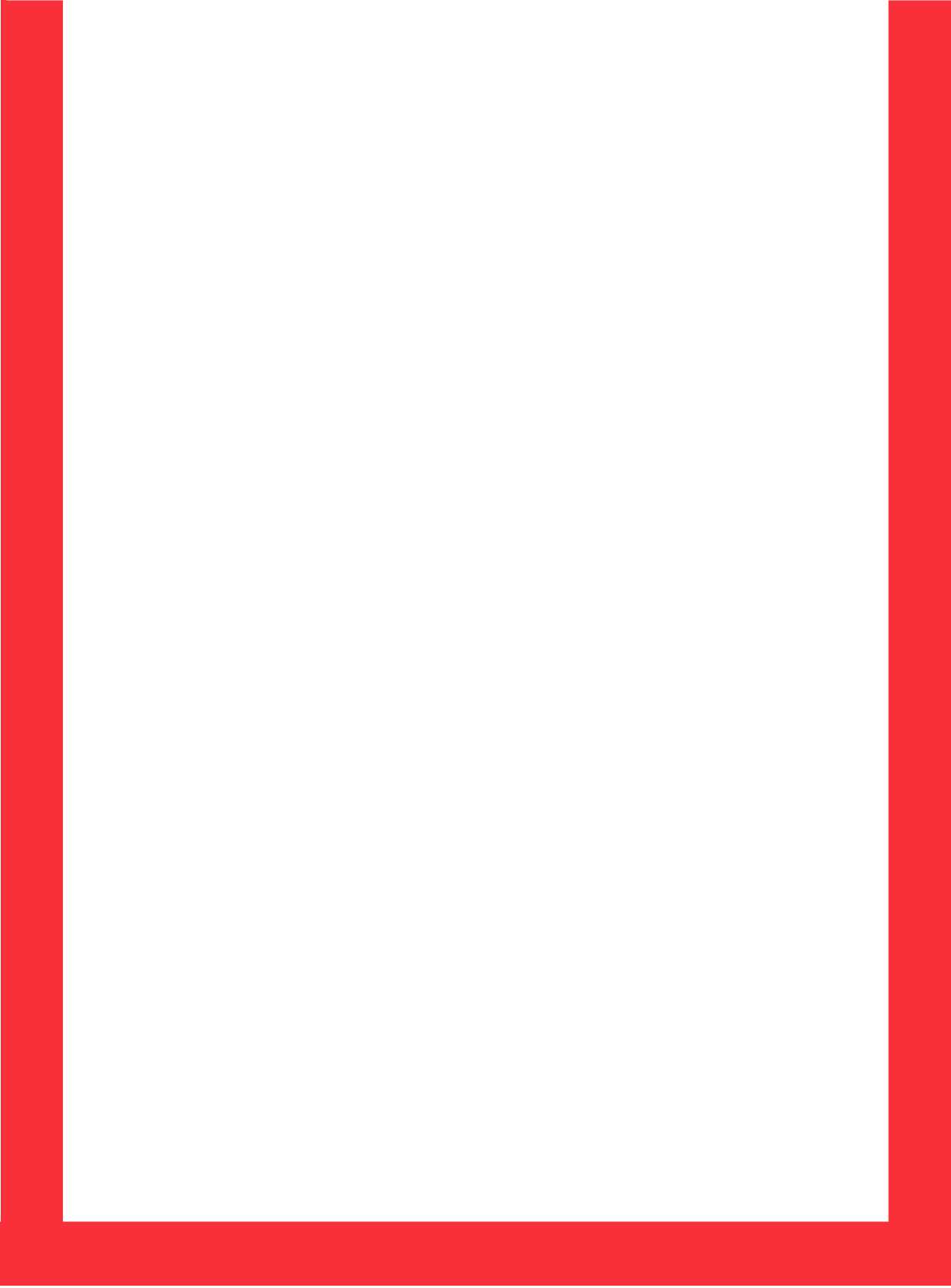
Hutang Luar Negeri yang Berkelanjutan di Negara-negara ASEAN terpilih
Apakah Data Makroekonomi dan Kelembagaan Penting?
Malik Cahyadin
Technical Efficiency of Independent Oil Palm Smallholder In Indonesia
Firna Varina, Sri Hartoyo, Nunung Kusnadi, Amzul Rifin
Impact Evaluation Program Keluarga Harapan (PKH) On Household Expenditure For Education in Java
Yunindyo Sasmito
Are Wealthier People Healthier?
Axellina Muara Setyanti, Nayaka Artha Wicesa
The Effect of Economic Openness, Democracy, and Institutional Quality on
Indonesia Exports to ASEAN Plus Three
Wikantioso
Putu Yudy Wijaya, Nyoman Reni Suasih
Analysis Of Factor Influencing The Interest Of Foreign Tourist To Revisit Bali
Agung Widanta
Tapping Box Application, Quality Service, Tax Knowledge, Tax Payer’s Obedience Whit Tax
Penalty As Moderating Variable
Ni Ketut Rasmini, Sri Harta Mimba
pISSN : 2301 – 8968
JEKT ♦ 14 [1] : 75-95
eISSN : 2303 – 0186
Evaluasi Dampak Program Keluarga Harapan (PKH) terhadap Pengeluaran
Rumah Tangga untuk Pendidikan di Pulau Jawa
Yunindyo Sasmito, Eny Sulistyaningrum
Universitas Gadjah Mada
ABSTRAK
Kemiskinan menjadi permasalahan global mencakup negara-negara berkembang maupun negara-negara maju. Untuk mengatasi permasalahan kemiskinan, berbagai negara menerapkan program pengentasan kemiskinan berupa paket kebijakan Conditional Cash Transfer (CCT). Di Indonesia program CCT diberi nama PKH. Program ini mewajibkan prasyarat (kesehatan dan pendidikan) kepada penerima program dengan tujuan agar dapat keluar dari lingkaran kemiskinan. Penelitian ini bertujuan untuk mengetahui dampak PKH terhadap pengeluaran rumah tangga untuk pendidikan di Pulau Jawa dengan menggunakan data IFLS 5 tahun 2014.
Penelitian ini menggunakan metode analisis Propensity Score Matching. Penelitian sebelumnya menunjukkan bahwa PKH di Indonesia tidak berdampak signifikan terhadap total pengeluaran rumah tangga untuk pendidikan. Dengan membagi komponen pengeluaran pendidikan, paper ini berhasil menunjukkan adanya dampak signifikan meningkatkan total pengeluaran pendidikan di dalam rumah tangga sebesar Rp1.031.963,53 per tahun. Peningkatan juga terjadi pada total biaya transportasi sebesar Rp603.085,86. Sedangkan total pengeluaran pendidikan di luar rumah tangga bagi penerima PKH justru menurun sebesar Rp277.475,49 per tahunnya.
Kata kunci: Evaluasi Dampak, PSM, Transfer tunai, Pengeluaran Pendidikan.
Klasifikasi JEL: H75, I22, I38, O15
Impact Evaluation Program Keluarga Harapan (PKH) On Household Expenditure
For Education in Java
ABSTRACT
Poverty becomes a global problem including developing countries and developed countries. To overcome the problem of poverty, various countries have implemented poverty alleviation programs in the form of a Conditional Cash Transfer (CCT) policy package. In Indonesia the CCT program is named PKH. This program requires prerequisites (health and education) to program recipients with the aim of getting out of the cycle of poverty. This study aims to determine the impact of PKH on household spending on education in Java using IFLS 5 data in 2014.
This study uses the Propensity Score Matching analysis method. Previous research shows that PKH in Indonesia has no significant impact on total household expenditure on
education. By dividing the components of education expenditure, this paper has successfully demonstrated the significant impact of increasing total education expenditure in the household by IDR 1,031,963.53 per year. The increase also occurred in the total transportation costs of IDR 603,085.86. While the total education expenditure outside the household for PKH recipients actually declined by IDR 277,475.49 per year.
Keyword: Impact Evaluation, PSM, Cash Transfer, Education Expenditures.
JEL Classification: H75, I22, I38, O15
INTRODUCTION
Poverty is an issue that is always interesting to study. In addition to the sensitive issue of poverty reduction is one measure of the success of development as a basis for planning a future state, and material evaluation of government programs. Poverty is not only a problem in developing countries but also a problem in developed countries.
Figure 1 Profile of Poverty in Indonesia (BPS, 2020)
Indonesia's poverty rate experiences a downward trend, even in 2018 for the first time since 1998 the percentage of poverty in Indonesia is below 10 percent and continues to fall until September 2019. Data also shows the number of poor people by island, Java
is an island with a number of people the highest poverty rate among other large islands in Indonesia (BPS, 2020).
In total there are still 24,79 million poor people in Indonesia, to overcome poverty some countries have implemented a conditional cash transfer (CCT) policy program, this program provides conditional cash assistance to poor households to help with their daily needs.
Conditional cash transfer (CCT) programs offer countries a new way to overcome poverty and prevent the transmission of poverty to future generations. They do so by providing regular cash payments to families on the condition that they fulfill basic obligations, which are typically related to the usage of health and education services (Alatas, 2011).
CCT programs in Indonesia was named the Program Keluarga Harapan (PKH). This program aims to reduce
poverty by targeting poor households and poor vulnerable households. CCT was first launched in July 2007 with a pilot in 7 provinces (DKI Jakarta, West Java, East Java, North Sulawesi, Gorontalo, East Nusa Tenggara, and West Sumatra) with the target audience of 500,000 households (TNP2K, 2014)
In 2012 PKH finally operated in all provinces in Indonesia, although it still did not reach all districts in each province. Expanding PKH coverage is a program challenge if it wants to have a large impact on Indonesia's poor population (Nazara and Rahayu, 2013)
PKH is given to Very Poor family or Keluarga Sangat Miskin (KSM), which meets at least one criterion of membership programs, namely, (1) having pregnant / postpartum / toddlers; (2) have children aged 5-7 years who have not entered primary education; (3) children aged SD/MI/PaketA/SDLB; (4) Child Junior/MTs/Package B/SMLB; (5) children 15-18 years old who have not completed basic education, including children with disabilities. The whole family in a household is eligible to receive cash assistance if they meet the
criteria for membership in the program and meet its obligations.
The obligations that must be carried out and fulfilled by PKH participants include, among others, (1) the health component requires PKH participants to use prenatal services, the birth process must be assisted by trained health workers, postnatal services as well as immunizing children and monitoring child growth and development routinely; (2) the education component requires PKH participating children to be registered and attend school at least 85 percent of the number of valid school days. The stipulation of these requirements is expected to bring changes in the behavior of PKH participants to the importance of health and education for their children.
One of the indicators of program success can be measured by the effect of the program on household expenditure. One of the specific objectives of PKH is to improve the level of education of PKH participants so that indicators of the success of this goal can be seen from the household expenditure for education. Is there a difference between program
beneficiary households and
households that don't get the program.
Table 1 the number of poor people by island | |||
Island |
Total Poor Populations (in 1,000) | ||
Rural |
Urban |
Total | |
Sumatera |
2.062,94 |
3.709,47 |
5.772,41 |
Jawa Bali dan |
6.338,48 |
6.217,43 |
12.555,90 |
Nusa Tenggara Kalimant |
564,78 |
1.427,26 |
1.992,04 |
an |
329,36 |
632,15 |
961,51 |
Sulawesi Maluku |
432,49 |
1.556,27 |
1.988,76 |
dan Papua |
129,70 |
1.385,53 |
1.515,23 |
Indonesia 9.857,74 Source: (BPS, 2020) |
14.928,11 |
24.785,85 | |
Java island is an island with |
the |
least |
85 percent at school. This will |
highest number of poor people in Indonesia. According to BPS (2020), the number of poor in Java amounted to 12.55 million out of 24,7 million poor people in Indonesia. The data shows that nearly 50 percent of citizen poor in Indonesia are located in Java. BPS poverty rate calculations based on the concept of ability to meet basic needs (Basic Needs Approach) means that poverty is seen as incompetence in economic terms to meet the basic needs of food and non-food (BPS, 2020).
One of the specific objectives of PKH is to improve the level of education of participants where participants must be able to complete basic education and attendance of at
later have an impact on spending on household education costs that will increase.
Soares et al. (2010) estimated the impact of the Bolsa Familia program on inequality, poverty, consumption, education, health care, and labor force participation. With the PSM method and using data from A nationally and regionally representative sample survey carried out by Cedeplar and commissioned by the Ministry of Social Development (MDS). The results obtained by the Program affect monthly education spending up by R $ 2.65 but do not significantly affect total household consumption (Soares, Ribas and Osório, 2010).
Specific research on PKH conducted by Bappenas shows that PKH has a positive impact. On education indicators, the attendance rate of students in class rose 0.2 percent point. On average, PKH increases per capita household expenditure per month for the education component, each IDR 2,786 (Bappenas, 2009). Based on research using IFLS 5 data, Almunawaroh revealed that PKH has a significant effect on total household expenditure, but does not significantly affect the increase or decrease in household expenditure for education (Almunawaroh, 2016).
Different from Almunawaroh that describes household expenditure for education in general, this study describes the components of household expenditure for education to be more specific. Household expenditure for education is divided into school fees, schooling needs cost, transportation costs, pocket money and boarding fees. after that, every component divided on a child or family inside the household and outside the household.
The selection of the research locus on the Java Island because Java is the island with the largest number of poor people in Indonesia. From the results of the IFLS 5 survey data tabulation it is also known that 371 households in Indonesia responded to PKH, while 264 households were in Java. It is interesting to evaluate the PKH program in terms of the objectives to be achieved namely whether there is a significant difference in the expenditure of education costs for PKH recipients and not PKH recipients specifically in Java.
RESEARCH METHOD
This study aims to see whether the Program Keluarga Harapan (PKH) has an impact on household expenditure on education costs. This is important because it is related to the purpose of PKH in improving the quality of education. This study uses a quasiexperimental approach. The data used are secondary data from the survey data IFLS wave 5 in 2014. With these data, the analysis method to use is the matching method because this method has been popular for use as a method of estimation for the causal treatment
effect and can accommodate the possibility of selection bias. The matching method with the objectives of this study is to analyze the relationship between the dependent variable that household expenditure for education by the independent variable is participation PKH.
Participation PKH in this paper is participation PKH in Java. This data was obtained from a questionnaire IFLS Book 1 Section KSR in question KSR17 "Has this household ever receive cash transfer from [...] program?" With a selection of "Yes" responses represented by the numeral 1 in the variable ksr17 to ksr3type status "B" in the file b1_ksr1.dta.
To retrieve PKH program recipient data in Java, the "PKH" interest variable will be combined with the "Java" control variable using the stata 14 analysis tool with the command [keep if PKH == Java] meaning to retain the "PKH" data if the value is equal to "Java ". Furthermore, the control variable data constructed refers to 14 poverty criteria according to BPS (2014). Of the poverty criterion, this study successfully using 7 criteria, such as,
(1) the type of floor; (2) wall type; (3) toilet facilities; (4) Availability of electricity; (5) drinking water sources; (6) cooking fuel; (7) the highest education of the family head. This paper also adds some control variables beyond the 14 characteristics of poverty among other things, the type of roof; homeownership; water purchase; SKTM ownership; BLSM membership; BSM membership; marital status of the household head; household head activities; number of family members; the sex of the household head; households living in cities; the age of the household head. (see Appendix Table 2). Results data or outcome variable data that are examined as the impact of PKH are education expenditure. This education expenditure data is household expenditure for one year in units of Rupiah (IDR). Data were obtained from the IFLS questionnaire 5 Book 1 section KS (consumption) with the question "What is (roughly) expenditure on school fees for the past 1 year from all household members?" (See Appendix Table 3).
This study uses a matching method. The basic idea of the
matching method is to match between the treatment group and the control group. One of these matching methods is the Propensity Score Matching (PSM) method. This PSM method was introduced by Rosenbaum and Rubin (1983). The purpose of this method is to choose observable factors, two groups with the same value of these factors will show no differences in the characteristics of the sample reaction to the intervention/policy or in other words the aim is to find the closest comparison group from a sample nonparticipants in the sample of program participants. The word "closest" is measured in terms of observable characteristics. Propensity scores are defined as conditional probabilities of receiving an intervention based on characteristics before the intervention (Rosenbaum and Rubin, 1983).
According to Caliendo and Kopeinig (2008), the core model in this study consisted of households, treatment (PKH participation) and outcomes (household education expenditure) (Caliendo and Kopeinig, 2008). The model is as follows,
Yi = DiYii + (1 - Di) Yoi ......(1)
Where:
Di ε {0,1} is the symbol for the treatment group. It will be equal to (1) if the household (i) is a PKH program participant and will be equal to 0 (zero) for the others. Yi is the outcome indicator that is education
expenditure. Y1i is the expected result outcome / (education expenditure) when household (i) is a PKH participant, which is the result of treatment or when Di is equal to (1). Y0i is the expected result when the household (i) is not a participant in the PKH program, which is the result of the control or when Di is equal to 0 (zero).
Thus the effect of the intervention on the individual can be written into the equation,
Τ = Yii - Yoi ......(2)
because it is not possible to observe a household when receiving treatment without receive treatment at the same time, the estimated of the treatment effect is done through the average treatment effect on the treated (ATET) is defined as follow,
τATET = E [Yii- Yoi | Di = 1]
τATET = E (τ |Di = 1)
τATET = E [Y1i |Di =1] -E [Y0i |Di = 1] ……(3)
E [Y1i | Di = 1] is a potential outcome of households that receive PKH and can be observed. E [Y0i | Di = 1] is a potential outcome to receive PKH when they don’t receive and cannot be observed because it is a counterfactual of data loss. To find the value ATET, the researcher should be able to find a replacement value of E [Y0i | Di = 1]. One way is to use the potential outcome of the households that don’t receive PKH E [Y0i | Di = 0] because of the potential outcome of the households that don’t receive PKH are not examined at the same time when households receive the intervention. So ATET can be searched using the formula:
τATET =E [Y1i | Di =1] -E [Y0i |Di = 0] ..….(4)
from here, ATET is an estimate of the potential outcomes of households that receive PKH E[Y1i | Di =1], minus the potential outcomes of the households that don’t receive PKH E[Y0i | Di = 0].
Before estimating the 5 stages of PSM, the data must be certain to meet
2 assumptions namely Conditional Independence Assumption (CIA) and common support. Potential outcomes are independent of treatment assigned based on observable attributes of covariate x which are not affected by treatment (Caliendo and Kopeinig, 2008). The difference in observed characteristics between the treatment group and the group that did not receive treatment should be controlled; the outcome of treatment absence was the same in both cases. Determination of assumptions for matching also determines assumptions for a simple regression estimator known as Conditional Independence Assumption (CIA).
Common support is a condition where there are areas that support overlap matching variables in the distribution of the density values of treatment groups and groups that do not receive treatment. The common support area is the range score that overlaps between the density values for groups that did not receive treatment and the density values for groups that received treatment.
After passing through the two PSM assumptions, the next process is 5
stages of PSM. PSM following 5 steps. First, Estimating the propensity score. According to (Caliendo and Kopeinig, 2008), there are two steps to be taken when estimating the Propensity Score, namely: choosing a model and choosing variables that must be included in the model. For the choice of models that can be used, namely Binary Logit, Binary Probit, Multinomial Logit, Conditional Logit, and Multinomial Probit. The choice of model is not very important when an intervention is applied to one of two groups, but when the model uses several interventions, several assumptions must be satisfied. Moreover, the choice of variables chosen must refer to economic theory and previous studies that have been found.
The model used in this study is a Binary Logit model with the dependent variable being PKH participation (1 = PKH participants and 0 = others) and the independent variable is poverty factors and other factors that determine households to obtain PKH.
For the poverty factors, a new variable is created which results from
the sum of all the poverty variables in this study as follows:
poor = No_electric + Water_Source + No_Toilet + Cooking_Source + Floor +
Roof + Wall + HHHeduc ......(5)
Thus obtained logit model equations used are:
Logit (PKH) = α1 + α2poor + α3SKTM + α4Purchase_Water + α5HHHMarried +
α6HHHActivity + α7HHsize + α8HHHFemale + α9HHHAge +
α10Urban + α11Rooms + α12NoHouse +
Ui ......(6)
After the process logit estimation process will be performed propensity score, then every observation in the treatment and control groups were matched (matching) which have the same propensity score value.
Second, Choosing a matching algorithm. There are several matching techniques used in this stage is Nearest Neighbor Matching, Caliper and Radius matching, Stratification and Interval Matching, Kernel and Local Linear matching, and weighting. The selection method for matching
where the literature has not found the best method among others.
Third, Checking common support. Common support ensures that the equivalent could be found between the treatment group and the control group when compared to see the distribution of both. This assumption can be met if there is a cross-sectional area (overlap) on the density of the propensity score between treatment and control groups.
Fourth, Measuring the quality of matching (match assessing quality). Tests that can be carried out to measure the quality of matching include the standard bias test, the average difference test before and after matching (t-test) and the test of the merging of variables in the matched sample (F-test). If the matching quality is poor, or there is still a difference, it is better to step back and repeat the steps taken to get a good and satisfying matching quality.
Last step, Sensitivity analysis. To deal with the problem of ignoring standard errors because the variations exceed the normal sample variations when estimated. (Lechner, 2002) suggests using a standard
bootstrapped error that is usually used when the parameters of the sample distribution may not be standard. Bootstrapped standard errors rely on the assumption that the current sample represents the population. Besides, sensitivity analysis should be applied to estimate the level of bias in research/investigations (Guo and Fraser, 2010). Based on (Rosenbaum and Rubin, 1983) and (Rosenbaum, 2005), sensitivity analysis is used to see hidden biases when the treatment and control groups may differ. Among other things, sensitivity analysis can be done by looking at Wilcoxon's signed-rank test developed by Rosenbaum. (Rosenbaum, 2005).
RESULTS AND DISCUSSION
This study uses a quasi-experimental approach method. The data used are secondary data from the survey data IFLS wave 5 in 2014.
Table 4 Distribution of the data sample | ||
Province code |
Province |
Household |
31 |
D.K.I Jakarta |
970 |
32 |
West Java |
2,148 |
33 |
Central Java |
1,861 |
34 |
D.I. Yogyakarta |
808 |
35 |
East Java |
2,073 |
36 |
Banten |
602 |
Total |
8,462 |
Source: IFLS 5, processed
The number of samples taken for 8,462 households in Java. The sample is divided into 6 provinces. The largest sample is in the province of East Java with a total of 2,073 households, while
the smallest sample is from the province of Banten. Then observation will be eliminated due to the merging of data into as shown in the descriptive statistics table .
Table 5 Descriptive Statistics of research variables | ||||||
variables |
variable name |
Obs |
Average |
Std. dev |
Min |
Max |
interest |
PKH |
6,797 |
0.365 |
0.187 |
0 |
1 |
outcome |
Total_Educ_Exp |
6,736 |
4,298,805 |
8,190,701 |
0 |
15,300,000 |
Educ_Exp_IHH |
6,764 |
3,116,859 |
5,680,665 |
0 |
8,900,000 | |
Educ_Exp_OHH |
6,758 |
1,189,794 |
5,484,237 |
0 |
14,200,000 | |
Total_School_Fees |
6,771 |
1,233,315 |
3,633,343 |
0 |
6,200,000 | |
School_Fees_IHH |
6,774 |
872,276.5 |
2,854,300 |
0 |
6,200,000 | |
School_Fees_OHH |
6,787 |
362,807.6 |
2,104,558 |
0 |
5,420,000 | |
Total_Schooling_Need |
6,758 |
505,426.3 |
1,077,650 |
0 |
3,020,000 | |
Schooling_Need_IHH |
6,777 |
420,633.6 |
788,194.1 |
0 |
1,800,000 | |
Schooling_Need_OHH |
6,771 |
86,500.07 |
703,534.4 |
0 |
3,000,000 | |
Total_School_Transport |
6,768 |
2,307,279 |
4,528,829 |
0 |
7,460,000 | |
School_Transport_IHH |
6,784 |
1,825,255 |
3,451,093 |
0 |
6,300,000 | |
School_Transport_OHH |
6,776 |
481,109.1 |
2,853,485 |
0 |
7,200,000 | |
Food_Rent_School |
6,788 |
289,050.6 |
1,645,119 |
0 |
4,500,000 | |
Control |
NoHouse |
6,777 |
0.300 |
0.458 |
0 |
1 |
No_Electric |
6,777 |
0.013 |
0.115 |
0 |
1 | |
Water_Source |
6,777 |
0.468 |
0.499 |
0 |
1 | |
Purchase_Water |
6,777 |
0.396 |
0.489 |
0 |
1 | |
No_Toilet |
6,777 |
0.183 |
0.387 |
0 |
1 | |
Cooking_Source |
6,777 |
0.334 |
0.471 |
0 |
1 | |
SKTM |
6,777 |
0.213 |
0.410 |
0 |
1 | |
BLSMcard |
6,777 |
0.170 |
0.375 |
0 |
1 | |
BSM |
6,777 |
0.141 |
0.348 |
0 |
1 | |
HHHMarried |
6,795 |
0.810 |
0.392 |
0 |
1 | |
HHHActivity |
6,795 |
1.731 |
1.597 |
1 |
7 | |
HHHeduc |
6,795 |
0.380 |
0.485 |
0 |
1 | |
HHsize |
6,795 |
1.820 |
1.431 |
1 |
11 | |
HHHFemale |
6,795 |
0.158 |
0.365 |
0 |
1 | |
HHHAge |
6,795 |
43.645 |
14.497 |
9 |
94 | |
Rooms |
6,794 |
5.366 |
2.392 |
1 |
40 |
Table 5 Descriptive Statistics of research variables
variables |
variable name |
Obs |
Average |
Std. dev |
Min |
Max |
floor |
6,795 |
0.210 |
0.407 |
0 |
1 | |
Wall |
6,795 |
0.315 |
0.465 |
0 |
1 | |
Roof |
6,770 |
0.009 |
0.097 |
0 |
1 | |
Urban |
6,797 |
0.503 |
0.500 |
0 |
1 | |
Java |
6,797 |
0.036 |
0.187 |
0 |
1 | |
poor |
6,749 |
1.917 |
1.625 |
0 |
8 |
Source: IFLS 5, processed
The table above explains the descriptive statistics of the variables of interest, outcome variables and control variables. After merging the data, the number of observations became 6797 households. Interest variable is a PKH recipient dummy, outcome variable is a household expense for education funding in Rupiah. While the control variable is a poverty criterion variable
that is majority dummy. There are some non-dummy control variables such as HHHActivity shows 7 activities during the last 1 week during the survey, then HHsize is the size of the household or the number of household members, HHage is the age of the head of the household, and Rooms are the number of rooms in the household.
ITable 6 Results of propensity score
Inferior of block of pscore |
PKH Participation Total Yes No |
0 0.025 0.05 0.10 0.20 |
40 2,927 2,967 94 2,336 2,430 81 1,053 1,134 31 221 252 2 12 14 |
total |
248 6549 6,797 |
Source: IFLS 5, processed
This study uses a logit model to estimate the impact of PKH. This study uses 20 control variables. To achieve the CIA condition, 2 variables must be discarded, including the BLSMcard and BSM variables, after the BLSMCard and BSM variable drop
processes are obtained the results are “satisfied” and show that the model meets the CIA conditions.
Table 7 logit regression results PKH
Dependent Variables: PKH |
parameter Estimates | |
Coefficient |
standard Error | |
poor | ||
1 |
0.769 *** |
0.266 |
2 |
1.308 *** |
0.264 |
3 |
1.376 *** |
0.283 |
4 |
1.472 *** |
0.314 |
5 |
1.457 *** |
0.365 |
6+ |
1.347 *** |
0.511 |
SKTM |
0.935 *** |
0.136 |
Purchase_Water |
0.155 |
0.155 |
HHHMarried |
1.391 *** |
0.274 |
HHHActivity |
0.045 |
0.041 |
HHsize |
-0.005 |
0.050 |
HHHFemale |
1.027 *** |
0.227 |
HHHAge |
-0.0007 |
0.005 |
Urban |
0.392 *** |
0.148 |
Rooms |
0.011 |
0.032 |
NoHouse |
-0.529 *** |
0.182 |
constants |
-6.169 *** |
0.527 |
Description: The dependent variable is PKH, where dummy 1 equal receive the program and 0 is other. *** significant at alpha 1 percent, ** significant at alpha 5 percent,* Significant at alpha 10 percent.
Source: IFLS 5, processed
After fulfilling the CIA assumption, a logit estimate is performed. The coefficient values shown in Table 7. Result show the probability of households receiving the PKH program. The poor variable as a poverty characteristic variable specified in 1-6 shows a coefficient
value that increases with the
After logit estimate, then the next step is 5 steps PSM. The first stage to estimate the propensity score is a selection matching algorithm. This study uses the Kernel Matching because there are differences in the distribution of data between treatment group and the control group (treated and untreated group).
increasing criteria for poverty,
meaning that the probability of
households receiving the higher the
CCT program if the household
increasingly meets the criteria of
poverty.
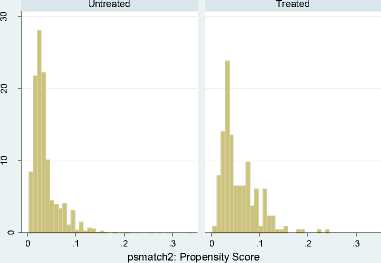
Graphs by psmatch2: Treatment assignment
-
Figure 2. Comparison of the distribution of the value of propensity score (IFLS 5, processed)
Figure 2 shows that the value of density in the untreated group (control) was higher than in the treated group (Treatment).
The next step is checking common support. Common support requires that the treatment and control groups have the same propensity values after matching.
Figure 3 shows that there are overlapping propensity scores. This can be seen from the red axis which represents the group that received the program (treatment group) and the blue axis for the group that did not get the treatment (control group). the two intersecting graphs show common support.
To check the success of matching all independent variables, there are
several tests conducted after matching. This checking according to (Caliendo and Kopeinig, 2008) is assessed matching quality by looking at the standard bias, t-test to determine the average equivalence before and after the matching process, F-test is used to find out the average quality together in the matched sample.
Kernel density estimate
0 .1 .2 .3 .4
psmatch2: Propensity Score
untreated treated
kernel = epanechnikov, bandwidth = 0.0029
-
Figure 3 Distribution propensity score and Common Support to estimate propensity score (IFLS 5, processed)
Table 8 standard test results matching bias of kernel for total spending on education
variables |
Before Matching After Matching (%) (%) |
Total_Educ_Exp Poor SKTM Purchase_Water HHHMarried HHHActivity HHsize HHHFemale HHHAge Urban Rooms NoHouse |
3.9 4.3 34.2 30.7 47.9 44.4 -15.4 -13.3 22.0 18.5 9.5 9.1 -8.5 -7.7 8.1 8.2 13.8 11.5 1.1 1.5 -5.8 -6.0 -29.9 -25.5 |
mean Bias
16.7
15.1
Source: IFLS 5, processed
Table 8 shows the results of the standard bias test, it is seen that 8 variables out of 13 variables experienced a reduction in the percentage of bias. The mean bias is
reduced by 1.6 percent after matching. So it can be concluded that the matching process in this study was successful.
Table 9 Test average difference before and after matching (t-test) | ||
variables |
p-value of t-test | |
Before matching |
After matching | |
Total_Educ_Exp |
0.566 |
0.634 |
Poor |
0.000 |
0.001 |
SKTM |
0.000 |
0.000 |
Purchase_Water |
0.020 |
0.139 |
HHHMarried |
0.002 |
0.038 |
HHHActivity |
0.132 |
0.317 |
HHsize |
0.196 |
0.391 |
HHHFemale |
0.197 |
0.362 |
HHHAge |
0.061 |
0.203 |
Urban |
0.862 |
0.871 |
Rooms |
0.436 |
0.507 |
NoHouse |
0.000 |
0.004 |
Source: IFLS 5, processed Table 9 shows the results of the |
condition is |
as expected because in |
average equality quality test before |
general it is |
indicated that there is no |
and after matching (t-test). The results |
significant |
difference between the |
show that overall the p-value after |
average propensity scores in the two | |
matching is not significant, this |
groups. |
Table 10 Hotelling test after matching
variables |
Mean PKH = 1 |
Mean PKH = 0 |
Total_Educ_Exp |
4,526,427 |
4,230,975 |
Poor |
2.431 |
1.899 |
SKTM |
0.423 |
0.206 |
Purchase_Water |
0.325 |
0.399 |
HHHMarried |
0.886 |
0.807 |
HHHActivity |
1.882 |
1.726 |
HHsize |
1.703 |
1.823 |
HHHFemale |
0.187 |
0.156 |
HHHAge |
45.325 |
43.562 |
Table 10 Hotelling test after matching
variables |
Mean PKH = 1 Mean PKH = 0 |
Urban Rooms NoHouse Hotelling p-value number of observations |
0.508 -0.502 5.240 5.361 0.179 0.305 0.000 246 6,442 |
Source: IFLS 5, Processed
Table 10 shows the results of the hotelling test, it appears that the hotelling p-value is less than 5 percent, meaning that the combination of control variables is unsatisfactory. Overall 3 methods of quality matching testing can be concluded that the control group has the same characteristics as the treatment group.
Last, the sensitivity analysis needs to be carried out as an
evaluation. Acccording to Rosenbaum research, selection bias may occur when two individuals with the same covariate have a different probability value in determining intervention. To handle with selection bias and also hidden bias, Rosenbaum suggested that a sensitivity analysis test be conducted using Wilcoxon's signed-rank test to determine the Rosenbaum limit (Sulistyaningrum, 2016).
Table 11 Summary results of sensitivity test
Outcome Sensitivity Test Results
Total_Educ_Exp |
sensitive |
Educ_Exp_IHH |
sensitive |
Educ_Exp_OHH |
Not sensitive |
Total_School_Fees |
Not sensitive |
School_Fees_IHH |
sensitive |
School_Fees_OHH |
Not sensitive |
Total_Schooling_Need |
sensitive |
Schooling_Need_IHH |
Sensitf |
Schooling_Need_OHH |
Not sensitive |
Total_School_Transport |
sensitive |
School_Transport_IHH |
sensitive |
School_Transport_OHH |
Not sensitive |
Food_Rent_School |
Not sensitive |
Source: IFLS 5, Processed | |
The results of the sensitivity test |
are sensitive to hidden bias. The |
summaries in Table 11 show the |
results of this sensitivity test indicate a |
results of various tests. There is 6 |
weakness of this study because there |
outcome variable with the results not |
are a number of test results showing |
sensitive to hidden bias, and 7 others |
that there are still hidden biases. Good |
test results show no sensitivity to hidden bias.
CONCLUSION AND SUGGESTION This study provides empirical evidence that Program Keluarga Harapan (PKH) in Java doesn’t have a significant impact on total household expenditure for education, but specifically, it turned PKH able to increase education expenditure for children or families in the household significantly by IDR1,031,963.53 per year. An increase also occurred in total transportation costs by an average of IDR603,085.86 per year.
Moreover, the education expenditure of children or families outside the household has a significant negative impact. Each of them for school fees, the school expenses, transportation costs, and rental fees for PKH recipients must reduce spending by IDR205,654.42. IDR50,901.28 IDR277,457.49 and IDR209,241.53 per year (see table 12).
Referring to the results of this study, there are several implications for program development and policy implementation as follows, (1) Increase the budget allocation so that it
can reach the educational needs of children or families outside the household; (2) The need for companion participation in conducting financial management training so that PKH recipient households can manage finances well; (3) The government through relevant ministries needs to ensure that educational support facilities are well provided, access from home to educational facilities can be easily reached.
REFERENCE
Alatas, V. (2011) Program Keluarga Harapan: impact evaluation of
Indonesia’s Pilot Household
Conditional Cash Transfer
Program (English). 72506.
Indonesia. Available at:
http://documents1.worldbank. org/curated/en/5891714682661 79965/pdf/725060WP00PUBL0l uation0Report0FINAL.pdf.
Almunawaroh, D. F. (2016) Dampak Kebijakan Program Keluarga Harapan Terhadap Konsumsi Rumah Tangga Indonesia Tahun 2014. Universitas Gadjah Mada.
Bappenas (2009) ‘Laporan Akhir Evaluasi Program Perlindungan Sosial: Program Keluarga
Harapan 2009’. Available at: http://perpustakaan.bappenas. go.id/lontar/file?file=digital/9 8524-[_Konten_]-Konten C6575.pdf.
BPS (2020) Profil Kemiskinan di Indonesia September 2019. Jakarta. Available at:
elease/download.html?nrbvfev e=MTc0Mw%3D%3D&sdfs=ldjf difsdjkfahi&twoadfnoarfeauf= MjAyMC0wNy0wNiAxMzo0M To0MA%3D%3D.
Caliendo, M. and Kopeinig, S. (2008) ‘Some practical guidance for the implementation of propensity score matching’, Journal of
Economic Surveys, 22, pp. 31–72. doi: 10.1111/j.1467-
6419.2007.00527.x.
Guo, S. Y. and Fraser, M. W. (2010) Propensity Score Analysis: Statistical Methods and
Applications, Counterfactual
Framework and Assumptions.
Lechner, M. (2002) ‘Some practical issues in the evaluation of heterogeneous labour market programmes by matching methods’, Journal of the Royal Statistical Society. Series A: Statistics in Society. doi: 10.1111/1467-985X.0asp2.
Nazara, S. and Rahayu, S. K. (2013) Program Keluarga Harapan (PKH): Program Bantuan Dana Tunai Bersyarat di Indonesia, International Policy Centre for Inclusive Growth. Jakarta. Available at:
http://www.tnp2k.go.id/imag es/uploads/downloads/PKH IPC Policy Research
Brief__Bahasa-2.pdf.
Rosenbaum, P. R. (2005) Sensitivity Analysis in Observational Studies Randomization Inference and Sensitivity. Edited by Brian S. Everitt & David C. Howell. Chichester: Encyclopedia of
Statistics in Behavioral Science. doi: https://doi.org/10.1002/04700 13192.bsa606.
Rosenbaum, P. R. and Rubin, D. B.
(1983) ‘The central role of the propensity score in
observational studies for causal effects’, Biometrika. doi:
10.1093/biomet/70.1.41.
Soares, F. V., Ribas, R. P. and Osório, R. G. (2010) ‘Evaluating the impact of Brazil’s Bolsa Família: Cash transfer programs in comparative perspective’, Latin American Research Review.
Sulistyaningrum, E. (2016) ‘Impact Evaluation of the School Operational Assistance Program (Bos) Using the Matching Method’, Journal of Indonesian Economy and Business, 31(1), p. 33. doi: 10.22146/jieb.10319.
TNP2K (2014) Reaching Indonesia’s Poor and Vulnerable and Reducing Inequality: Improving Programme Targeting, Design, and Processes. Jakarta. Available at:
http://www.tnp2k.go.id/imag es/uploads/downloads/Report _REACHING_Mar30_LR.pdf.
APPENDIX
Table 2 List of control variables
No. |
variable control |
variable type |
Definition |
Name File / Variable |
1 |
floor |
dummy |
Type floor of the building 1 = Land, bamboo, wood / board 0 = other |
Bk_krk, krk08 |
2 |
Wall |
dummy |
Type of wall, 1 = timber / boards / plywood 0 = other |
Bk_krk, krk09 |
3 |
Roof |
dummy |
This type of roof 1 = leaves / palm 0 = other |
Bk_krk, krk10 |
4 |
No_Toilet |
dummy |
ownership toilet 1 = latrines shared / common / time / garden / ditch / pond / ocean 0 = other |
B2_kr, kr20 |
5 |
No_Electric |
dummy |
ownership of electricity 1 = don’t use electricity 0 = use electricity |
B2_kr / kr11 |
6 |
Water_Source |
dummy |
The main source for drinking, 1 = well / spring / river water 0 = other |
B2_kr, kr13 |
7 |
Cooking_Source |
dummy |
Cooking fuel, 1 = firewood / charcoal / kerosene / no cooking 0 = other |
B2_kr, kr24 |
8 |
HHHeduc |
dummy |
Education of household head, 1 = no school, elementary, kindergartens, package A 0 = other |
*Bk_ar1, ar16 |
9 |
NoHouse |
dummy |
Home ownership 1 = occupied / rental 0 = own their own home |
B2_kr / KR03 |
10 |
Purchase_Water |
dummy |
Is the home water purchased? 1 = yes 0 = no |
B2_kr, kr17b |
11 |
SKTM |
dummy |
ownership SKTM 1 = yes 0 = no |
B2_kr, kr27a |
12 |
BLSMcard |
dummy |
BLSM card ownership 1 = yes 0 = no |
B2_kr, kr27b |
13 |
BSM |
dummy |
Households got BSM 1 = yes 0 = no |
B2_kr, kr27d |
14 |
HHHMarried |
dummy |
Status married households head 1 = married 0 = other |
*Bk_ar1, ar13 |
15 |
HHHActivity |
discrete |
Household head activities last week |
*Bk_ar1, ar15c |
16 |
HHsize |
Person |
Number of household members |
*Bk_ar1, ar01a |
17 |
HHHFemale |
dummy |
The sex of the household head 1 = female 0 = male |
*Bk_ar1, ar07 |
Table 2 List of control variables
No. variable control variable type |
Definition Name File / Variable |
18 Urban dummy |
Location dwelling households Bk_sc1, sc05 1 = town 0 = village |
19 HHHAge Year 20 Java dummy |
Age of household head *Bk_ar, ar09 Category territory Bk_sc1, 1 = java sc01_14_14 0 = other |
information: * If variable ar02b equal 1 (for household head)
Source: IFLS 5
Table 12 Impact of PKH
Outcome Variables |
Impact |
Number of observations |
Total_Educ_Exp |
319,719.76 *** |
6,688 |
Educ_Exp_IHH |
1,031,963.53 *** |
6,716 |
Educ_Exp_OHH |
-713,808.25 *** |
6,710 |
Total_School_Fees |
-114,437.33 *** |
6,723 |
School_Fees_IHH |
89,431.34 *** |
6,726 |
School_Fees_OHH |
-205,654.42 *** |
6,739 |
Total_Schooling_Need |
4,539.24 *** |
6,710 |
Schooling_Need_IHH |
52,730.01 *** |
6,729 |
Schooling_Need_OHH |
-50,901.28 *** |
6,723 |
Total_School_Transport |
603,085.86 *** |
6,720 |
School_Transport_IHH |
887,805.35 *** |
6,736 |
School_Transport_OHH |
-277,475.49 *** |
6,728 |
Food_Rent_School |
-209,241.53 *** |
6,740 |
Description: *** significant at alpha 1 percent, ** significant at alpha 5 percent, * significant at alpha 10 percent
Source: IFLS 5, processed
Table 3 List of the outcome variables
No. |
variables Definition source variable |
1 |
Total_Educ_Exp Total spending on education ks10aa + ks10ab + ks11aa + ks11ab + ks12aa + ks12ab + ks12bb |
2 |
Educ_Exp_IHH Total expenditures on education of ks10aa + ks11aa + ks12aa children / families in the household |
3 |
Educ_Exp_OHH Total expenditures on education of ks10ab + ks11ab + ks12ab + children / family outside the ks12bb household |
4 5 |
Total_School_Fees The total cost of attending school ks10aa + ks10ab School_Fees_IHH School fees of children / families in ks10aa the household |
6 |
School_Fees_OHH School fees of children / family ks10ab outside the household |
7 8 |
Total_Schooling_Need Total cost of school supplies ks11aa + ks11ab Schooling_Need_IHH The cost of school supplies children / ks11aa families in the household |
9 |
Schooling_Need_OHH The cost of school supplies children / ks11ab |
Evaluasi Dampak Program Keluarga Harapan (PKH) terhadap Pengeluaran …..Yunindyo Sasmito family outside the household | |
10 11 |
Total_School_Transport Total cost of transportation ks12aa + ks12ab School_Transport_IHH Cost of transporting children / ks12aa families in the household |
12 |
School_Transport_OHH Cost of transporting children / family ks12ab outside the household |
13 |
Food_Rent_School Boarding fees / rent included ks12bb |
Description: The outcome variables derived from data IFLS 5 b1_ks0 variables. OHH: Outside the Household, IHH: Inside the Household
95
Discussion and feedback